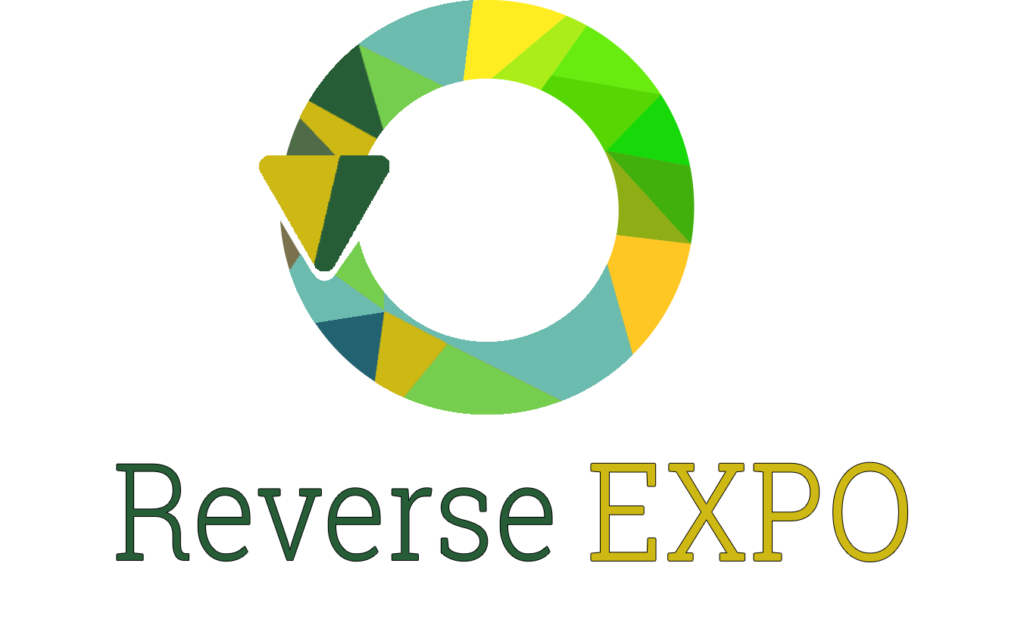
2022
On February 18th, 2022 AI4Society organized Reverse EXPO, a space where more than 60 AI related projects by Undergraduate and Graduate students, Post-doc fellows, and Institutions from the University of Alberta were showcased for the industry and community.
Find in this website the materials shared of each of these future researchers!
Social Sciences and Humanities
- Social Sciences and Humanities
- Addison Primeau
- Christina Li
- Deepak Paramashivan
- Gavriel Myles Froilan
- Grace McLean
- Hero Laird 1
- Hero Laird 2
- Hero Laird 3
- Kaitlyn Konkin
- Kate Bang
- Kaydin Dion Williams
- Leila Zolfalipour
- Marilène Oliver
- Nicolás Arnáez
- Sara Barnard
- Vardan Saini
Social Sciences and Humanities
16 projects related to Law, Visual Arts, Music, and more!
Click on the tabs in the left to access the material of each presenter
AI Generated Affordable Housing
Researcher: Addison Primeau
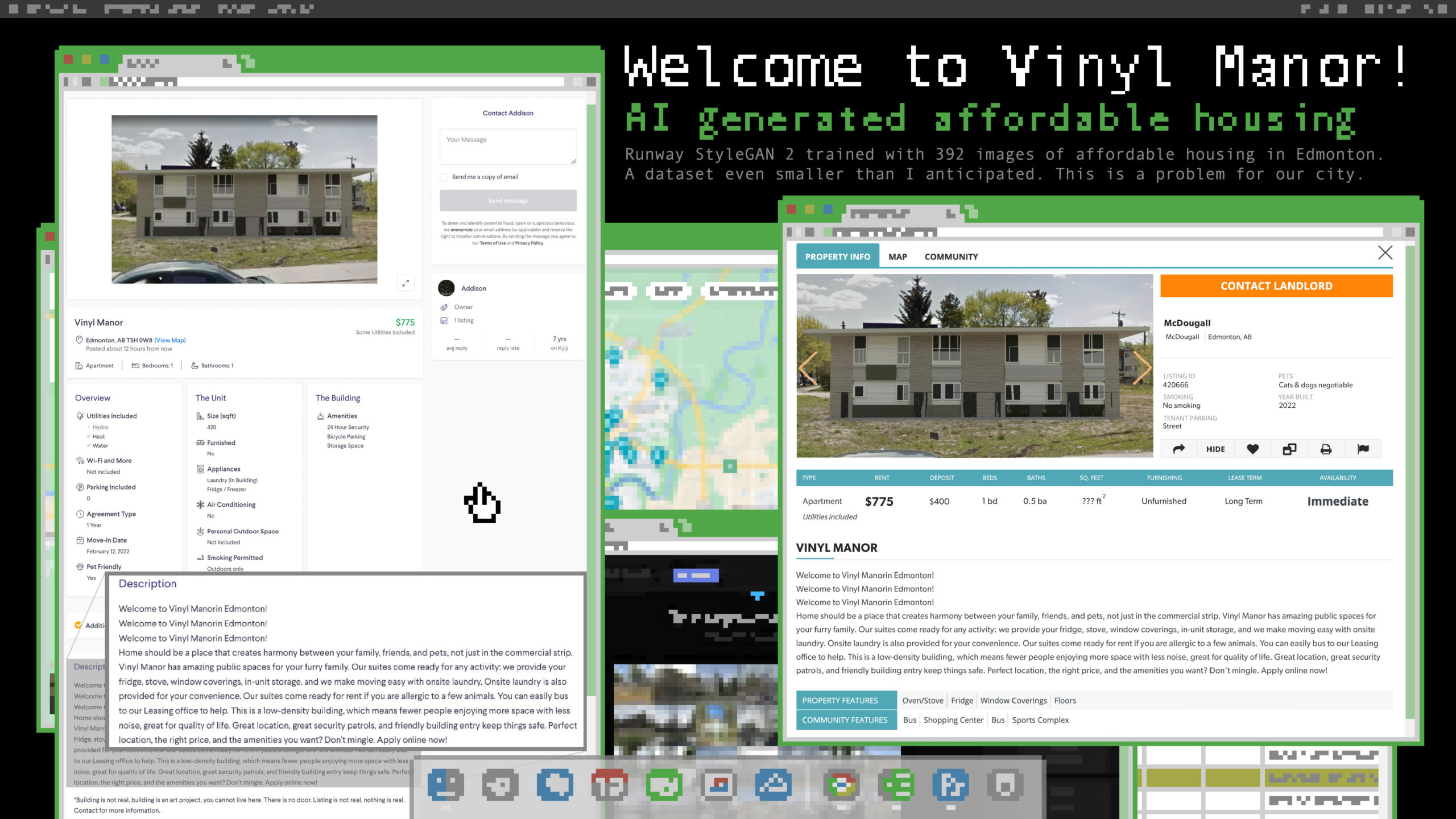
More at: https://www.kijiji.ca/v-apartments-condos/edmonton/vinyl-manor/1605274810
A Demonstration of Mechanic Maker: An AI for Mechanics Co-Creation
Researcher: Vardan Saini | Supervisor: Matthew Guzdial
Developing games is challenging as it requires expertise in programming skills and game design. This process is costly and consumes a great deal of time. In this paper we describe a tool we developed to help decrease this burden: Mechanic Maker. Mechanic Maker is designed to allow users to make 2D games without programming. A user interacts with a machine learning AI agent, which learns game mechanics by demonstration.
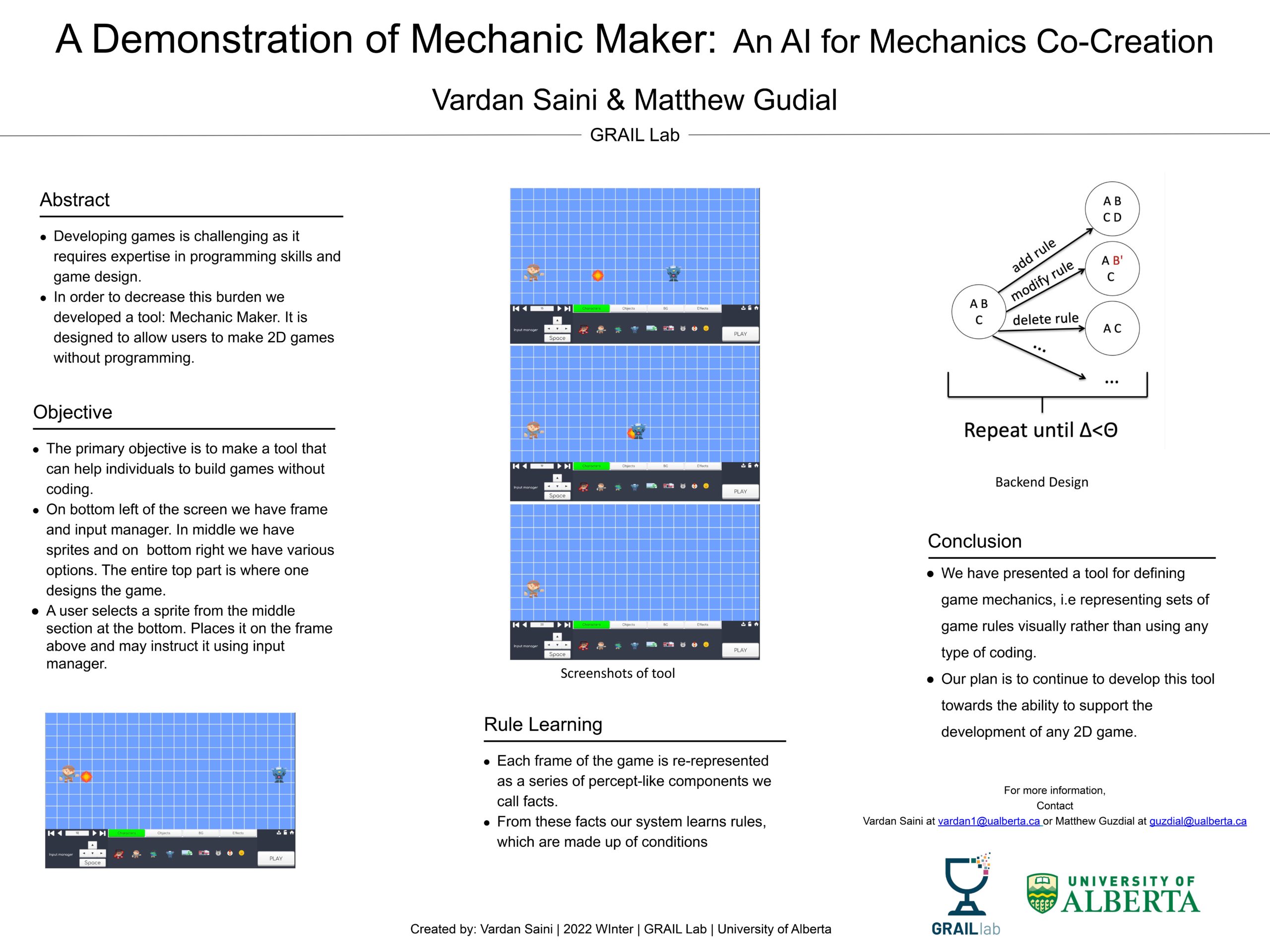
A Demonstration of Mechanic Maker: An AI for Mechanics Co-Creation
Researcher: Christina Li
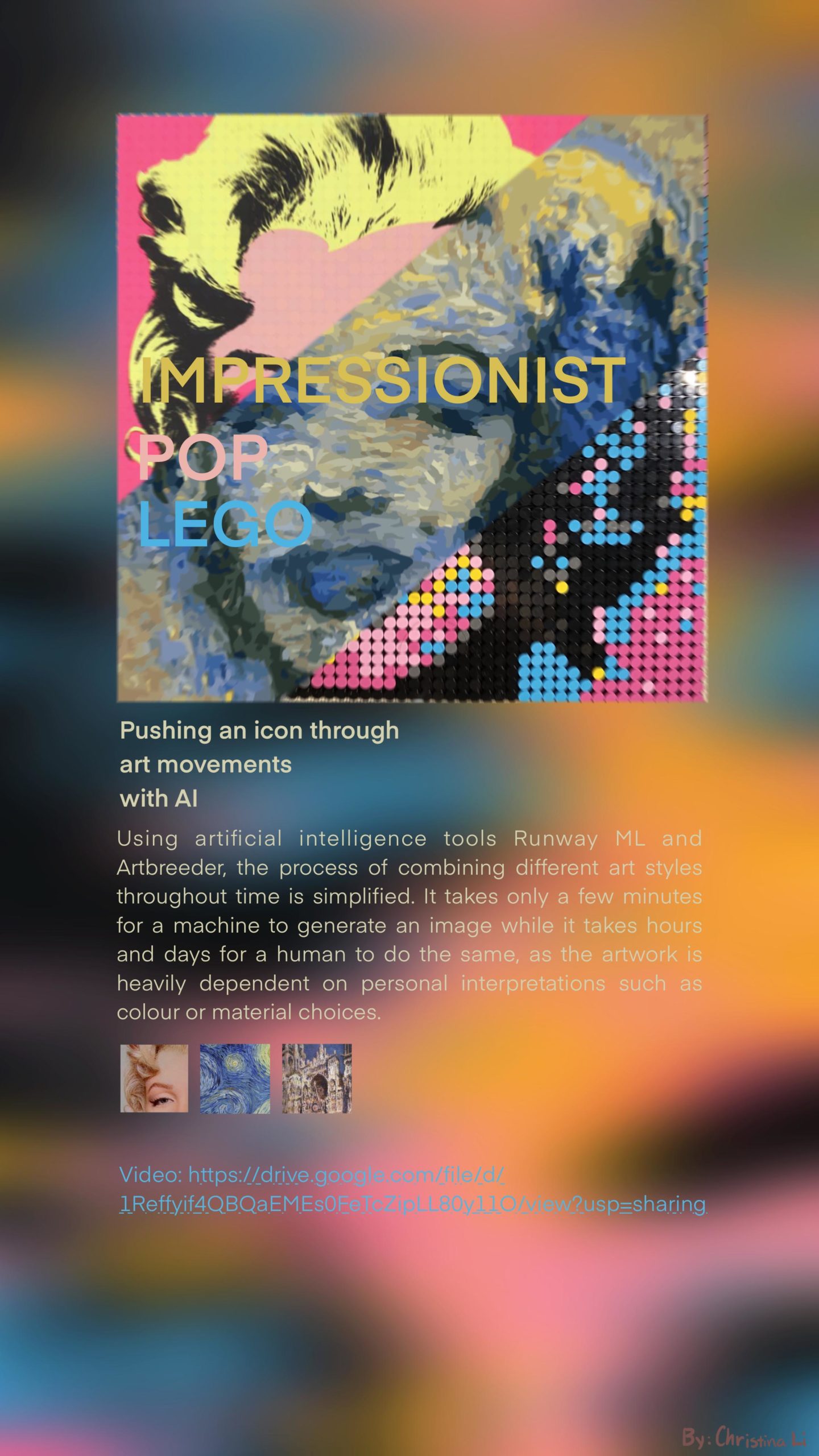
AI and Social Media Identity
Researcher: Grace McLean
Learn more at:
- https://www.instagram.com/kat.aleyn/
- https://xd.adobe.com/view/6895b77e-2275-4133-a08e-6dc5a6ea6909-3dc6/
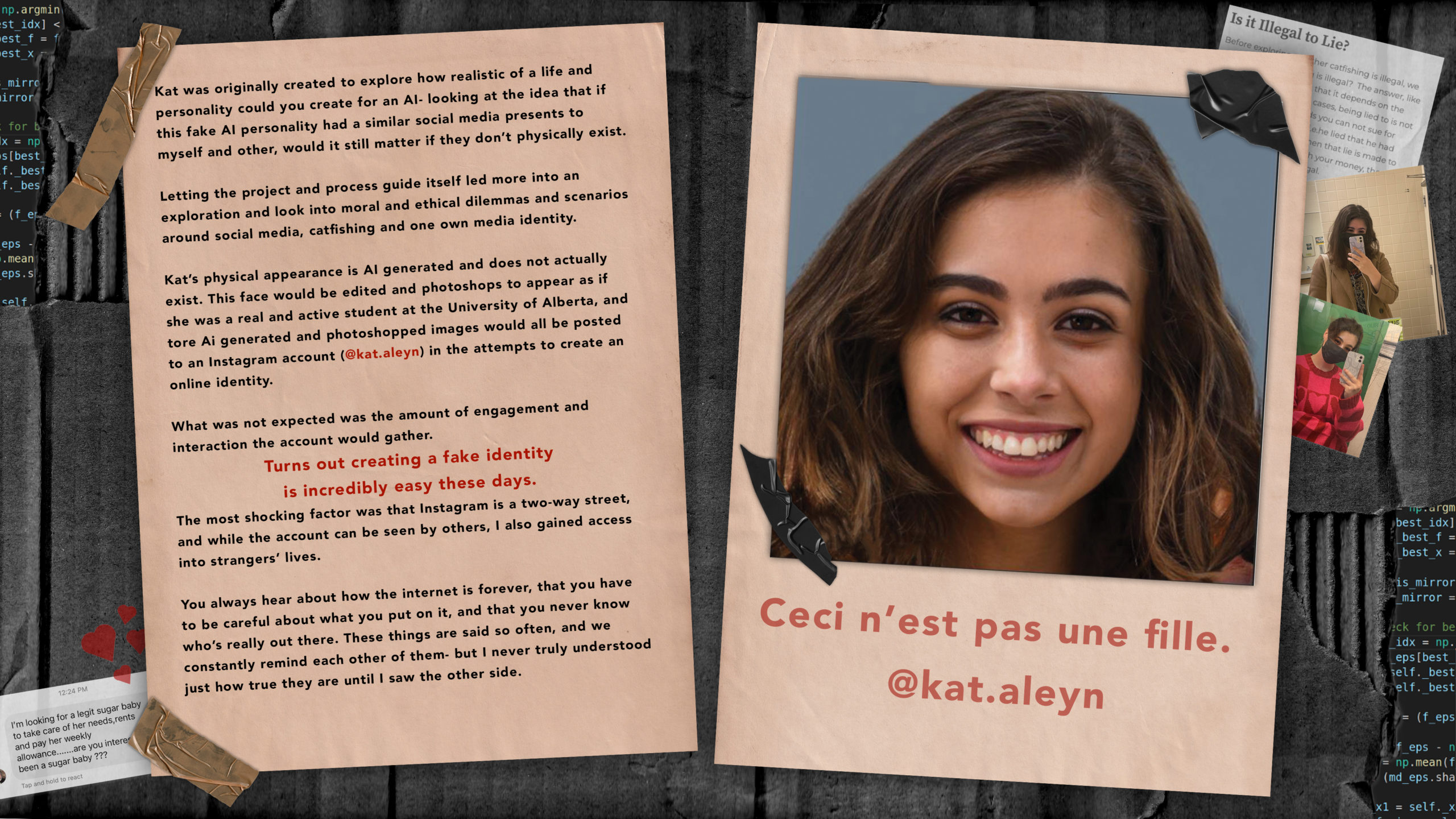
Legal Innovation Zone feasibility study
Researcher: Hero Laird
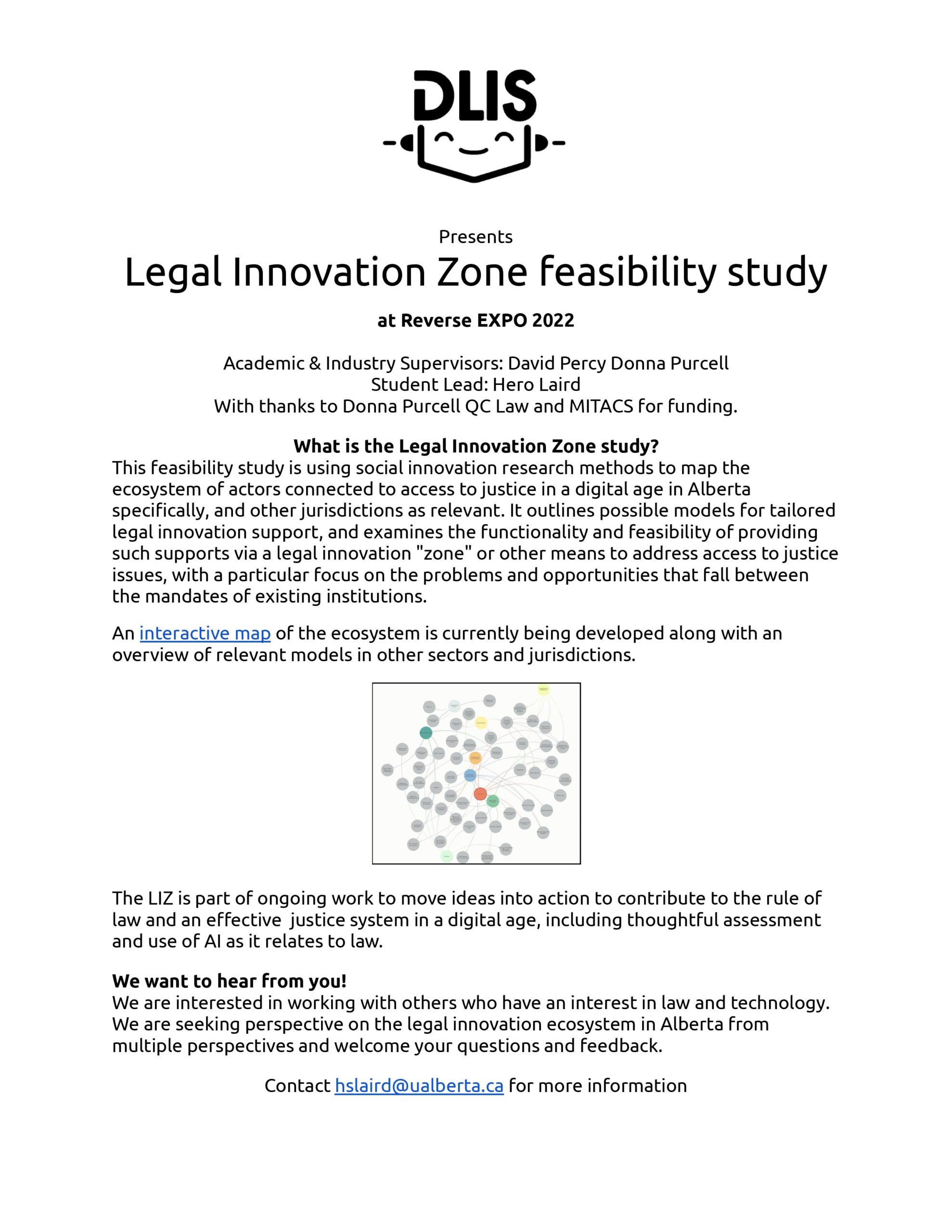
Interactive Map: https://kumu.io/hslaird/lizproject#legal-systems-reform-groups-in-alberta
Beacon
Researchers Heor Laird, Ohi Ahimie, Isaias Briones
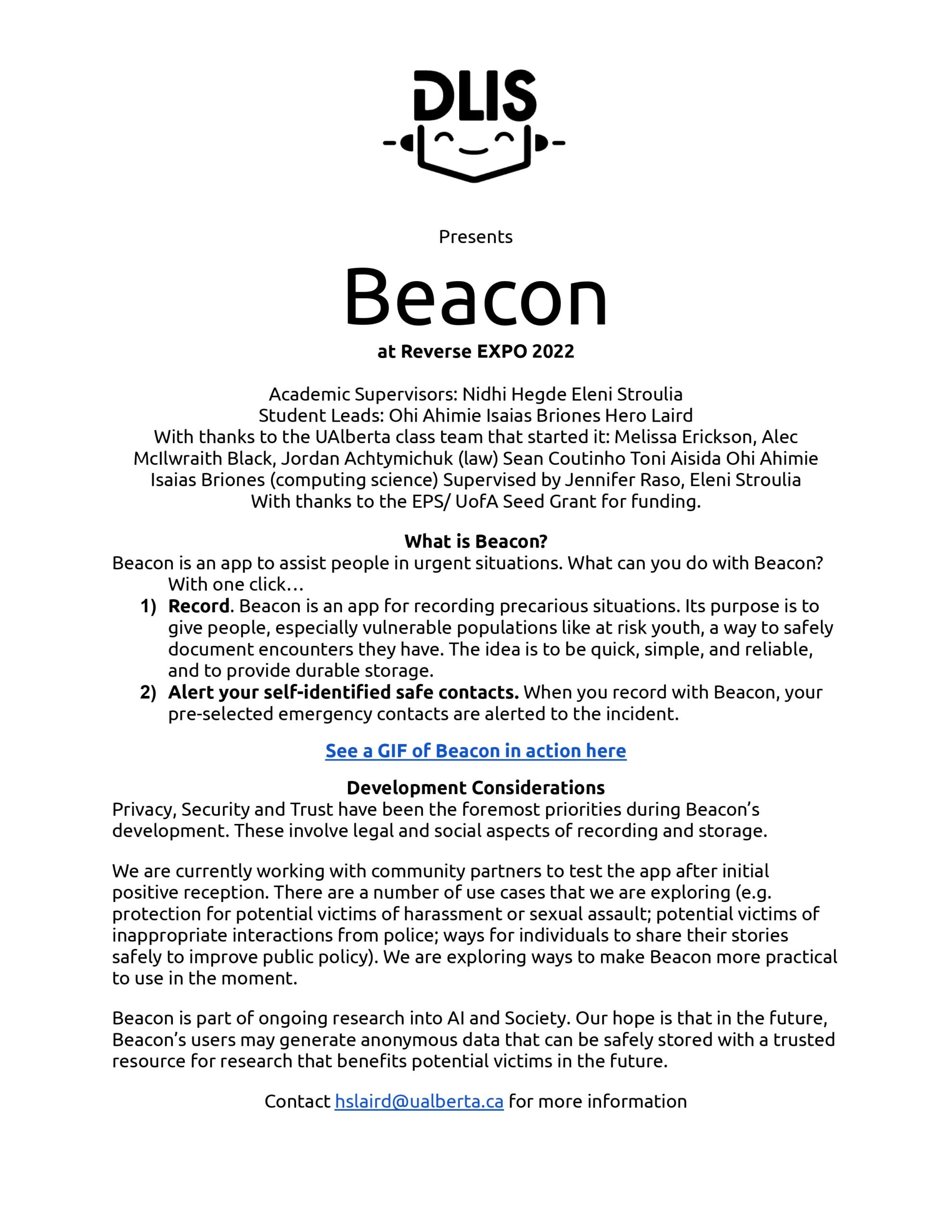
See a GIF of Beacon in action here: https://media.discordapp.net/attachments/850466274861383698/940070055981494312/BeaconTutorialDemo.gif
GEMUTLICH; Playing Family Games with an AI
Researcher: Kaitlyn Konkin
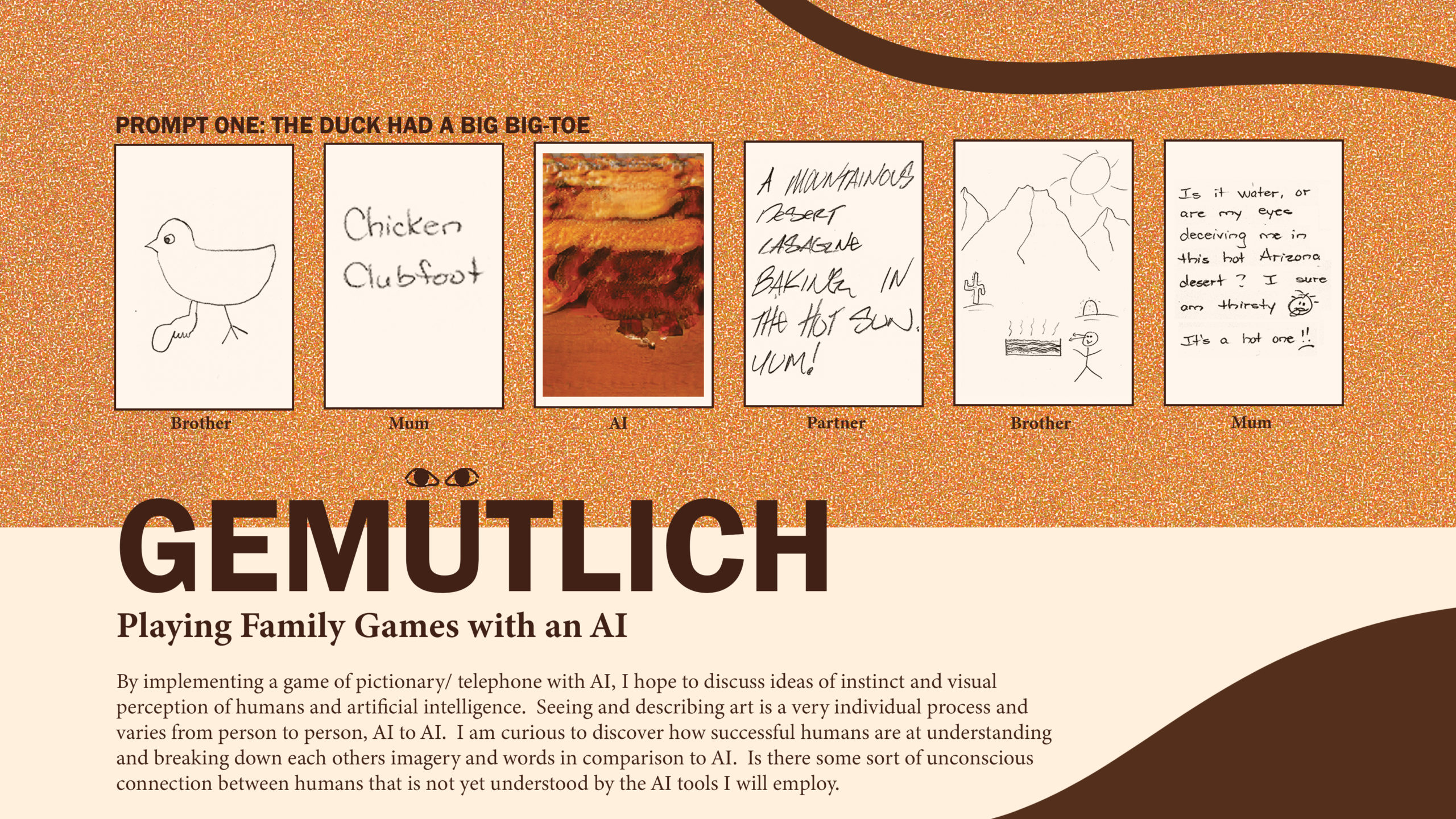
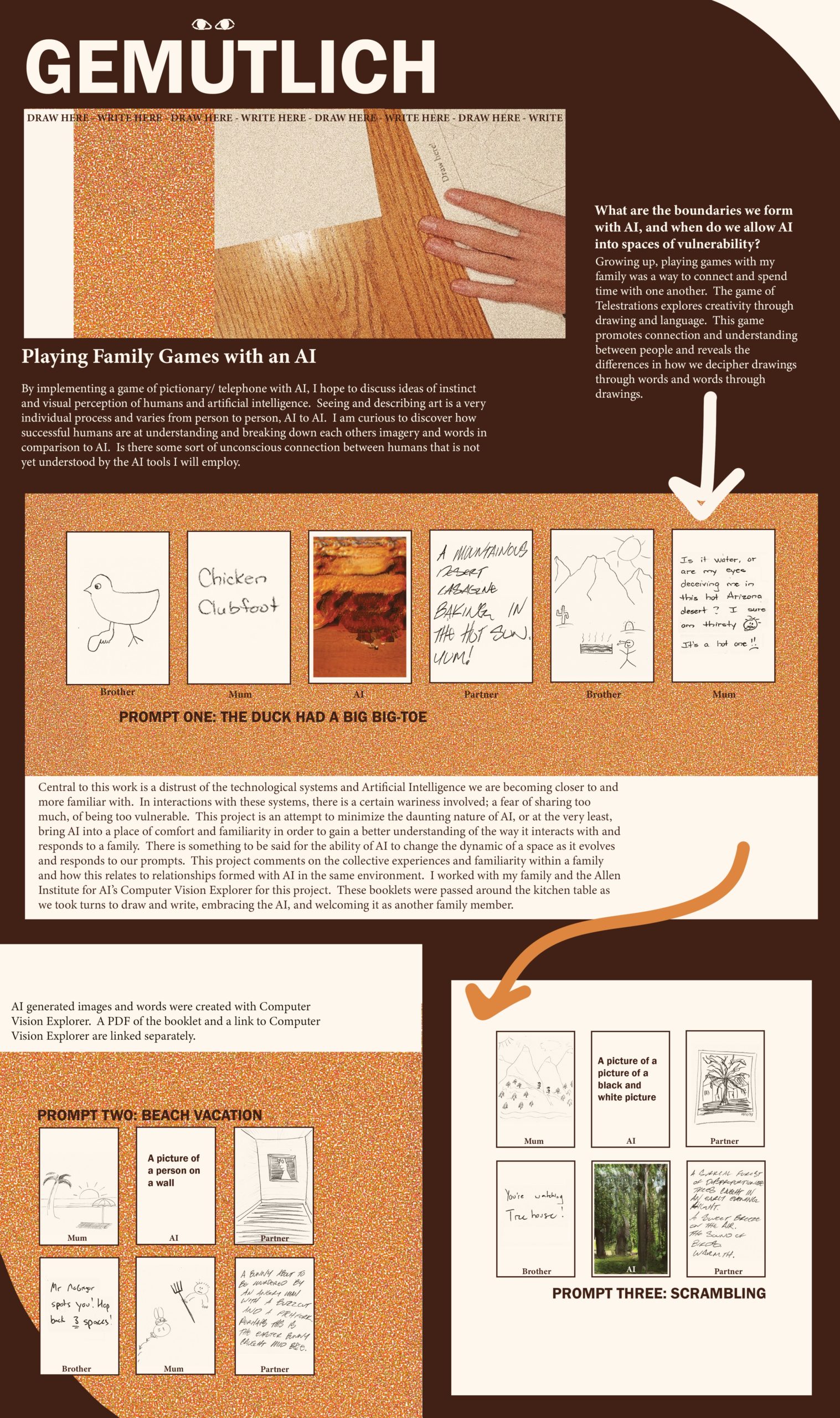
Links:
Computer Vision
To generate images from text prompts / captions: https://vision-explorer.allenai.org/text_to_image_generation
To caption an uploaded image: https://vision-explorer.allenai.org/image_captioning
Points of Absolute Reality
Researcher: Kaydin Dion Williams
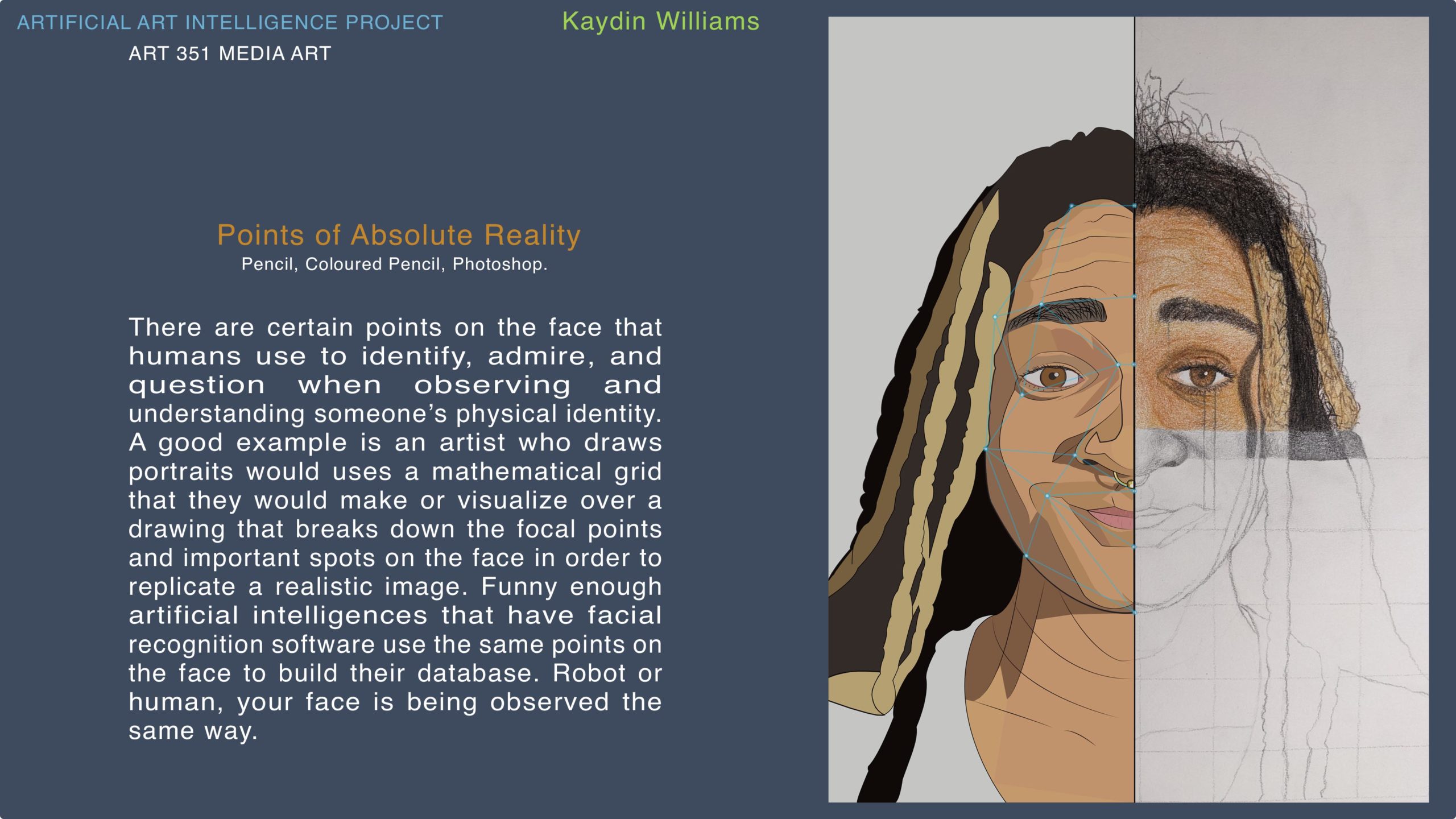
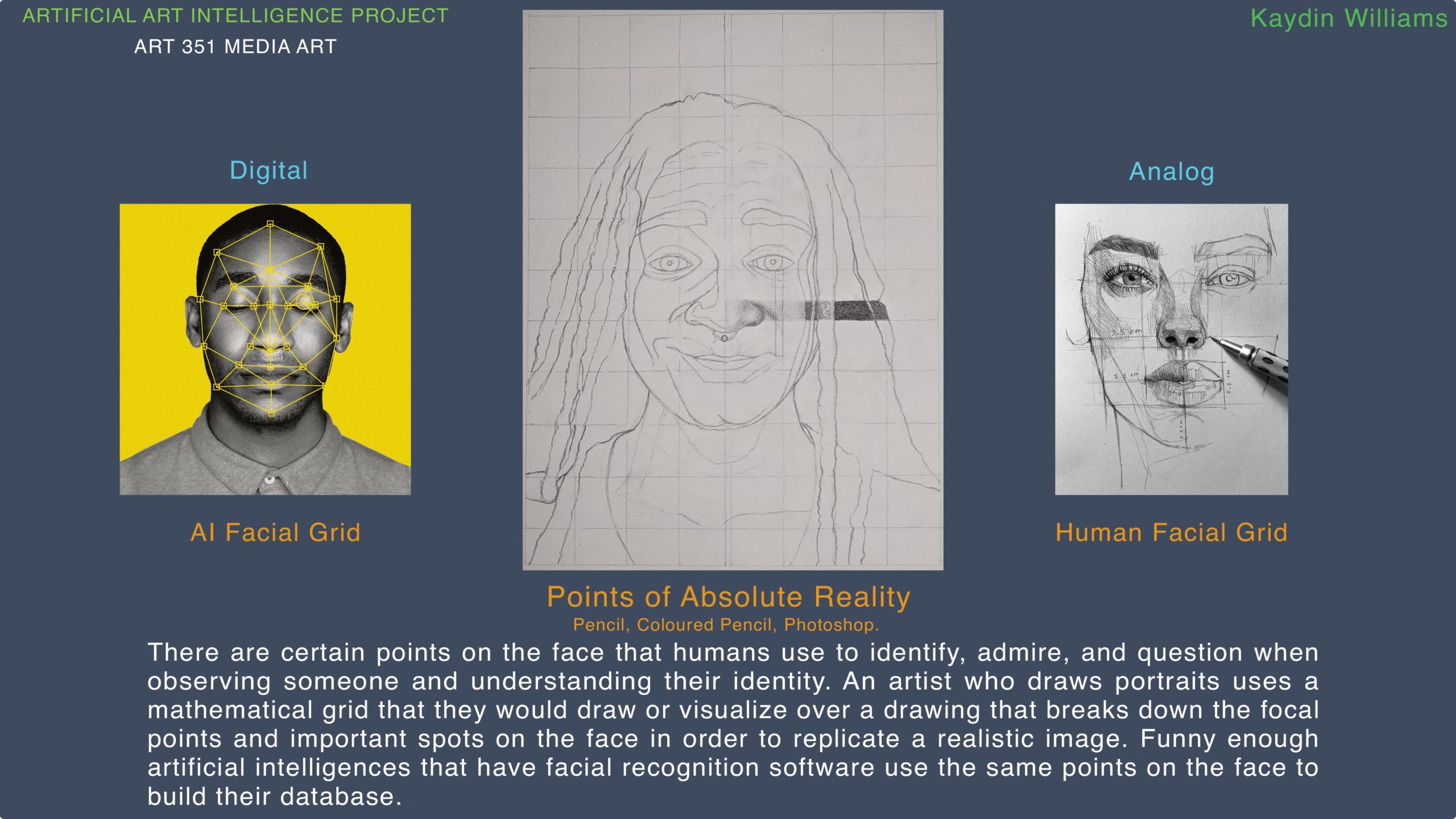
Artificial Art Intelligence Project: Art 351 Media Art
Researcher: Marilène Oliver
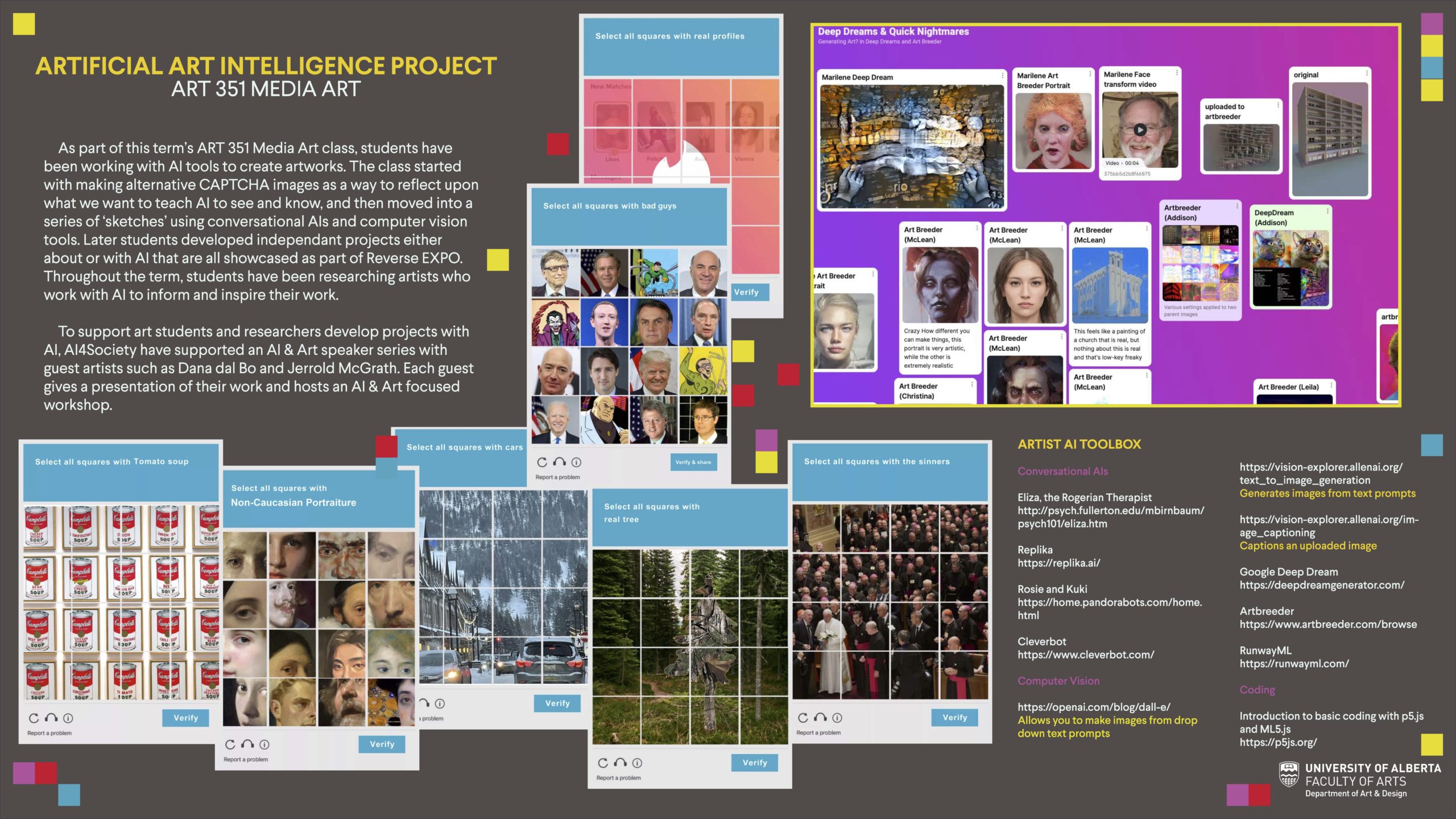
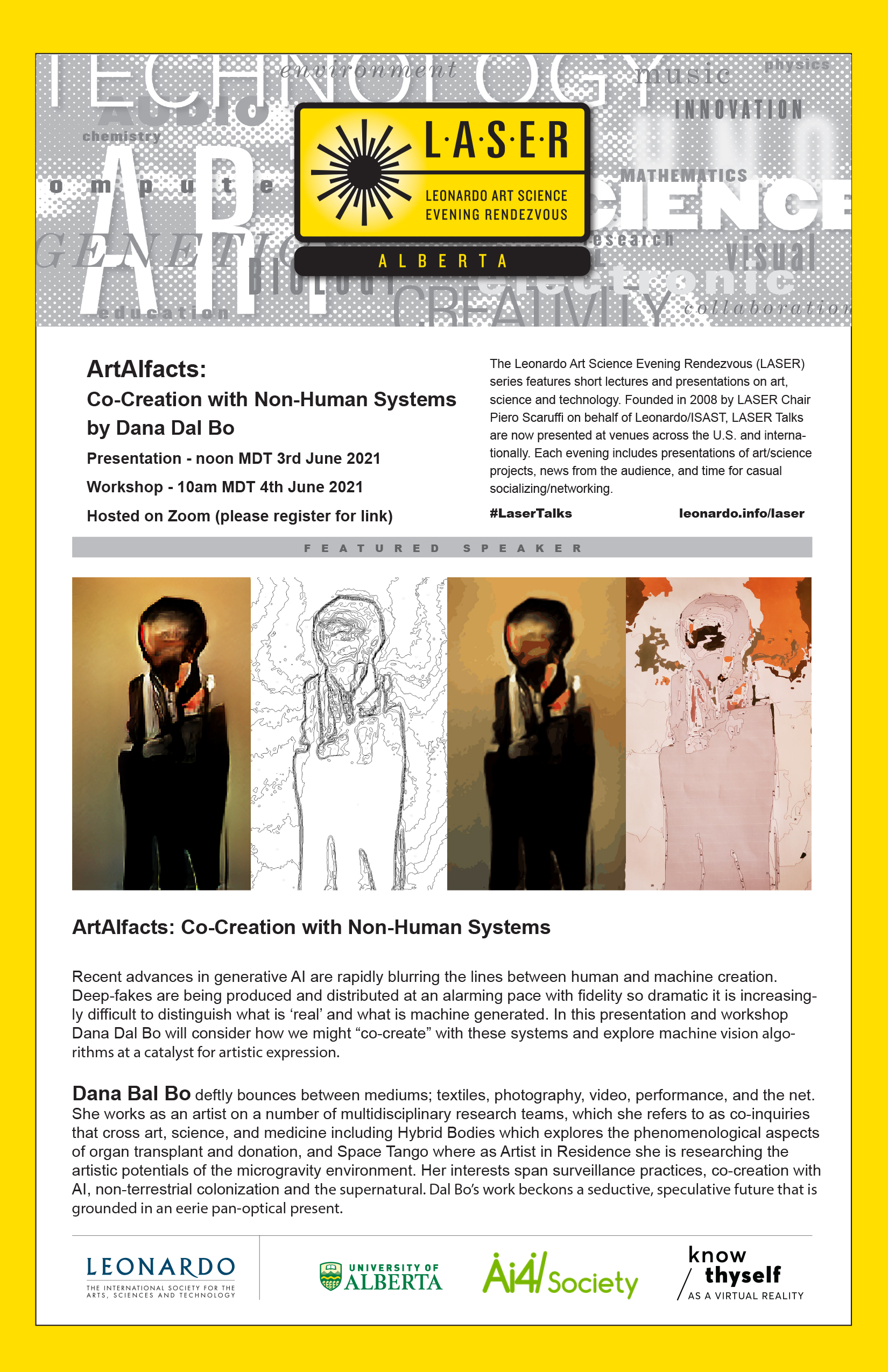
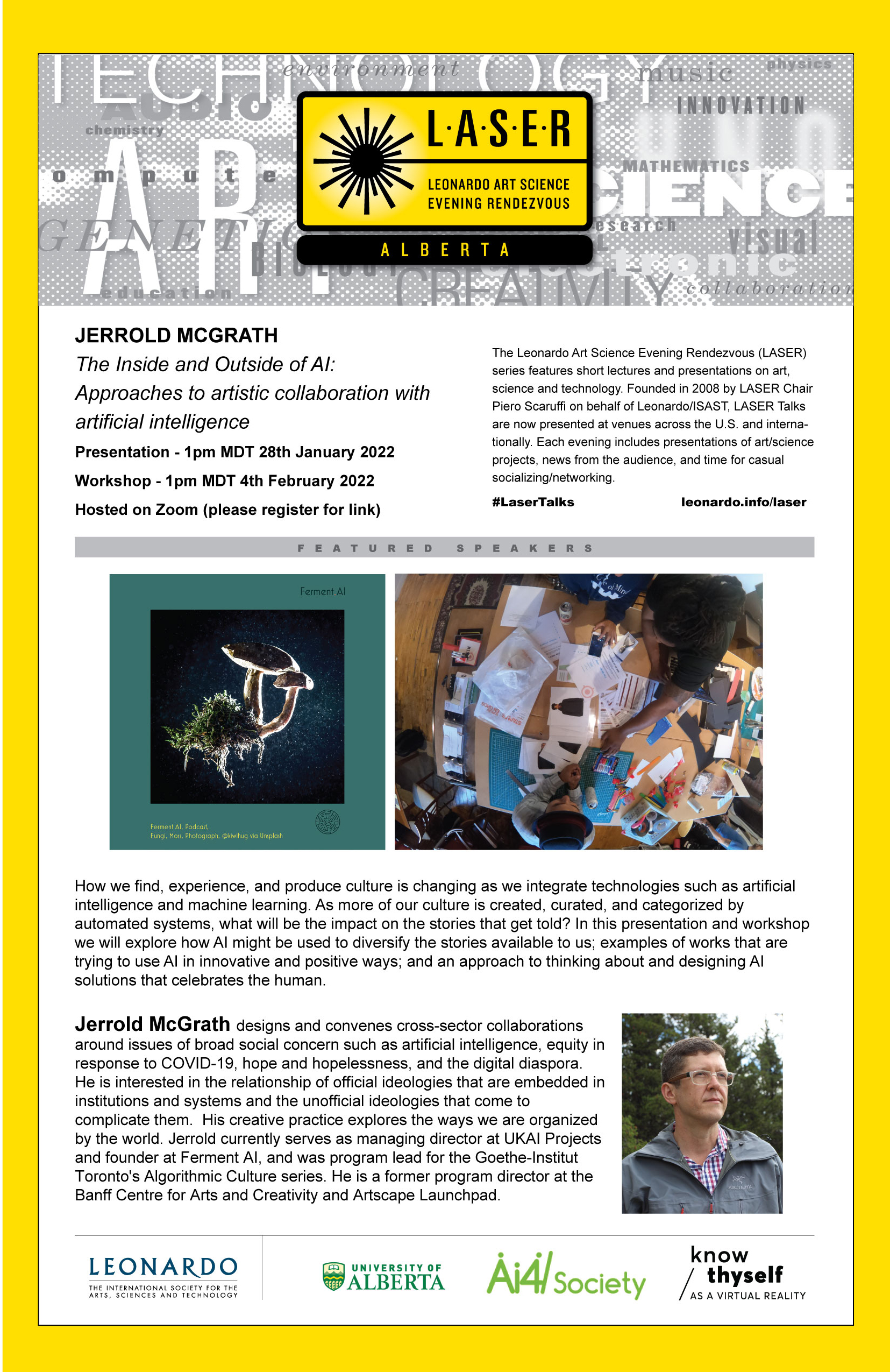
The video for Jerrold McGrath lecture will be available soon
More at: https://www.knowthyself.ualberta.ca/
Ethicsbot: Considering the Ethics of Artificial Intelligence
Researchers: Sara Barnard, Emad Mousavi, Paolo Verdini, Jingwei Wang, Ali Azarpanah
Supervisor: Geoffrey Rockwell
Within the field of Digital Humanities, artificial intelligence (AI) is commonly applied to a variety of tasks and situations such as sentiment analysis, data visualization, and language processing. However, the widespread usage of AI does not come without ethical concerns, and ongoing discussions on the ethical ramifications of AI usage in the Digital Humanities have developed almost simultaneously with its use. Conceptually descending from other interactive and thematic-specific chatbots such as Faulknerbot and Eververse, the Ethicsbot, an artificially intelligent chatbot, was constructed to explore, provoke, and reflect upon the relationship between artificial intelligence and its ethical use, especially in relation to datafication and bias. The poster will provide an overview of the Ethicsbot and how we are using it to provoke reflection on the ethics of artificial intelligence. This will include an overview of its construction using GPT-2, our methodology in prompting responses from the chatbot, and some of our preliminary results.
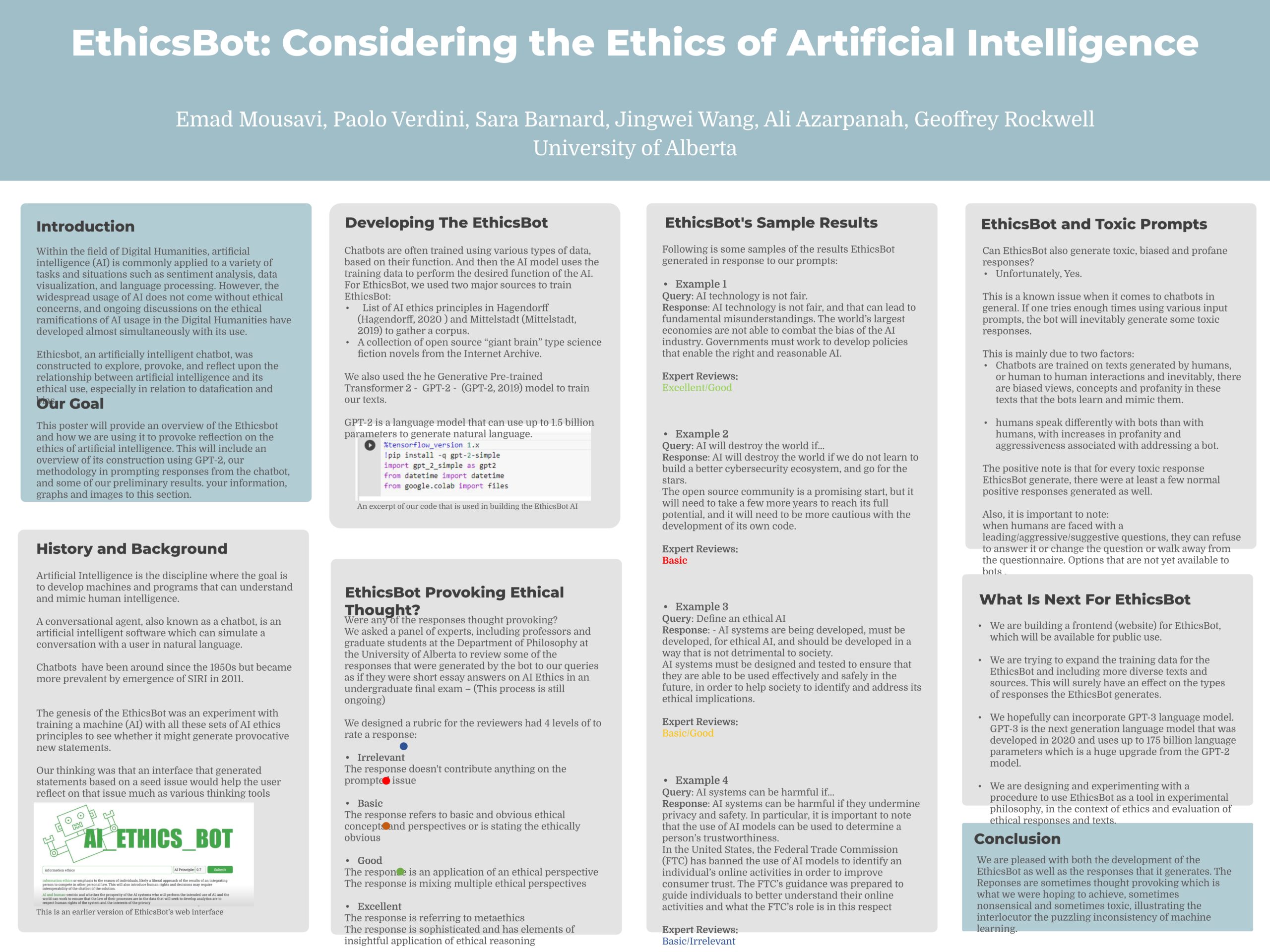
A Demonstration of Mechanic Maker: An AI for Mechanics Co-Creation
Researcher: Vardan Saini | Supervisor: Matthew Guzdial
Developing games is challenging as it requires expertise in programming skills and game design. This process is costly and consumes a great deal of time. In this paper we describe a tool we developed to help decrease this burden: Mechanic Maker. Mechanic Maker is designed to allow users to make 2D games without programming. A user interacts with a machine learning AI agent, which learns game mechanics by demonstration.
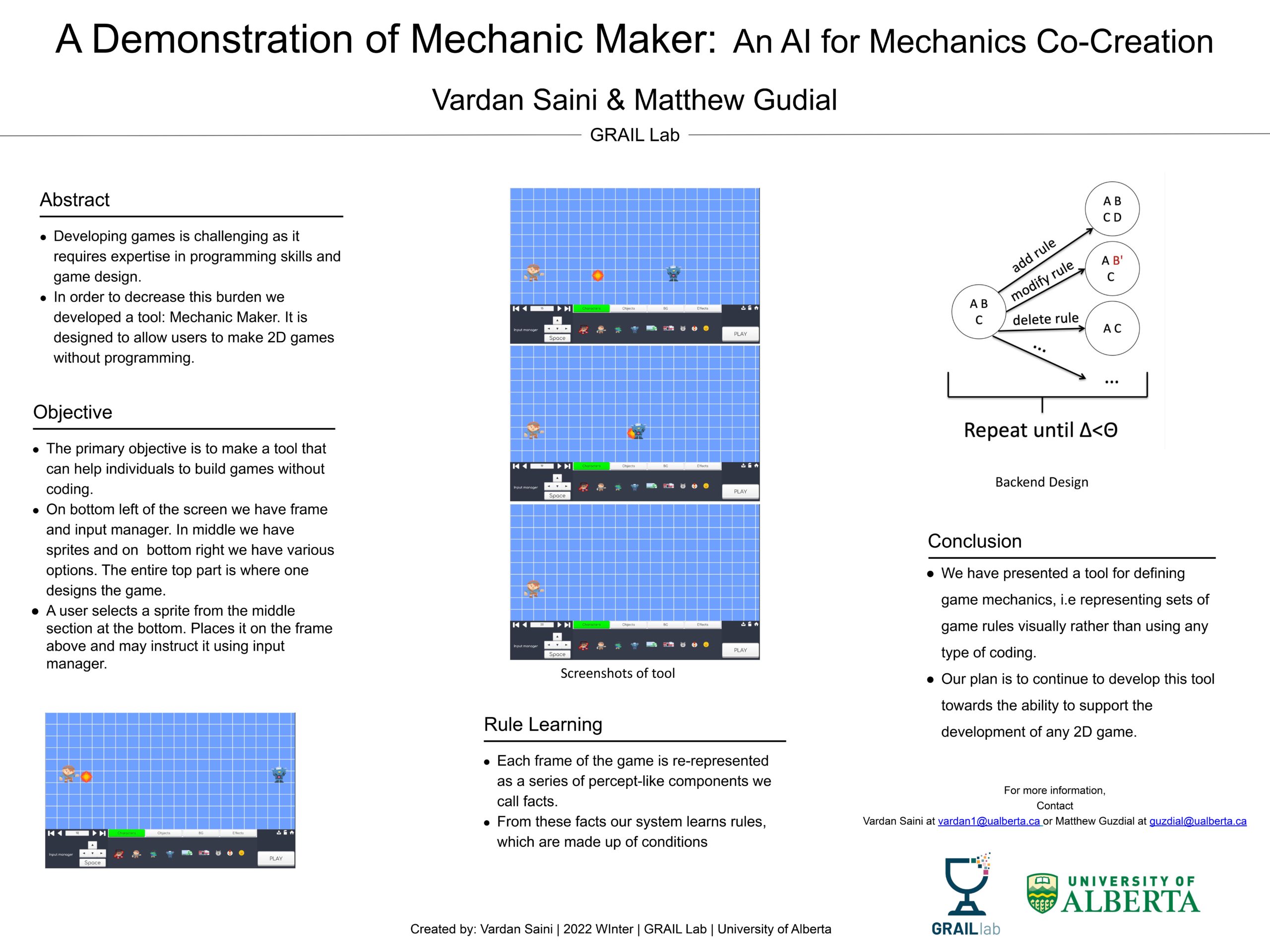
AI and Social Media Identity
Project description:
Supervisor:
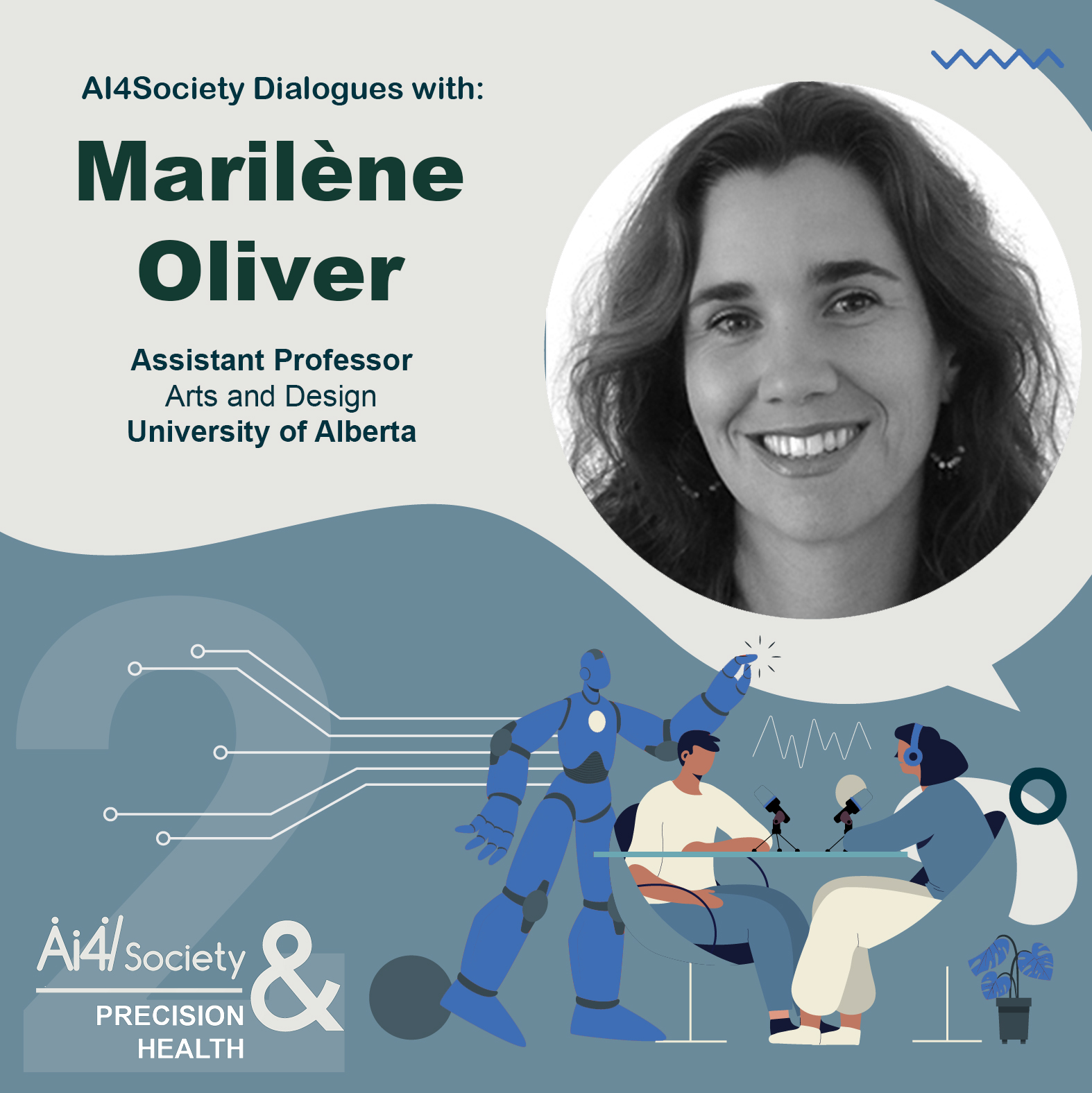
Description:
Supervisor:
Description:
Developing games is challenging as it requires expertise in programming skills and game design. This process is costly and consumes a great deal of time. In this paper we describe a tool we developed to help decrease this burden: Mechanic Maker. Mechanic Maker is designed to allow users to make 2D games without programming. A user interacts with a machine learning AI agent, which learns game mechanics by demonstration.
sdafsdf
Researcher: Vardan Saini
Supervisor: Matthew Guzdial
Developing games is challenging as it requires expertise in programming skills and game design. This process is costly and consumes a great deal of time. In this paper we describe a tool we developed to help decrease this burden: Mechanic Maker. Mechanic Maker is designed to allow users to make 2D games without programming. A user interacts with a machine learning AI agent, which learns game mechanics by demonstration.
Description:
Learn more at https://www.knowthyself.ualberta.ca/
Education
- Education
- Bin Tan 1
- Bin Tan 2
- Bukola Oladunni Salami
- Cathy Adams
- Echo Zexuan Pan
- Hao-Yue Jin
- Seyma Yildirim-Erbasli
- Sheldon Roberts
- Tarid Wongvorachan
Education
9 projects related to Education, Youth, Pathways, and more!
Click on the tabs in the left to access the material of each presenter
Machine Learning Methods in Computational Thinking Assessments: A Scoping Review
Researcher: Bin Tan, Hao-yue Jin | Supervisor: Maria Cutumisu
Computational thinking is a fundamental skill set for solving problems in the contemporary computational world. To facilitate teaching and learning computational thinking, researchers have developed and implemented many computational thinking assessments that yielded a wealth of complex assessment data. Many existing studies have demonstrated that machine learning approaches are promising in replacing human graders when analyzing assessment data. The present study employed a scoping review methodology to examine the extent, range, and nature of relevant research activities, summarize and disseminate research findings, and identify gaps in the existing body of literature. This study identified 22 peer-reviewed publications that applied machine learning approaches to assess computational thinking competencies. It analyzed the existing research activities from four perspectives: (1) the data used for training and validating machine learning algorithms; (2) the educational context where the assessments were implemented; (3) the specific machine learning approaches employed; and (4) the aspects of computational thinking assessed. This work makes the following contributions: (1) it reveals that using machine learning approaches to assess computational thinking competencies tends to be effective, reliable, time-saving, and superior to human graders; (2) it identifies several research gaps in the related literature; and (3) it provides directions for future research. We hope this review brings attention to the ML-based computational thinking assessments and potentially provides researchers with a guide for future research and educational practices of computational thinking assessments
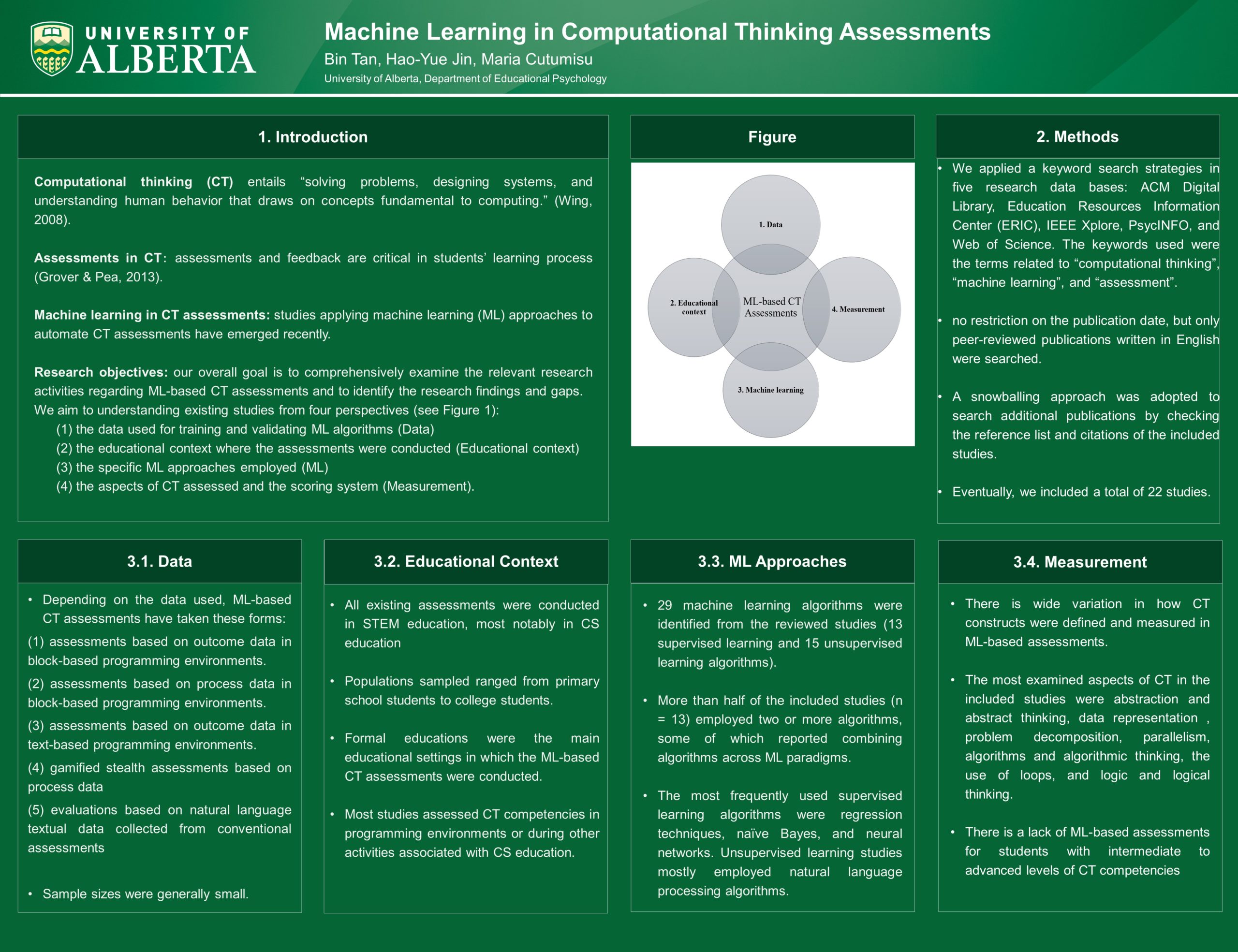
A Machine Learning Approach to Predicting Students’ Self-Efficacy
Researcher: Bin Tan | Supervisor: Maria Cutumisu
Self-efficacy is a critical psychological trait that has a significant impact on students’ learning and overall well-being. Thus, it is important for educators and researchers to trace students’ self-efficacy and identify low self-efficacious individuals early in the research process. This research uses the OECD Programme for International Student Assessment (PISA) 2018 dataset to train two tree-based ensemble learning models (random forest and XGBoost) to predict self-efficacy from students’ responses to survey questions. The findings revealed that the two trained machine learning models were capable of accurately predicting students’ self-efficacy with small error sizes. Students’ psychological characteristics and attitudes appeared to be the most important predictors when ranking the relative importance of predictors in the models. This study provided an example of how machine learning approaches can be used to predict students’ self-efficacy.
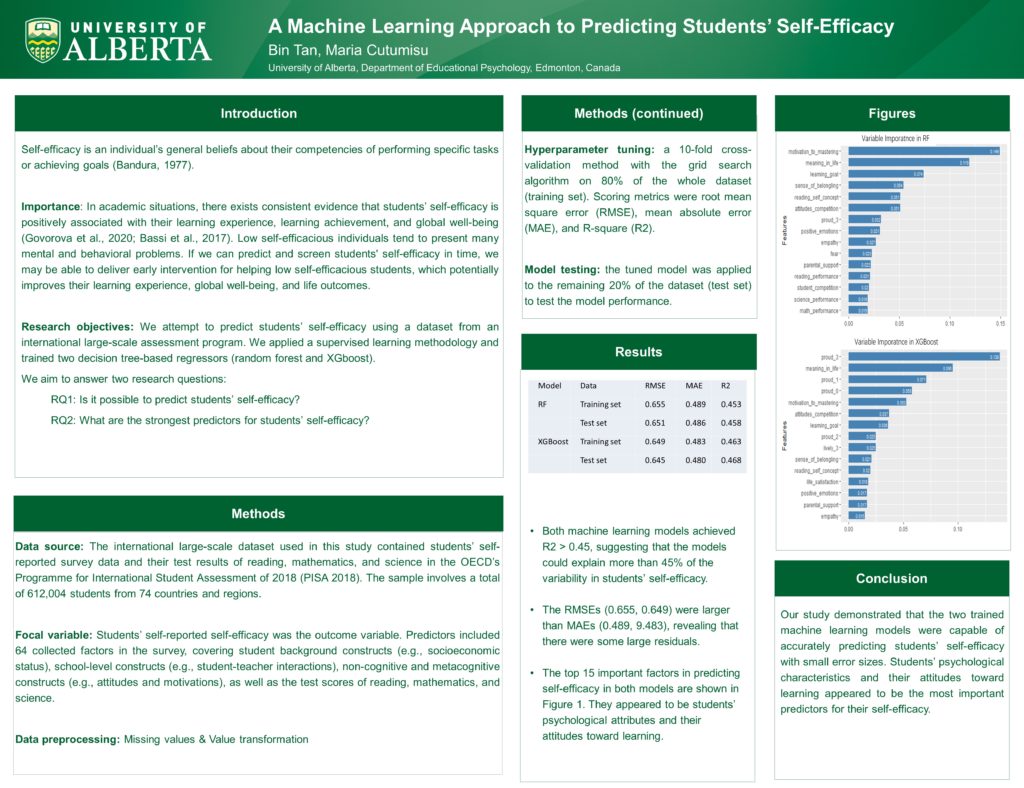
The Black Youth Mentorship and Leadership Program
Researcher: Bukola Oladunni Salami, Jared Wesley, Myra Kandemiri, Omolara Sanni
Black youths experience social, economic, and health inequities in Canada, and are less likely to attain a post-secondary qualification. Strong evidence indicates mentorship is effective across behavioral, social, emotional, and academic domains of youth development. The Black Youth Leadership and Mentorship Program (BYMLP) uses a participatory approach aimed at improving community belonging and leadership skills, as well as fostering a positive cultural identity for Black youths.
The program began in Fall 2020, when it was offered to 36 youths. It ran again in the Summer and Fall of 2021, when it was offered to 37 youths. The youths are selected via a competitive process. Evaluation feedback suggests that the program has a positive impact on overall cultural identity for Black youth. It creates a sense of community and fosters a positive sense of identity among mentees, Black professors and graduate students involved.
Mentors, and program staff serve as important references supporting program youths’ future work and academic endeavors. Further, youths become part of a network of alumni to which research assistantships, community-based projects, and other employment opportunities are shared by program staff, during and after the program, with positive economic outcomes for those taking up the opportunities.
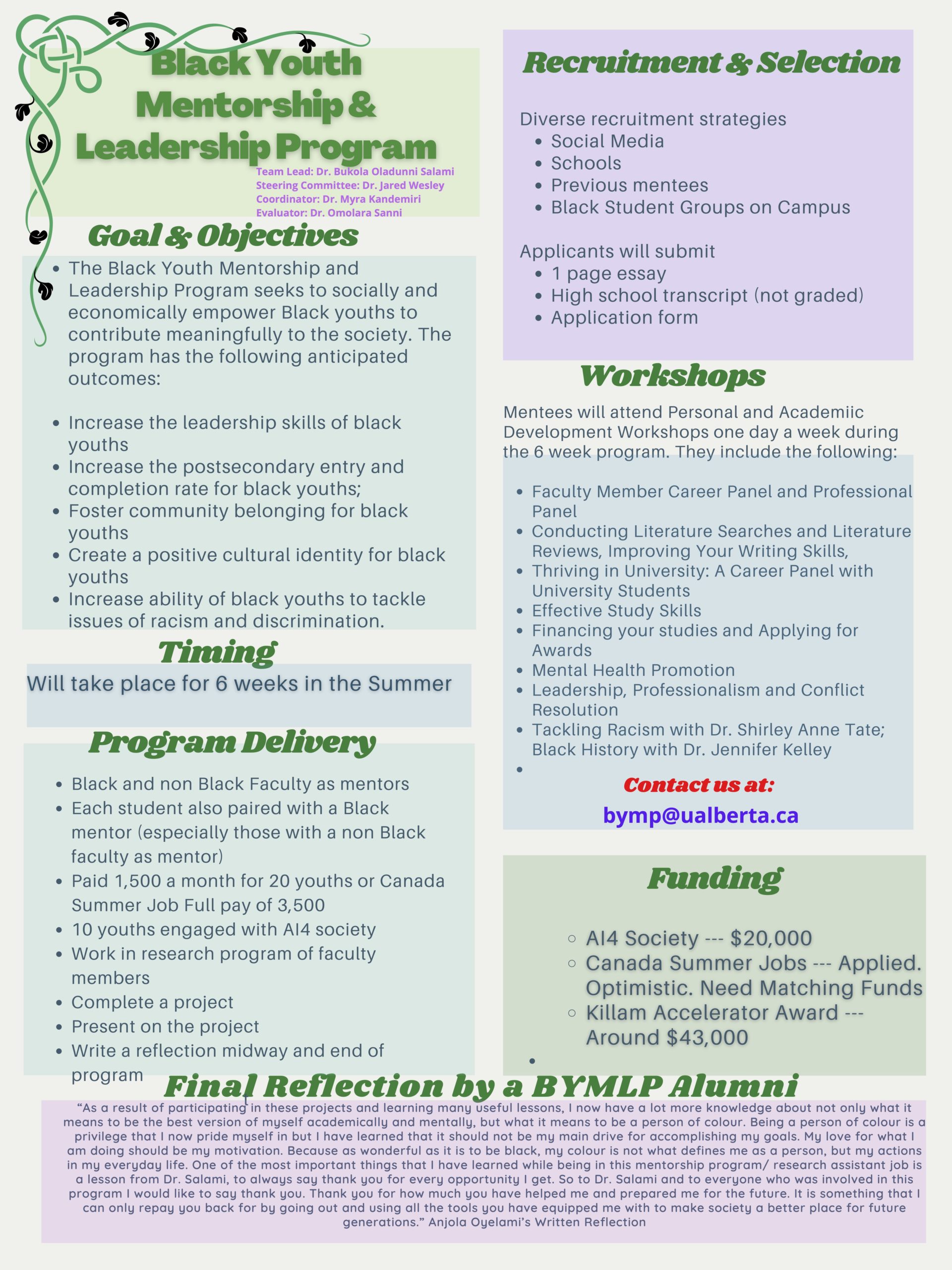
Artificial Intelligence Ethical Guidelines in K-12 Education: A Global Perspective
Researchers: Cathy Adams, Patti Pente, Gillian Lemermeyer
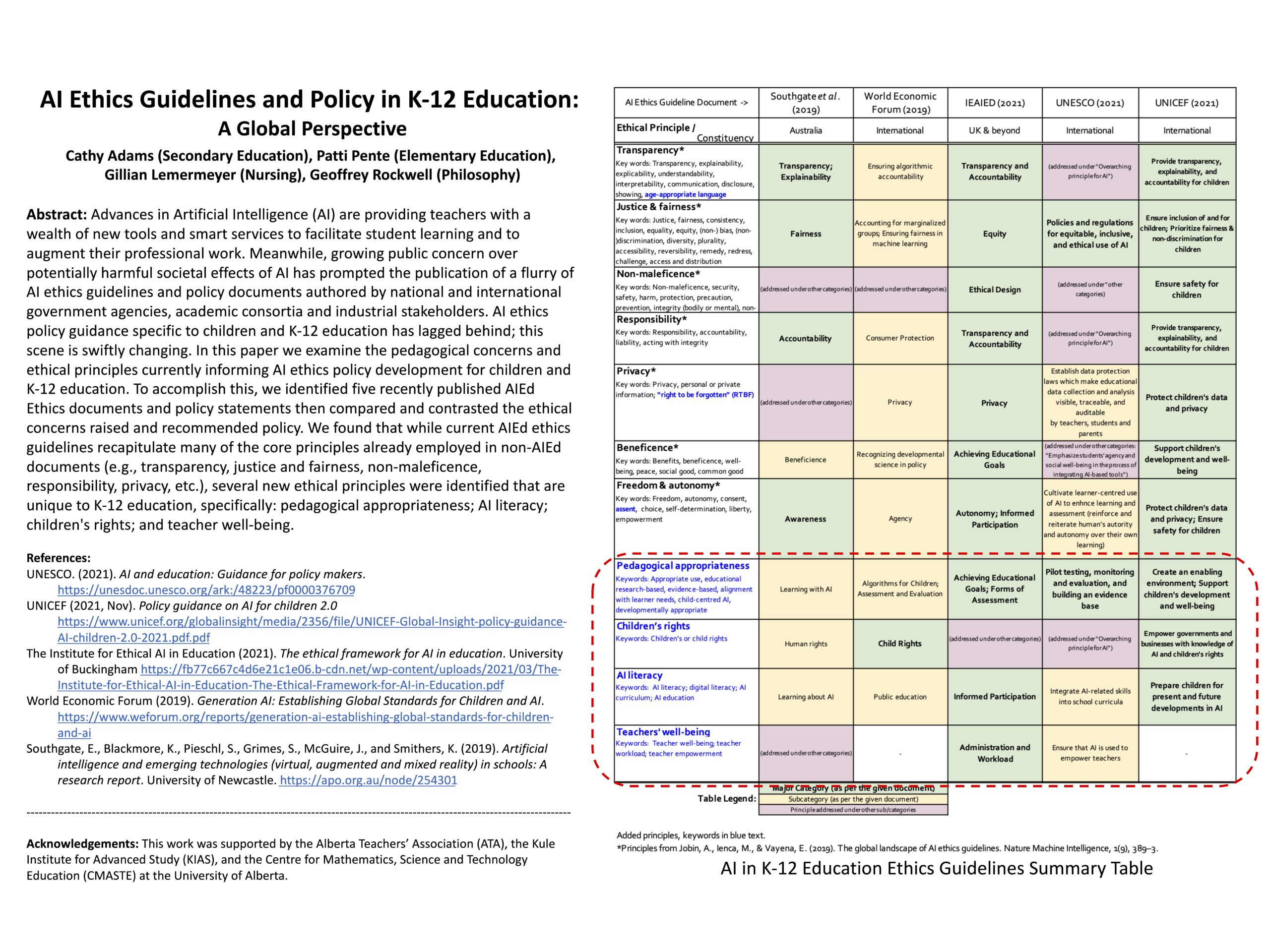
Publication:
Adams C., Pente P., Lemermeyer G., & Rockwell G. (2021). Artificial Intelligence ethics guidelines for K-12 education: A review of the Global Landscape. Lecture Notes in Computer Science, 12749, 24-28. Springer, Cham. https://doi.org/10.1007/978-3-030-78270-2_4
References:
- UNESCO. (2021). AI and education: Guidance for policy makers. https://unesdoc.unesco.org/ark:/48223/pf0000376709
- UNICEF (2021, Nov). Policy guidance on AI for children 2.0 https://www.unicef.org/globalinsight/media/2356/file/UNICEF-Global-Insight-policy-guidance-AI-children-2.0-2021.pdf.pdf
- The Institute for Ethical AI in Education (2021). The ethical framework for AI in education. University of Buckingham https://fb77c667c4d6e21c1e06.b-cdn.net/wp-content/uploads/2021/03/The-Institute-for-Ethical-AI-in-Education-The-Ethical-Framework-for-AI-in-Education.pdf
- World Economic Forum (2019). Generation AI: Establishing Global Standards for Children and AI. https://www.weforum.org/reports/generation-ai-establishing-global-standards-for-children-and-ai
- Southgate, E., Blackmore, K., Pieschl, S., Grimes, S., McGuire, J., and Smithers, K. (2019). Artificial intelligence and emerging technologies (virtual, augmented and mixed reality) in schools: A research report. University of Newcastle. https://apo.org.au/node/254301
Predicting Secondary Students’ Life Satisfaction with Machine Learning Approaches: Does Culture Matter?
Researcher: Echo Zexuan Pan | Supervisor: Maria Cutumisu
Life satisfaction is a key component of subjective well-being due to its impact on lifelong health and academic achievement. Although previous studies have identified several variables associated with life satisfaction, little is known about their synergistic effect and relative importance. The current study examines the synergistic effect of material resources, social relations, and psychological dispositions in predicting the life satisfaction of students in secondary school. Additionally, the cultural dimensions of individualism versus collectivism are considered. Two machine learning (ML) models were developed based on the UK (individualistic) data and the Japanese (collectivistic) data in PISA 2018. Findings show that (1) the random forest model outperformed the k-nearest neighbors (KNN) model in terms of prediction accuracy in both countries; (2) both the random forest model and the KNN model yielded better accuracy in the UK data than in the Japanese data; (3) the variables related to psychological dispositions played the most important role in predicting students’ life satisfaction in both individualistic and collectivistic cultures. Theoretically, the study implies the potential influence of culture. Practically, it serves as a reference for improving students’ life satisfaction across different cultural contexts.
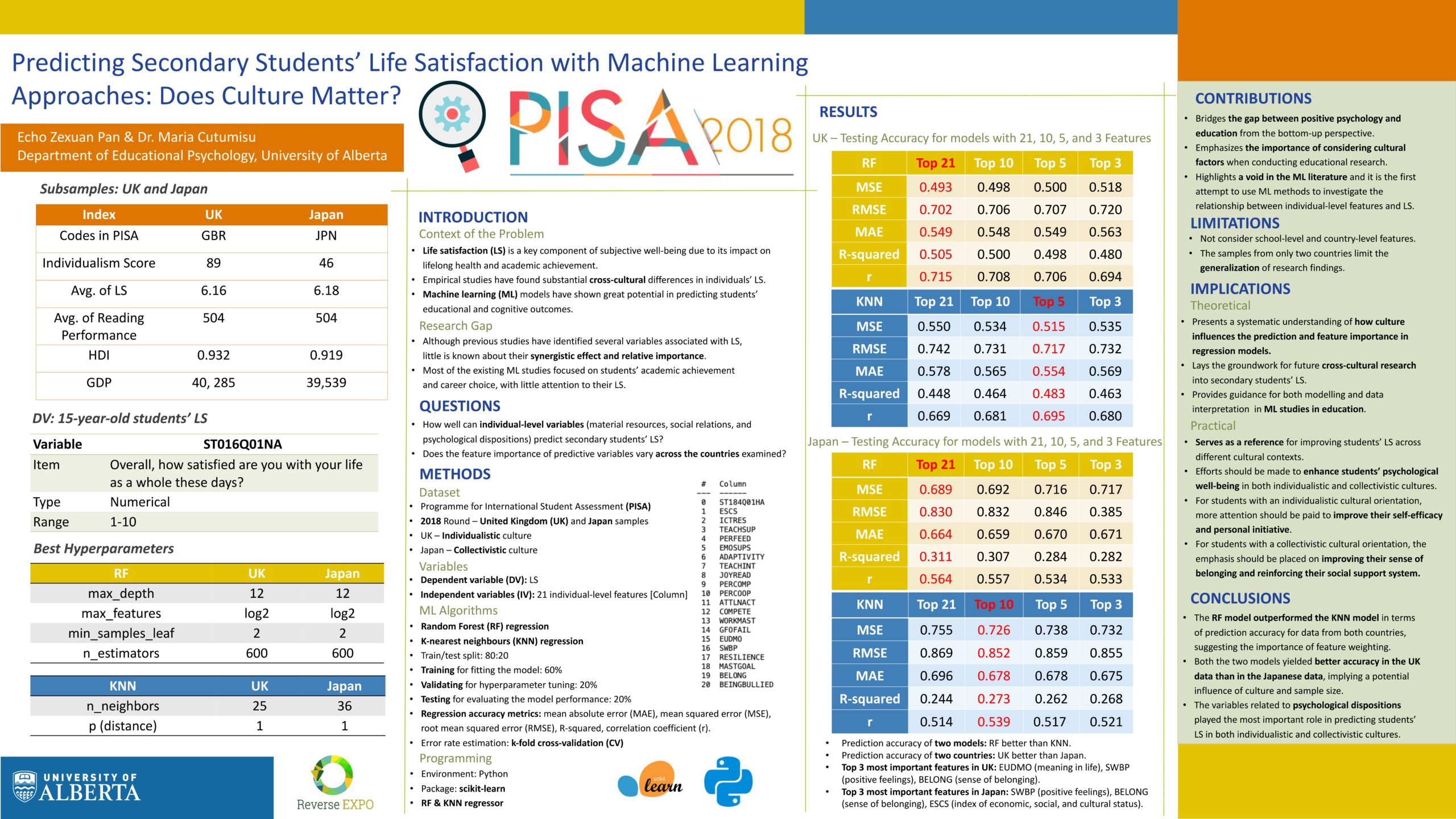
A Machine Learning Approach to Identify Factors Affecting PISA 2018 Digital Reading Literacy
Researchers: Hao-Yue Jin, Lydia González Esparza – Supervisor: Maria Cutumisu
Digital reading literacy is an essential skill for 21st-century citizens who engage in complex digital-based communication on a daily basis. Recent studies revealed that adolescents struggle with evaluating digital content despite using it frequently to communicate. Thus, the present study aims to identify factors affecting the digital reading literacy of n = 37,514 15-year-old students from 33 countries participating in the PISA 2018 using the Lasso and Random Forest machine learning algorithms. Findings showed that Lasso outperformed Random Forest in predicting students’ digital reading literacy and revealed that Meta-Cognition: Assess Credibility and Availability of ICT Devices were the most salient factors associated with digital reading literacy. Thus, instructors and administrators could consider teaching students how to use metacognitive strategies and providing more ICT opportunities that promote digital reading literacy.
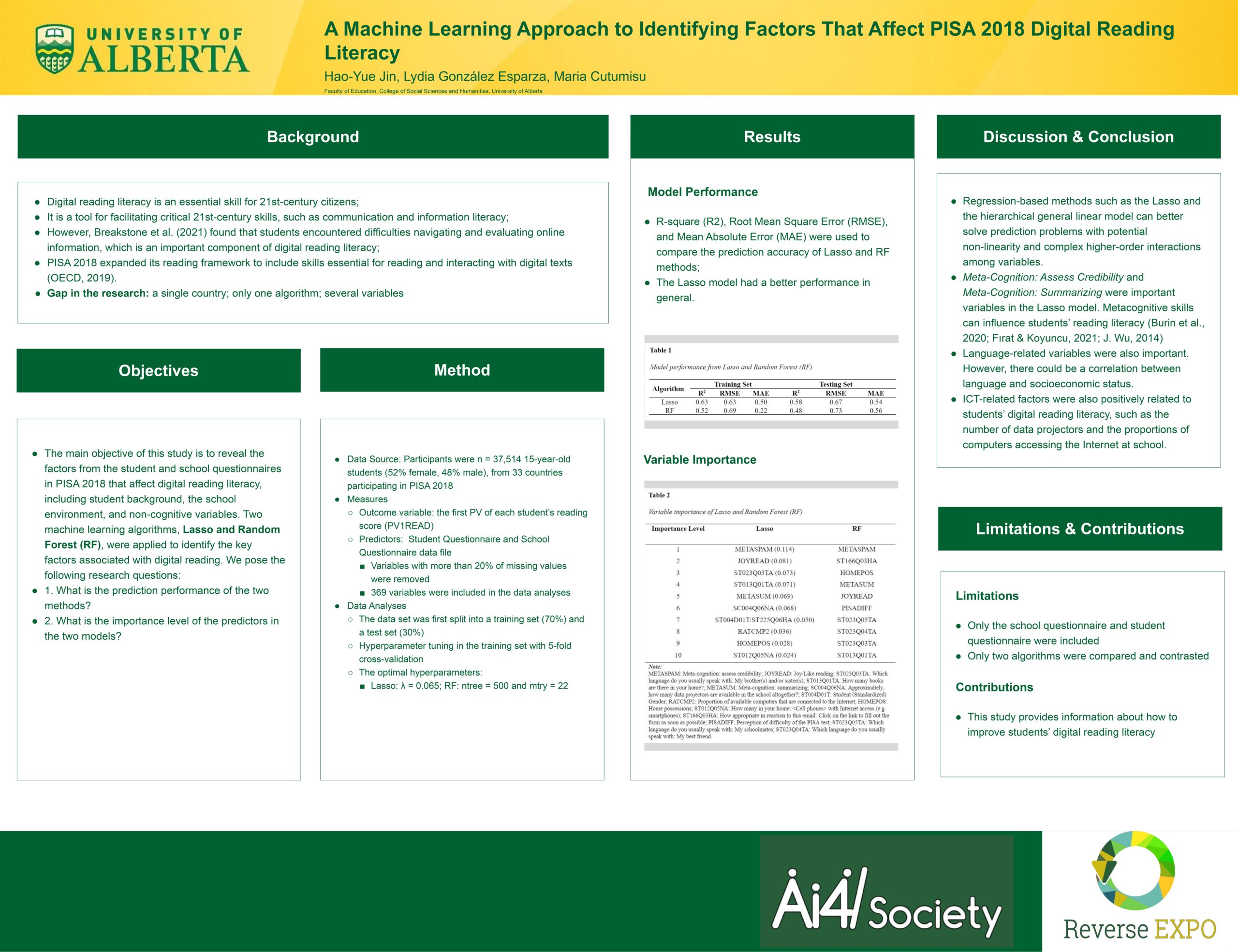
Conversation-based assessment: Measuring student learning with conversational agents
Researcher: Seyma N. Yildirim-Erbasli | Supervisors: Okan Bulut, Ying Cui
One of the main goals of educational assessments is to obtain valid scores that reflect students’ true ability levels. A major threat against the validity is the presence of non-effortful responses from unmotivated students. Due to insufficient interaction between learners and the non-interactive assessment environment, some students may get disengaged and fail to show effortful response behavior. Unlike non-interactive assessments, interactive assessments (e.g., assessments involving one-on-one tutoring) can keep the students engaged in the assessment process. However, implementing interactive assessments in practice can be very challenging. Although such assessments are easy to use with a small number of students, they become more expensive and harder to implement with a large group of students. A promising solution to this problem is to create a conversation-based assessment (CBA) harnessing artificial intelligence (AI). The purposes of this study are twofold: (1) create an AI-based CBA that measures student learning and provides feedback; (2) examine students’ attitudes towards CBA using an online survey. The CBA is designed for an undergraduate course using Rasa and deployed to a user-friendly environment, Google Chat. The findings help us understand the potential of CBAs in advancing conventional assessments (e.g., computer-based assessments) and increasing student motivation through interactivity.
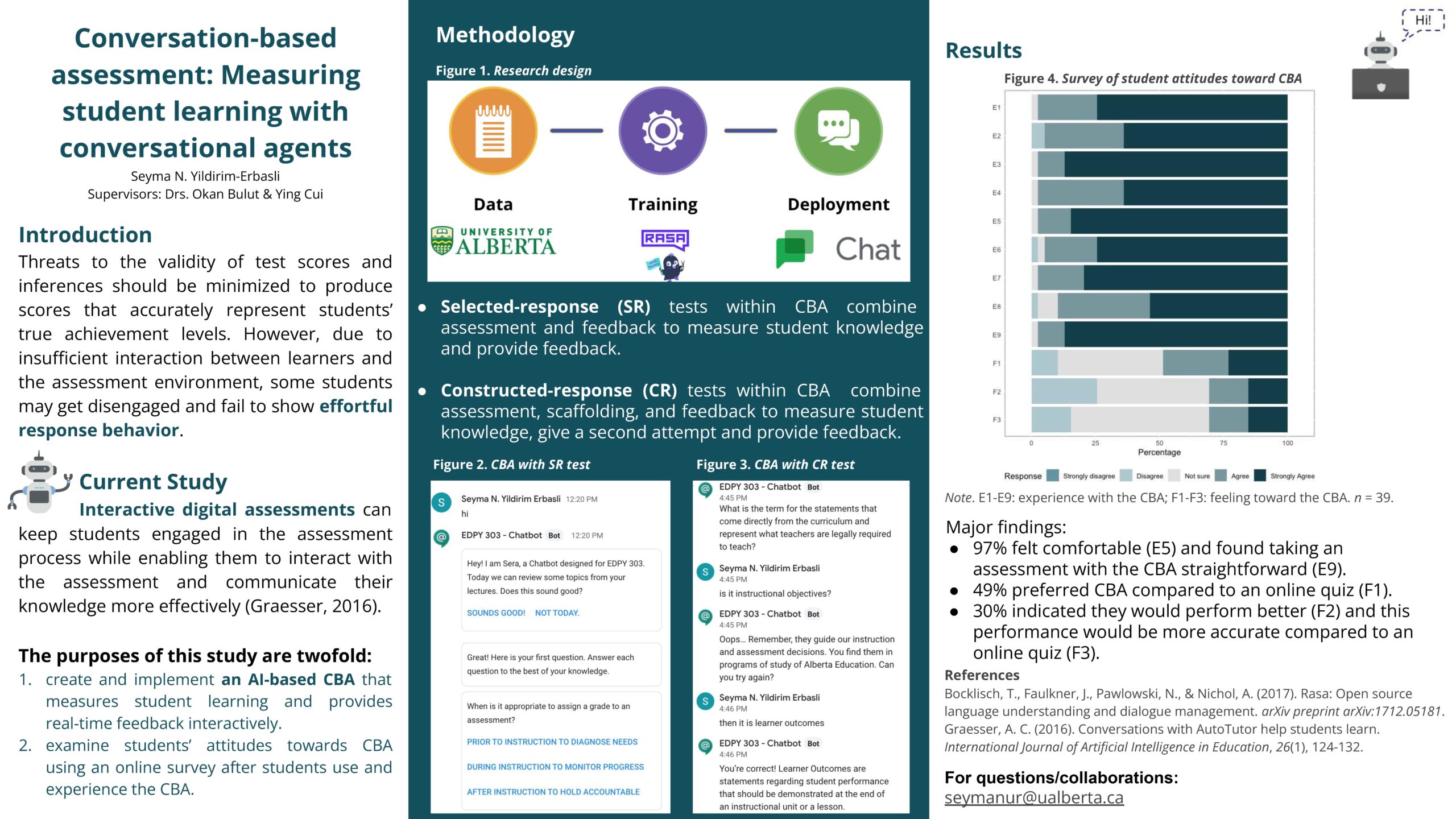
The Application of Artificial Intelligence in Educational Feedback
Researcher: Tarid Wongvorachan & Ka Wing Lai | Supervisors: Okan Bulut, Yi-Shan Tsai & Guanliang Chen
Feedback—a process where learners make sense of the provided information to reduce the gap between their current and desired performance—is a crucial component of student learning (Carless & Boud, 2018). As advancements in technology have enabled new ways of learning and changed the dynamics of education (e.g., the transition from traditional paper-and-pencil assessments to digital online assessments), the use of Artificial Intelligence (AI) has also made its way into feedback practice such as the use of Educational Data Mining (EDM) and natural language processing (NLP) to evaluate student performance in real-time and generate personalized feedback, or the use of Learning Analytics (LA) to develop analytics dashboards for both students and instructors (Jimenez & Boser, 2021; Tsai et al., 2021).
However, previous studies on educational feedback mainly focused on the conceptual part of feedback practices, and empirical evidence on the use of AI for feedback purposes is also scattered potentially due to the rapid development of technology. To inform both researchers and practitioners on the application of AI to producing personalized feedback, we propose a new framework by identifying and organizing potential areas for the use of EDM, NLP, and LA in feedback practices to establish venues of AI research and practice in educational feedback.
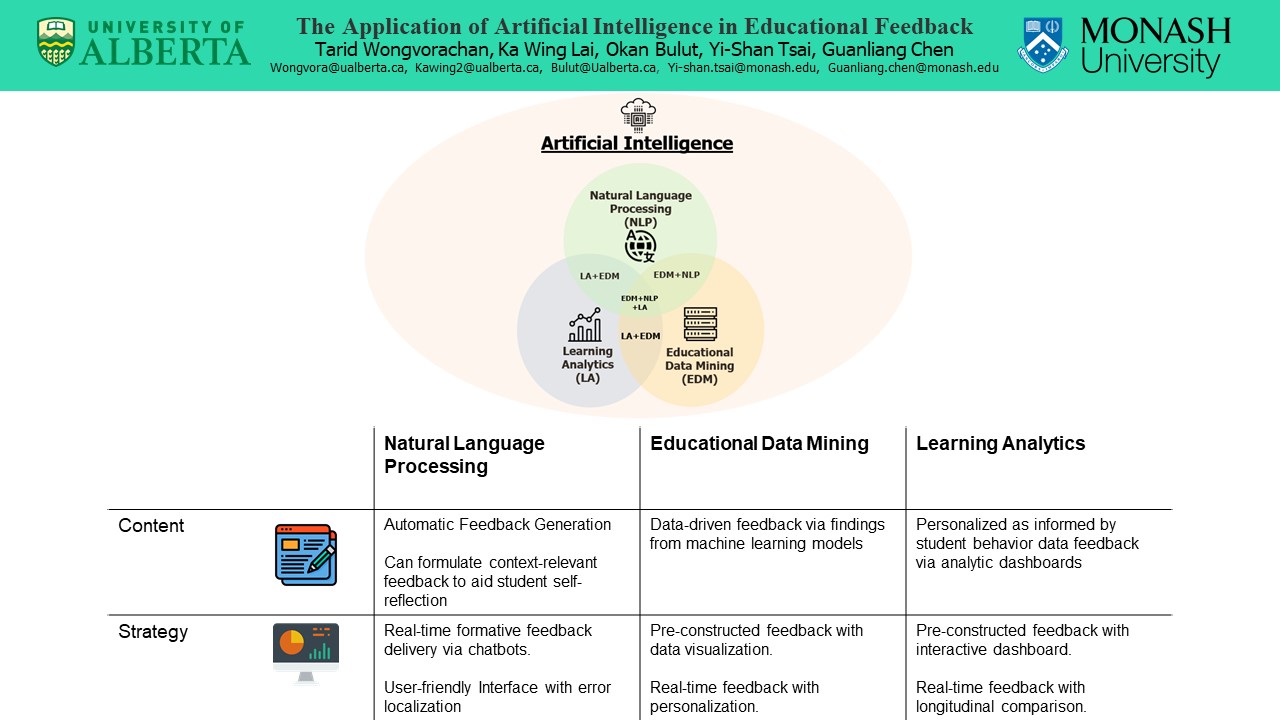
AI and Social Media Identity
Project description:
Supervisor:
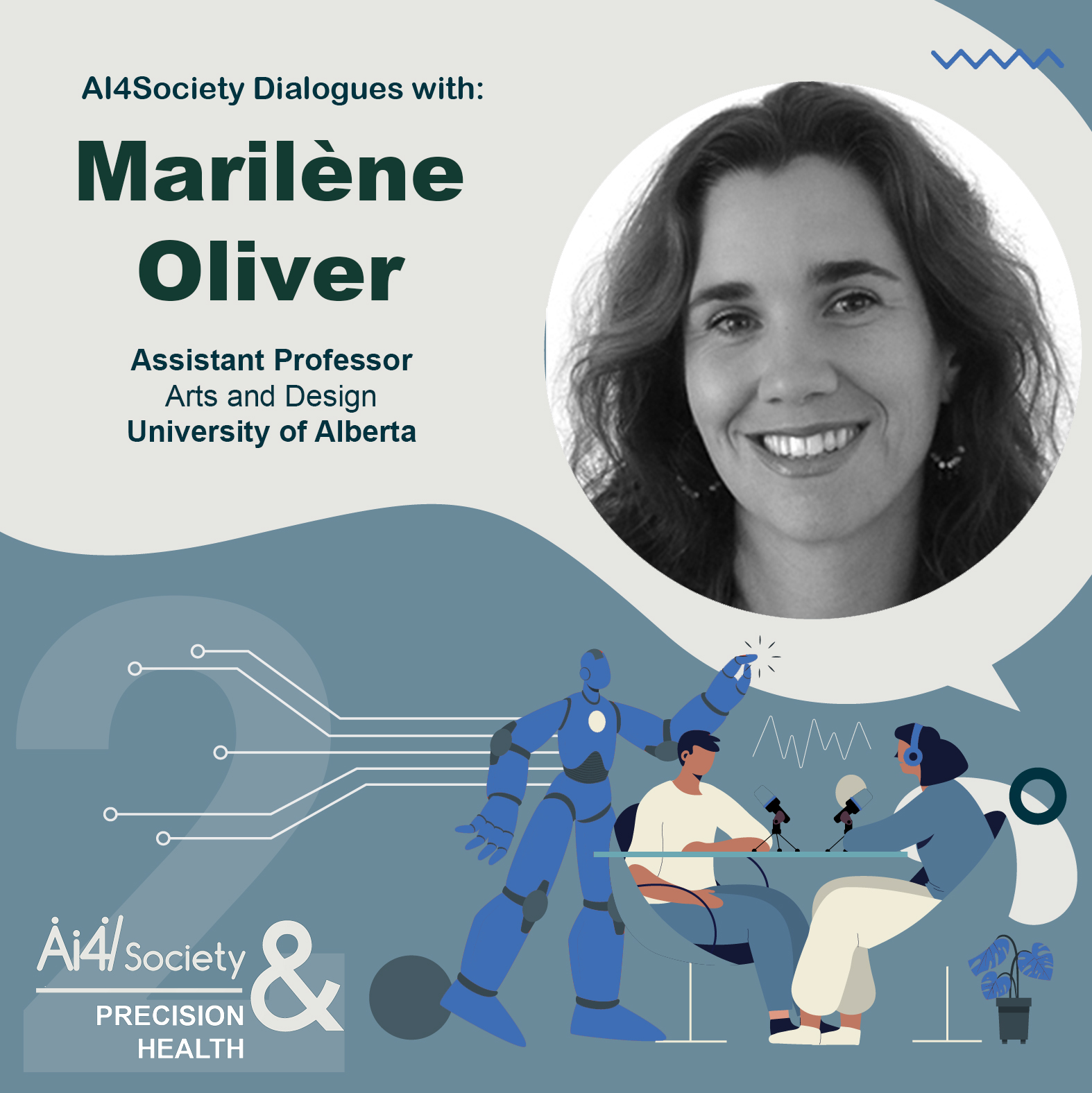
Description:
Supervisor:
Description:
Developing games is challenging as it requires expertise in programming skills and game design. This process is costly and consumes a great deal of time. In this paper we describe a tool we developed to help decrease this burden: Mechanic Maker. Mechanic Maker is designed to allow users to make 2D games without programming. A user interacts with a machine learning AI agent, which learns game mechanics by demonstration.
sdafsdf
Researcher: Vardan Saini
Supervisor: Matthew Guzdial
Developing games is challenging as it requires expertise in programming skills and game design. This process is costly and consumes a great deal of time. In this paper we describe a tool we developed to help decrease this burden: Mechanic Maker. Mechanic Maker is designed to allow users to make 2D games without programming. A user interacts with a machine learning AI agent, which learns game mechanics by demonstration.
A Demonstration of Mechanic Maker: An AI for Mechanics Co-Creation
Researcher: Vardan Saini | Supervisor: Matthew Guzdial
Developing games is challenging as it requires expertise in programming skills and game design. This process is costly and consumes a great deal of time. In this paper we describe a tool we developed to help decrease this burden: Mechanic Maker. Mechanic Maker is designed to allow users to make 2D games without programming. A user interacts with a machine learning AI agent, which learns game mechanics by demonstration.
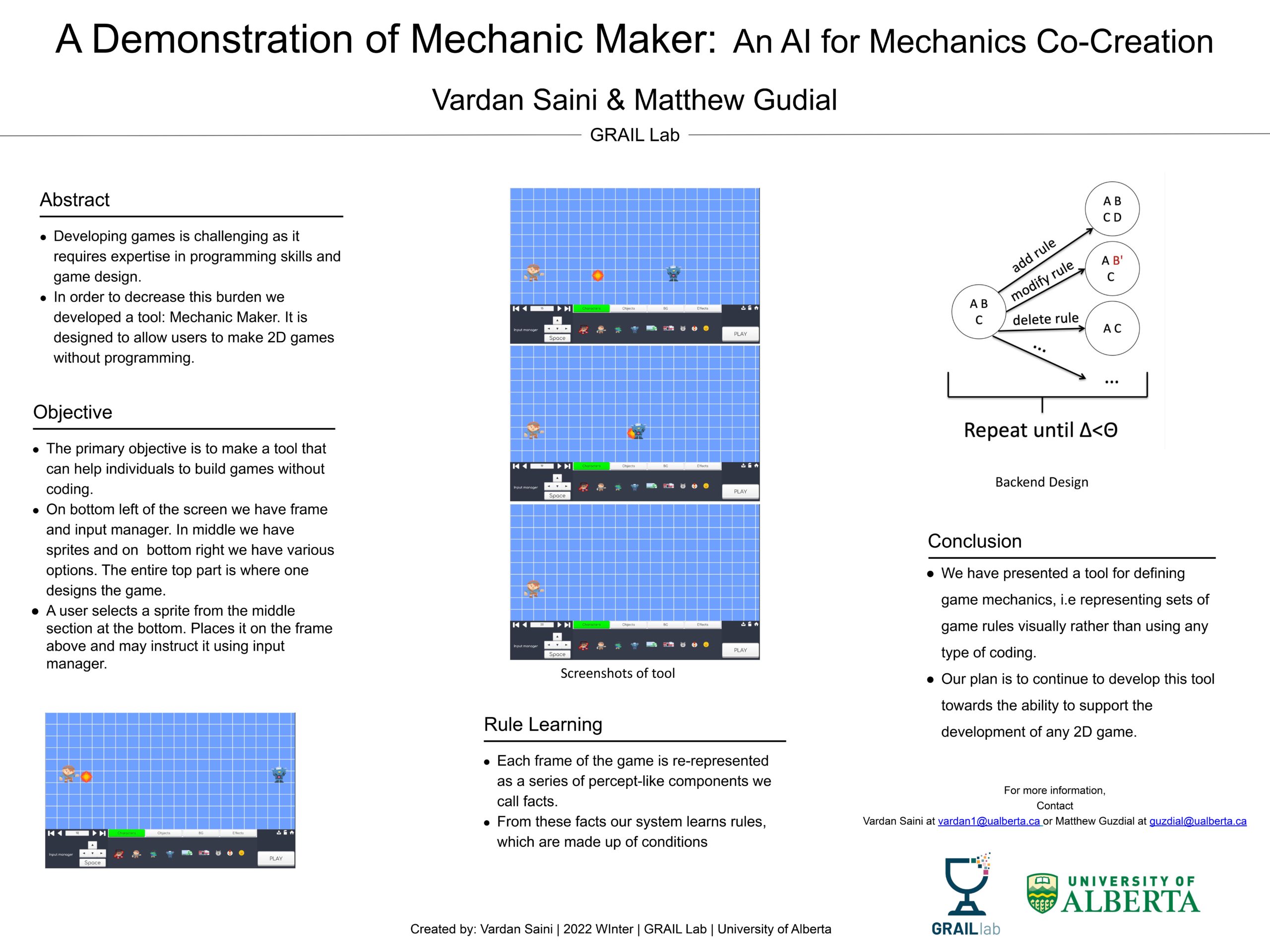
More at: https://taridwong.github.io/
Natural and Applied Science
- Natural and Applied Sciences
- Akeem Afuwape
- Christoph Sydora 1
- Christoph Sydora 2
- Ehsan Hashemi
- Elyar Pourrahimian
- Hadis Ebrahimi
- Jashwanth Sarikonda
- Mona Ahmadi Rad
- Narges Sajadfar
- Nima Narjabadifam
- Saidur Rahman
- Shahin Atakishiyev
- Shehroze Khan
- Sourav Sarkar
Natural and Applied Sciences
14 projects related to Society, Energy, Housing, and more!
Click on the tabs in the left to access the material of each presenter
Exploring the potentials of AI in sustainable built environments
Researcher: Akeem Afuwape | Supervisors: Jenn McArthur, Eleni Stroulia
The emergence of Industry 4.0 has led to the development of cyber-physical building and facilities capable of leveraging data from embedded sensors, and IoT platforms to drive innovation, and process optimization. At Ryerson’s Smart Buildings Research Group (SBRG), the Daphne Cockwell Complex (DCC) building has undergone extensive metering to provide real-time streaming data to aid the development of data-driven solutions. Algorithms have been developed for the optimization of energy consumption, fault detection and diagnosis of building systems, and other facility management processes. Deep learning models and classification algorithms have been used to identify Building Automation System time series from HVAC systems with over 85% accuracy. The algorithms learn the underlying characteristics of given time series and show potential for use in system events or fault detection.
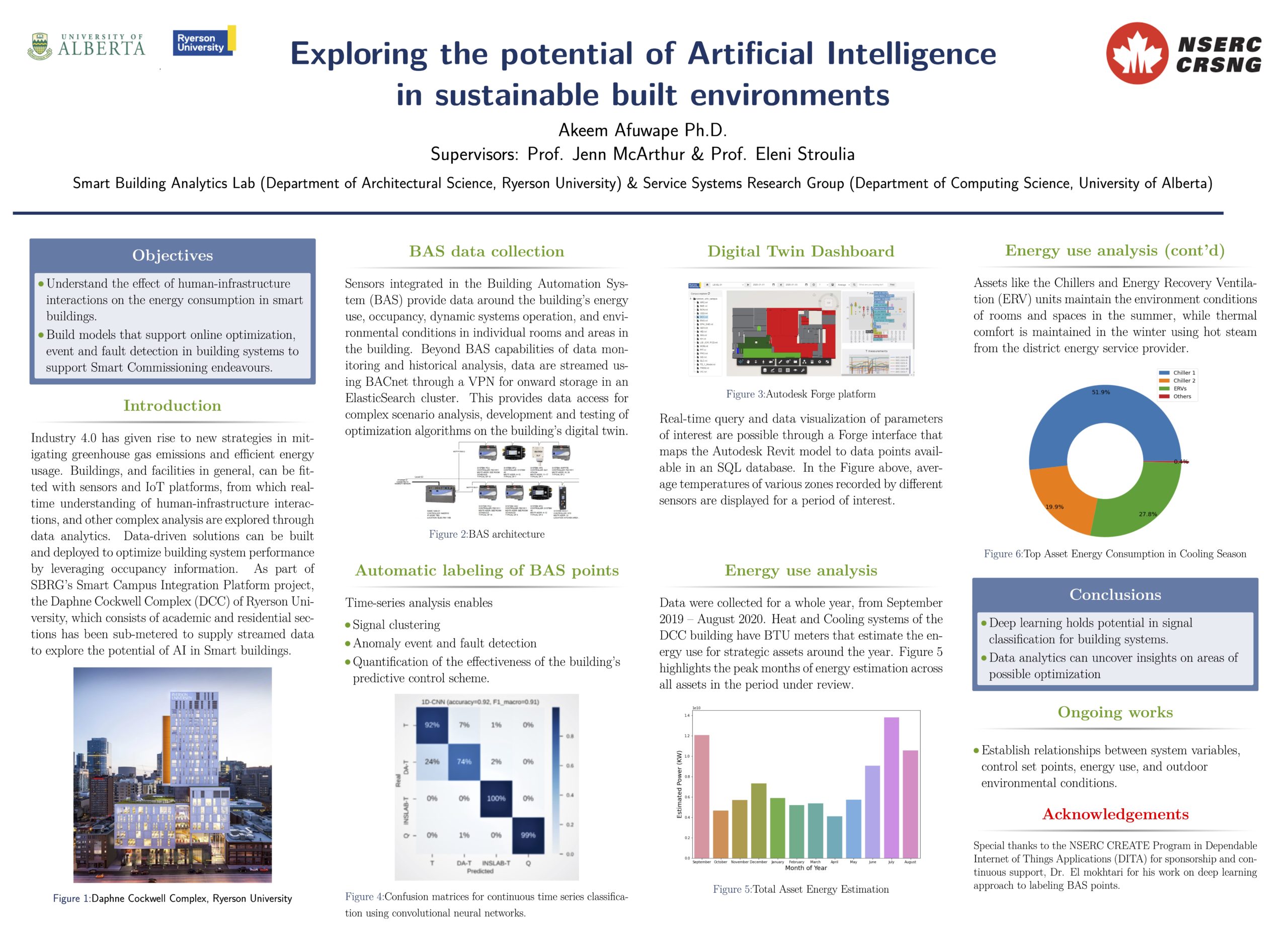
Data-Driven Decision-Support approach For Construction Equipment Replacement
Researcher: Elyar Pourrahimian | Supervisor: Simaan AbouRizk
The objective of this research is to develop a data driven decision support approach for construction equipment replacement to make use of existing data in company’s database to support the equipment replacement decision.
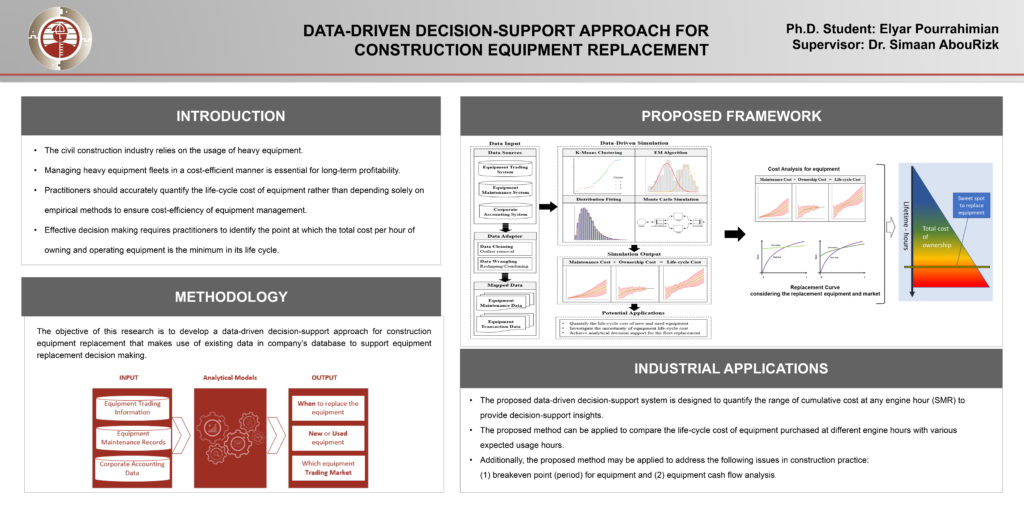
Evaluating machine learning performance in predicting the potential consequences of hazardous materials release in railway incidents
Researchers: Hadis Ebrahimi, Fereshteh Sattari | Supervisors: Lianne Lefsrud, Renato Macciotta
A large volume of hazardous materials (hazmat) shipments is transported by railway in Canada. Railway transportation of hazmat is dangerous, and severe consequences, even with low probability, may happen to people in railway incidents. Risk assessment is necessary to control and reduce the consequences of railway incidents. Besides the traditional methods of risk assessment, machine learning provides new ways to manage new situations and to find new knowledge. The main purpose of this study is to propose a reliable machine learning model to predict the potential consequences of railway incidents transporting hazmat. Regression algorithms, including neural networks, linear regression, decision trees, and support vector machine (SVM) are employed to predict the potential consequences of railway incidents transporting hazmat. Railway occurrences database system (RODS) is used to find the input and output variables. Input variables are including, the primary causes of railway incidents, the weather conditions, the conditions of the track, etc., and the output variables are including, the number of injuries, fatality, evacuation, and the total damage cost in the railway incidents. Principal Component Analysis (PCA) method is used to identify the variables that have the most significant effects on potential consequences of railway incidents. Then, the performances of the different machine learning algorithms are compared to find the most reliable method. The result of this study shows that the consequences of railway incidents transporting hazmat are not random, and there are underlying patterns and trends between data that need to be extracted. The findings of this study can help decision-makers towards safe operations of hazmat railway transportation.
Optimizing the urban buildings solar rooftop potential considering economic and environmental visions of stakeholders: using computer vision techniques
Researcher: Nima Narjabadifam, Mohammed Al-Saffar, Yongquan Zhang, Joseph Nofech, Asdrubal Cheng Cen, Hadia Awad, Michael Versteege | Supervisor: Mustafa Gül
Implementing renewable energy sources (RES) into buildings at the microgeneration level to mitigate GHG emissions and make societies sustainable has been widely accepted as an effective strategy. Keeping this in mind, we try to find the optimum layout of solar photovoltaic (PV) systems regarding economic and environmental aspects at the same time. First, an automated method using computer vision techniques is developed to identify and classify roofs of urban buildings to extract available roofs area based on buildings’ 3D models, considering their complex shapes and existing obstacles. Then, the extracted roofs with the mentioned details are used to find the optimal layout of PV systems on them that simultaneously satisfies stakeholders’ economic and ecological visions. This work is applied to the North Campus buildings of the University of Alberta as the case study.
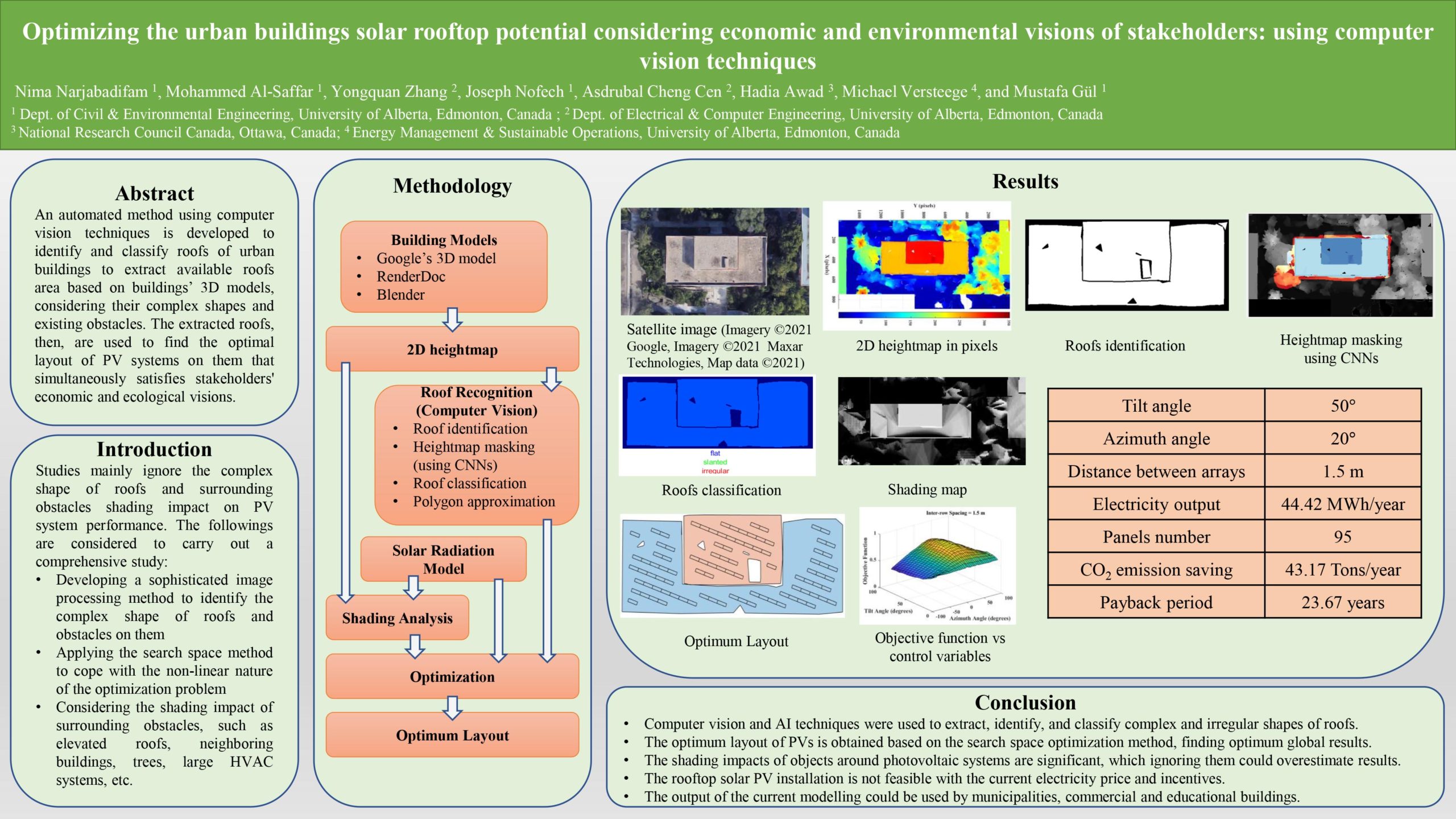
Two-Stage Stochastic Optimization of a Virtual Power Plant
Researcher: Saidur Rahman | Supervisor: Omid Ardakanian
We propose two profit-maximizing operating strategies for a virtual power plant (VPP) that aggregates electric vehicle (EV) chargers with vehicle-to-grid (V2G) support and local solar systems, and trades in day-ahead and imbalance electricity markets. Both strategies solve a two-stage stochastic optimization problem. In first stage, hourly energy bids are placed in day-ahead market by solving a mixed-integer linear program, given the VPP’s predictions for next day. In second stage, decisions with respect to charging or discharging EVs are made sequentially for every hour, and adjustments to day-ahead commitments are settled in imbalance market. The two strategies differ in how they solve
the sequential decision making problem in second stage. They both foresee the effects of their charge and discharge decisions on feasibility of fulfilling the EV charging demands using one-step lookahead technique. The first strategy employs a heuristic algorithm that finds a feasible charging schedule for each EV while maximizing VPP’s profit. The second one utilizes a soft actor-critic reinforcement learning method with a differentiable projection layer that enforces constraint satisfaction. We evaluate the performance of these algorithms using real market and EV charging data, and analyze sensitivity of the VPP’s profit to percentage of EVs that participate in V2G.
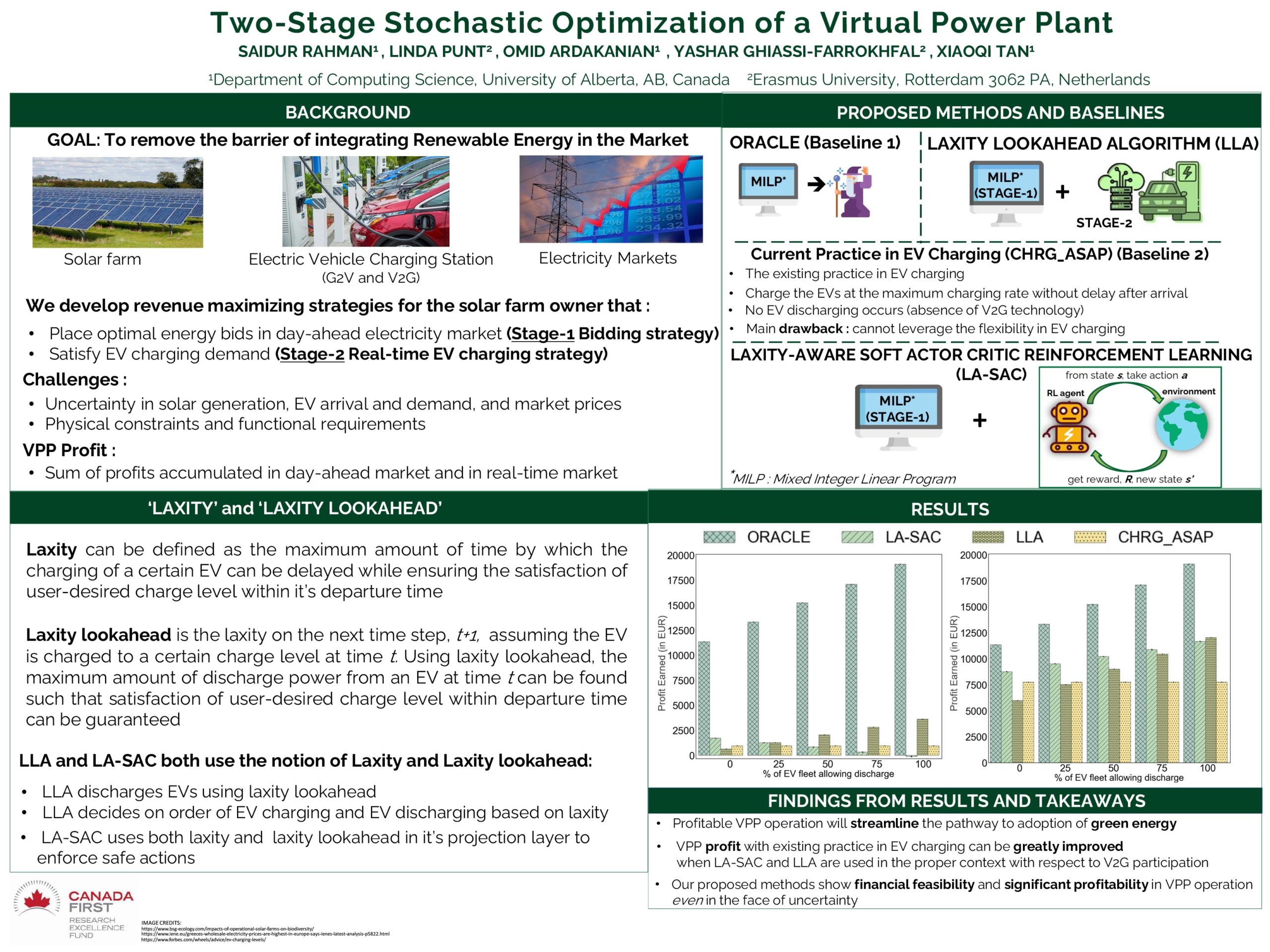
Disinformation, Stochastic Harm, and Costly Effort: A Principal-Agent Analysis of Regulating Social Media Platforms
Researcher: Shehroze Khan, James R. Wright
The spread of disinformation on social media platforms is harmful to society. This harm may manifest as a gradual degradation of public discourse; but it can also take the form of sudden dramatic events such as the recent insurrection on Capitol Hill. The platforms themselves are in the best position to prevent the spread of disinformation, as they have the best access to relevant data and the expertise to use it. However, mitigating disinformation is costly, not only for implementing detection algorithms or employing manual effort, but also because limiting such highly viral content impacts user engagement and thus potential advertising revenue. Since the costs of harmful content are borne by other entities, the platform will therefore have no incentive to exercise the socially-optimal level of effort. This problem is similar to that of environmental regulation, in which the costs of adverse events are not directly borne by a firm, the mitigation effort of a firm is not observable, and the causal link between a harmful consequence and a specific failure is difficult to prove. For environmental regulation, one solution is to perform costly monitoring to ensure that the firm takes adequate precautions according to a specified rule. However, a fixed rule for classifying disinformation becomes less effective over time, as bad actors can learn to sequentially and strategically bypass it.
Encoding our domain as a Markov decision process, we demonstrate that no penalty based on a static rule, no matter how large, can incentivize adequate effort. Penalties based on an adaptive rule can incentivize optimal effort, but counterintuitively, only if the regulator sufficiently overreacts to harmful events by requiring a greater-than-optimal level of effort. We prescribe the design of mechanisms that elicit platforms’ costs of precautionary effort relating to the control of disinformation.
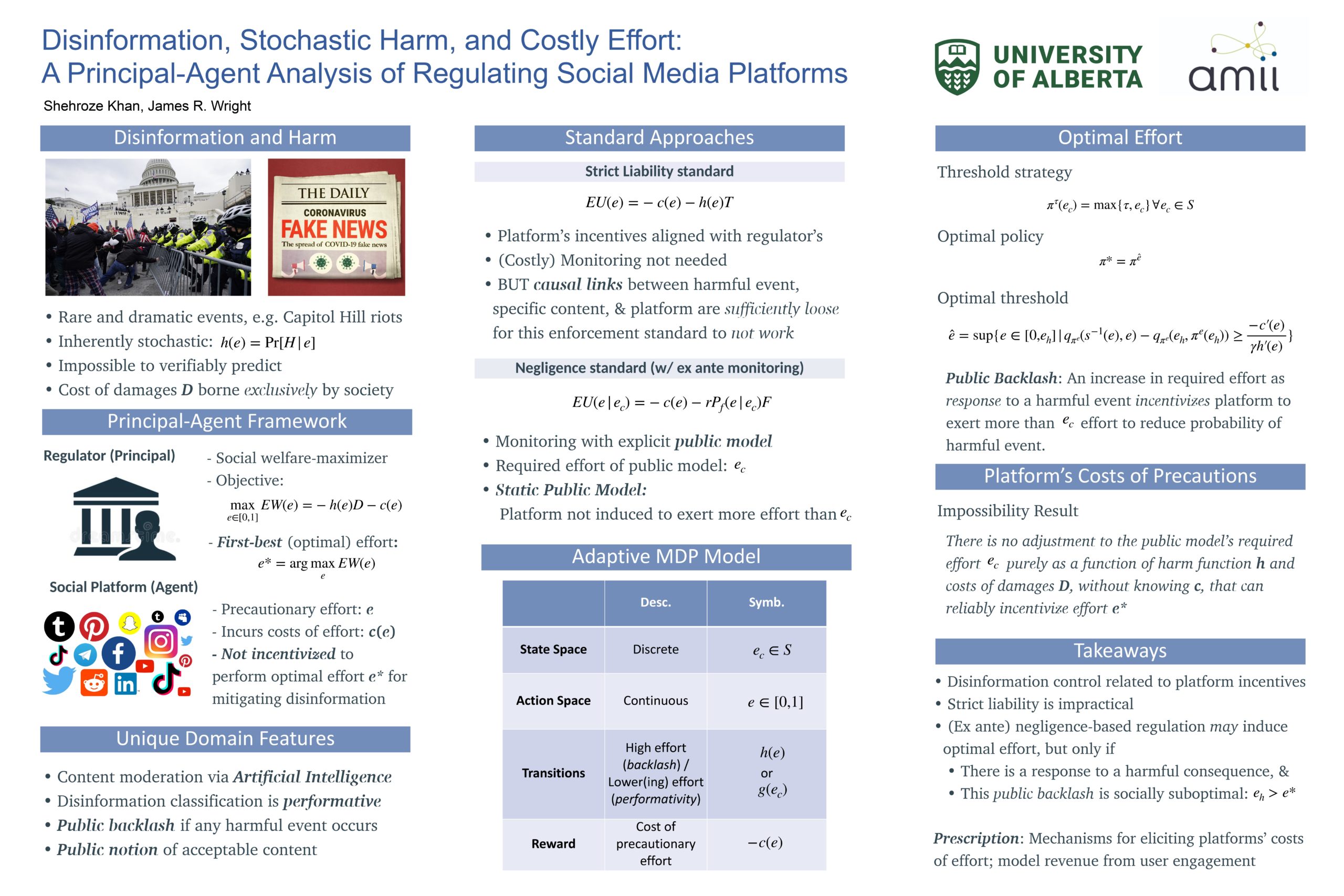
Neutrino Event Classification Using Graph Neural Network
Researcher: Sourav Sarkar | Supervisor: Roger Moore
The IceCube neutrino observatory is a Cherenkov detector located at the geographic South Pole and uses a cubic kilometer of the Antarctic ice as the detector volume by instrumenting 3D arrays of photosensors called digital optical modules (DOM). The charged secondary particles produced from the neutrino interactions in ice emit Cherenkov light and are detected by the DOMs. The type of neutrino interaction can then be identified based on the spatiotemporal topology of the detected light. In this project, we are building an event classification method using graph convolutional neural network (GCNN) to identify a rare type of neutrino interaction called trident production. Limited by the detector resolution, the detected light patterns in most of the DOMs for the rare trident events look very similar to one of the major background events and thus, pose a challenge for building high efficacy classifier. Using high-level physics properties as model input through feature engineering, we have achieved moderately high performance (~82% a.u.c. score). In addition, we have explored the application of a differentiable graph pooling method called DIFFPOOL that learns on the hierarchical graph representation and improved the model’s performance by ~4% (~86% a.u.c. score).
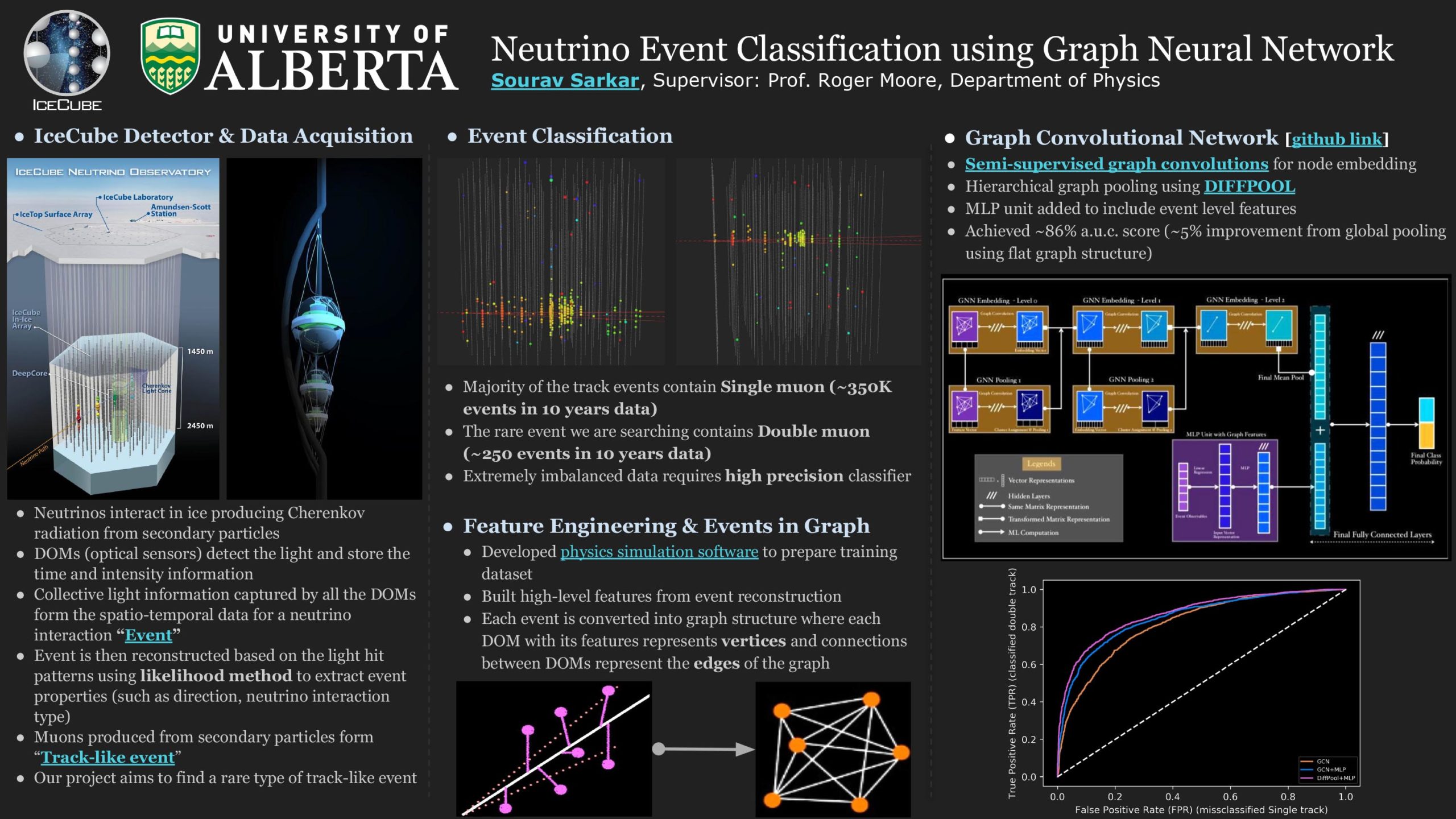
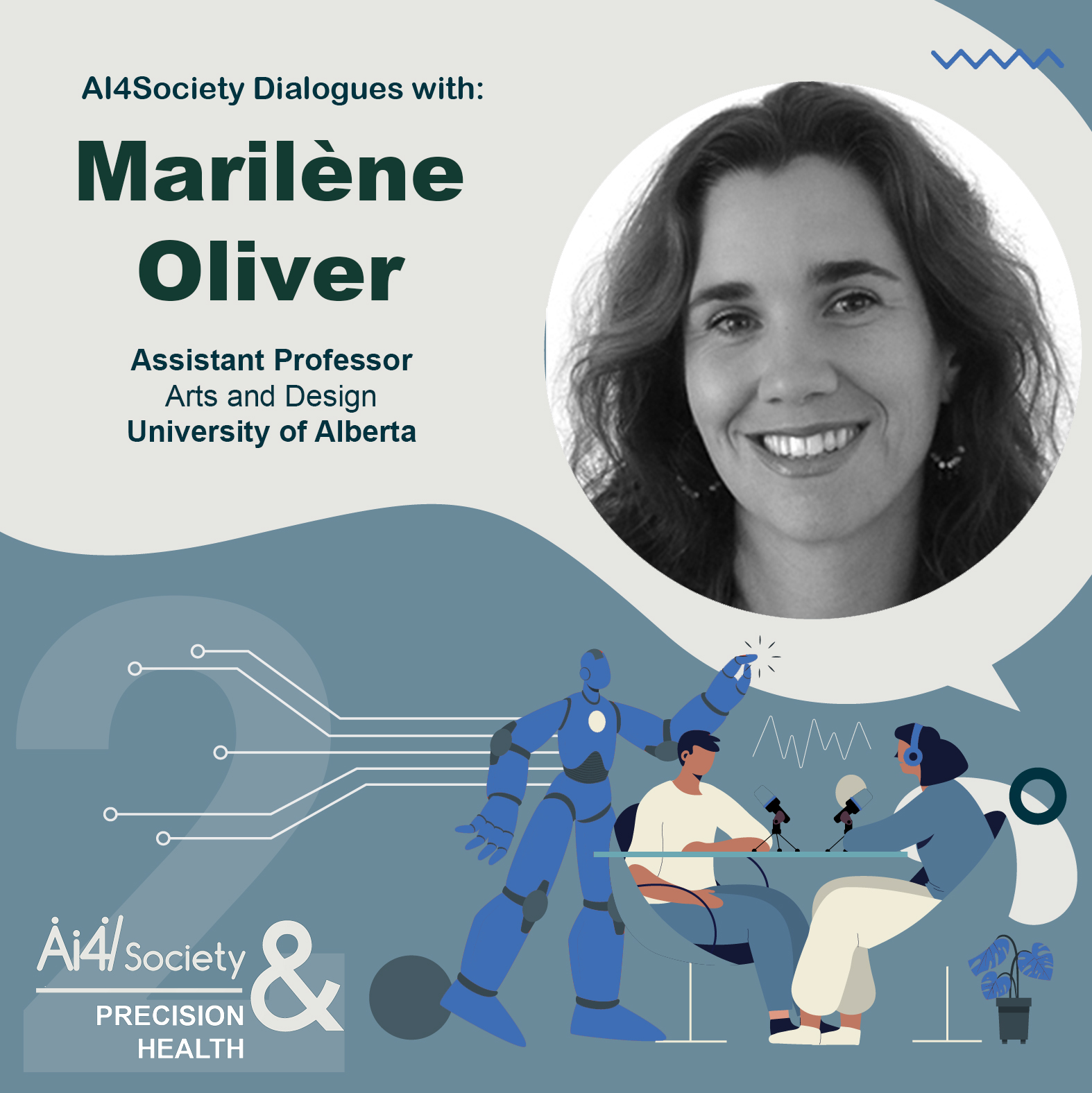
Description:
Supervisor:
Description:
Developing games is challenging as it requires expertise in programming skills and game design. This process is costly and consumes a great deal of time. In this paper we describe a tool we developed to help decrease this burden: Mechanic Maker. Mechanic Maker is designed to allow users to make 2D games without programming. A user interacts with a machine learning AI agent, which learns game mechanics by demonstration.
sdafsdf
Researcher: Vardan Saini
Supervisor: Matthew Guzdial
Developing games is challenging as it requires expertise in programming skills and game design. This process is costly and consumes a great deal of time. In this paper we describe a tool we developed to help decrease this burden: Mechanic Maker. Mechanic Maker is designed to allow users to make 2D games without programming. A user interacts with a machine learning AI agent, which learns game mechanics by demonstration.
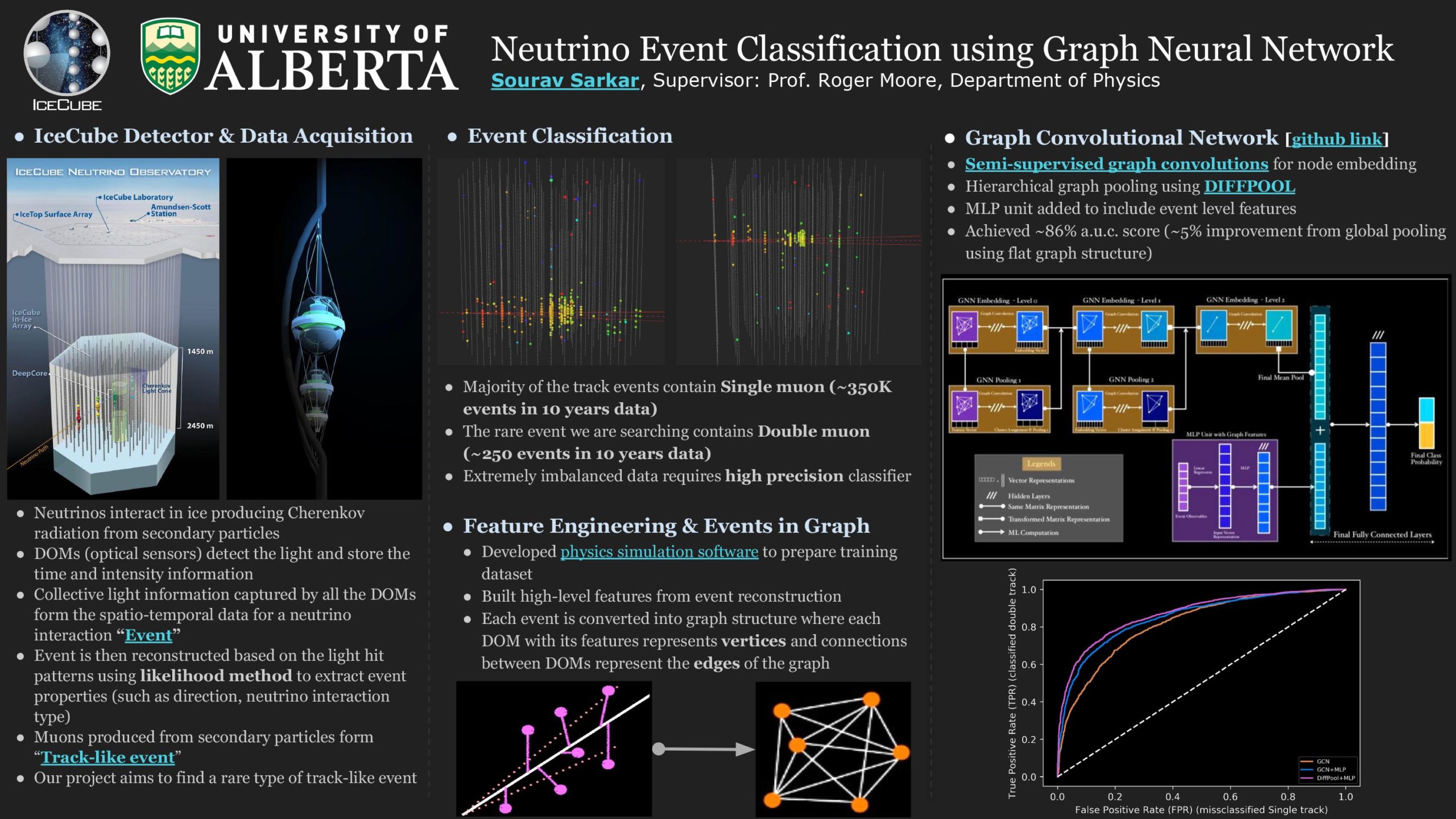
Algorithms
- Algorithms
- Abhineet Singh
- Akash Saravanan
- Andrea Whittaker
- Bennett Tchoh
- Candelario Gutierrez
- Chuan Guo
- Fatemeh Tavakoli
- Maryam Mirzaei
- Peiran Yao
- Pete Jones
- Sara Elkerdawy
- Siyuan Yu
- Talha Ibn Aziz
- Yuyang Nie
- Zahra Bashir
Algorithms
15 projects related to Machine Learning, Modeling, Datasets, and more!
Click on the tabs in the left to access the material of each presenter
Inducible Pluripotent Stem Cell (IPSC) Viability Prediction from Time-Lapse Microscopy Images
Researcher: Abhineet Singh | Supervisor: Nilanjan Ray
The objective of this work is to develop a deep learning pipeline to make an early prediction of the viability of IPSCs to develop into insulin producing islet cells for type 1 diabetes patients. IPSCs have a minuscule viability rate of around 1 in 10,000 which makes it extremely costly to produce even a small number of usable cells since that requires culturing millions of cells to later stages of growth. The chemical assaying process needed to make this determination is also very tedious and labor-intensive. The ability to reliably predict which cells are likely to be viable at an early stage would therefore be ground-breaking in rendering this a practical and cost-effective treatment for type 1 diabetes.
Temporal information about the way that the appearance of a cell changes over time is crucial for predicting its future growth outcome. This data is collected by capturing time-lapse images of cell cultures from an ultra-high resolution microscope. These images are then manually annotated to mark the locations and identities of cells in order to train deep neural networks to perform automatic cell segmentation and tracking. Finally, cell classification is performed by multi-frame analysis of cell appearance through sequential deep networks.
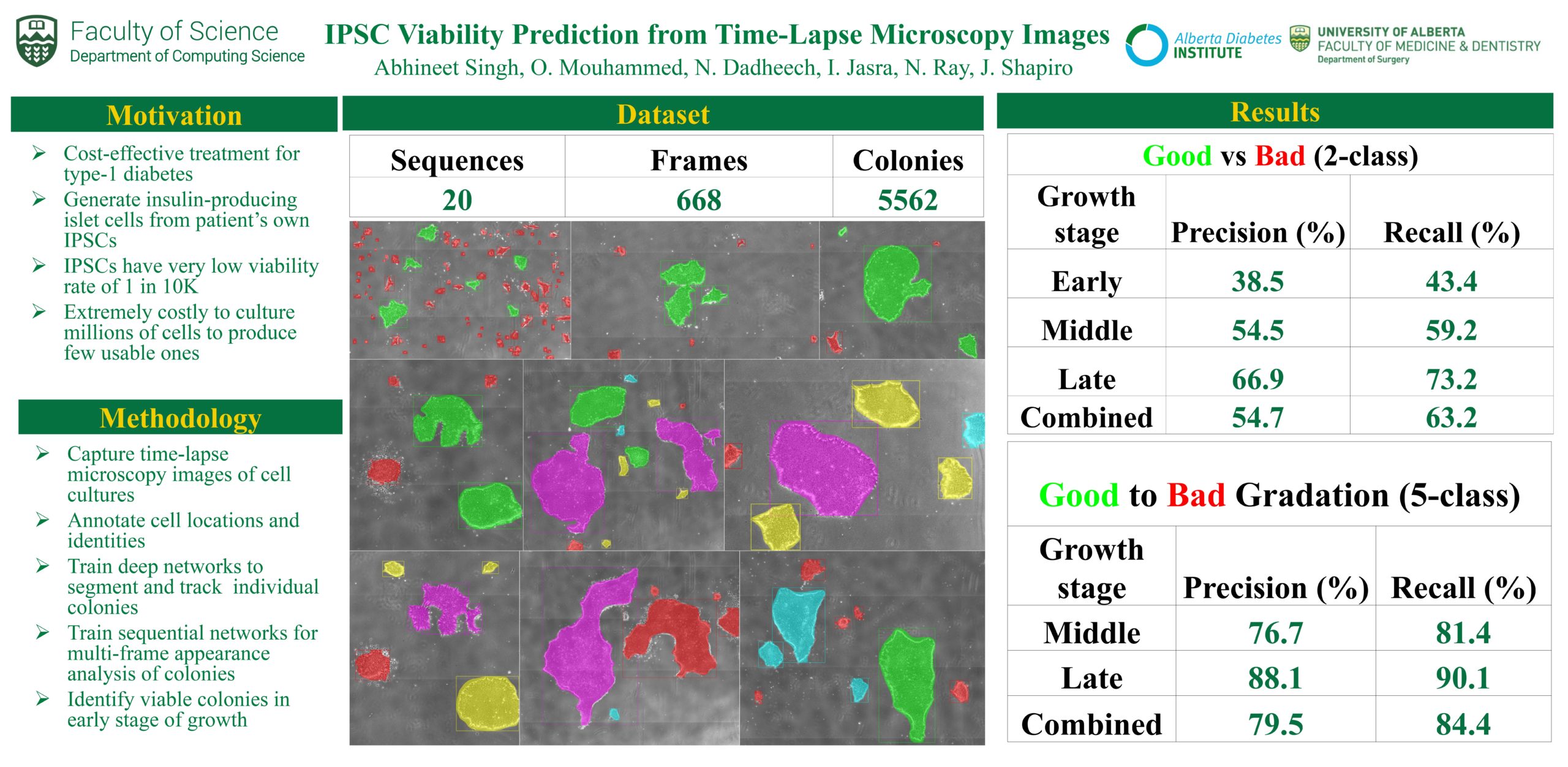
Pixel VQ-VAEs for Improved Pixel Art Representation
Researcher: Akash Saravanan | Supervisor: Matthew Guzdial
Machine learning has had a great deal of success in image processing. However, the focus of this work has largely been on realistic images, ignoring more niche art styles such as pixel art. Additionally, many traditional machine learning models do not work well with pixel art due to its distinct properties. We propose the Pixel VQ-VAE, a specialized VQ-VAE model that learns representations of pixel art. We show that it outperforms other models in both the quality of embeddings as well as performance on downstream tasks.
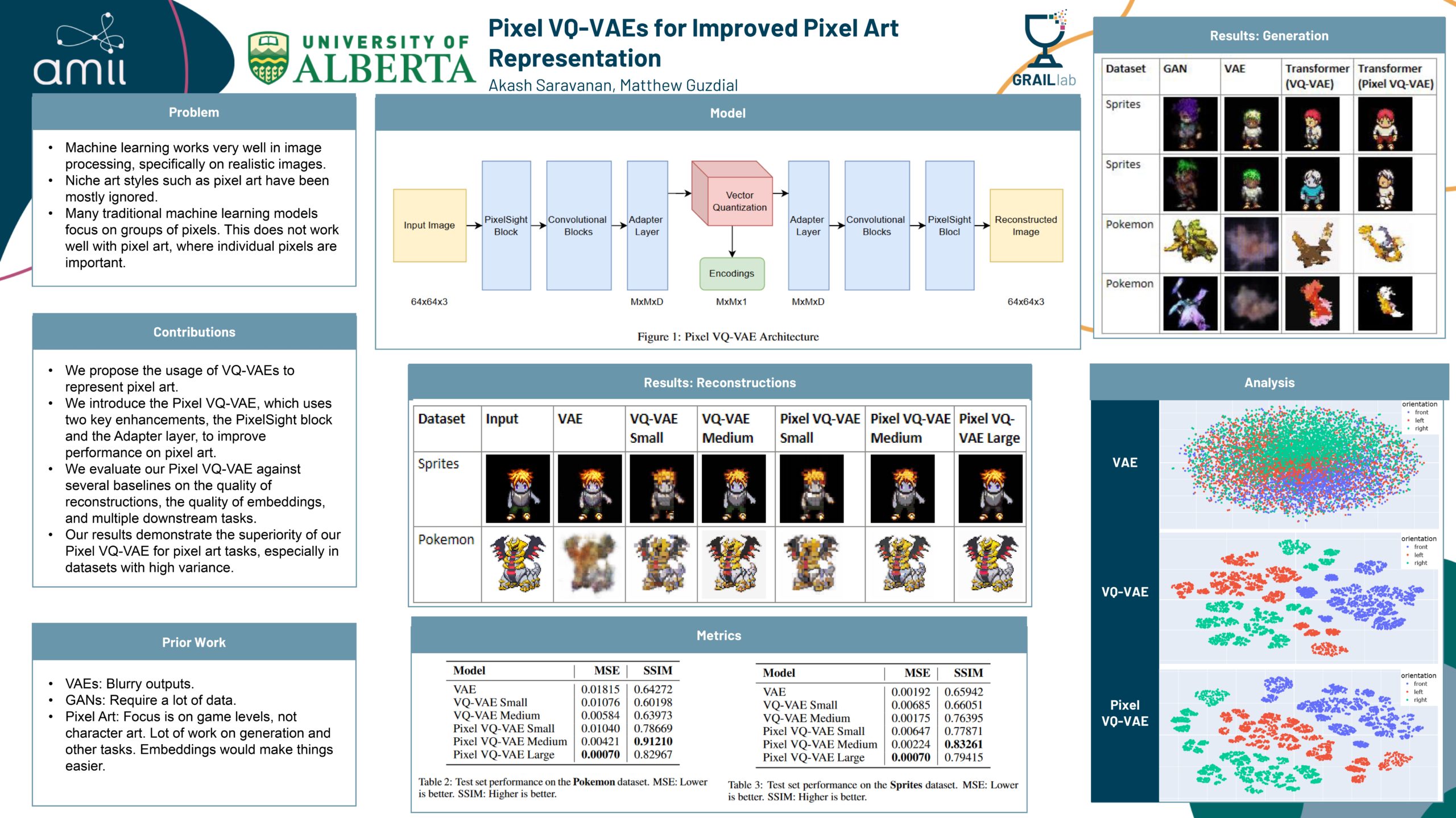
ADaPT-ML: A Data Programming Template for Machine Learning
Researcher: Andrea Whittaker | Supervisor: Denilson Barbosa
Classification is a task that involves making a prediction about which class(es) a data point belongs to; this data point can be text, an image, audio, or can even be multimodal. This task can become intractable for many reasons, including:
• Insufficient training data to create a data-driven model; available training data may not be appropriate for the domain being studied, it may not be of the right type (e.g. only text but you want text and images), it may not have all of the categories you need, etc.
• Lack of available annotators with domain expertise, and/or resources such as time and money to label large amounts of data.
• Studying a phenomenon that changes rapidly, so what constitutes a class may change over time, making the available training data obsolete.
ADaPT-ML is a multimodal-ready MLOps system that covers the data processing, data labelling, model design, model training and optimization, and endpoint deployment, with the particular ability to adapt to classification tasks that have the aforementioned challenges. ADaPT-ML is designed to accomplish this by:
• Using Snorkel as the data programming framework to create large, annotated, multimodal datasets that can easily adapt to changing classification needs for training data-driven models.
• Integrating Label Studio for annotating multimodal data.
• Orchestrating the Labelling Function / Label Model / End Model development, testing, and monitoring using MLflow.
• Deploying all End Models using FastAPI.
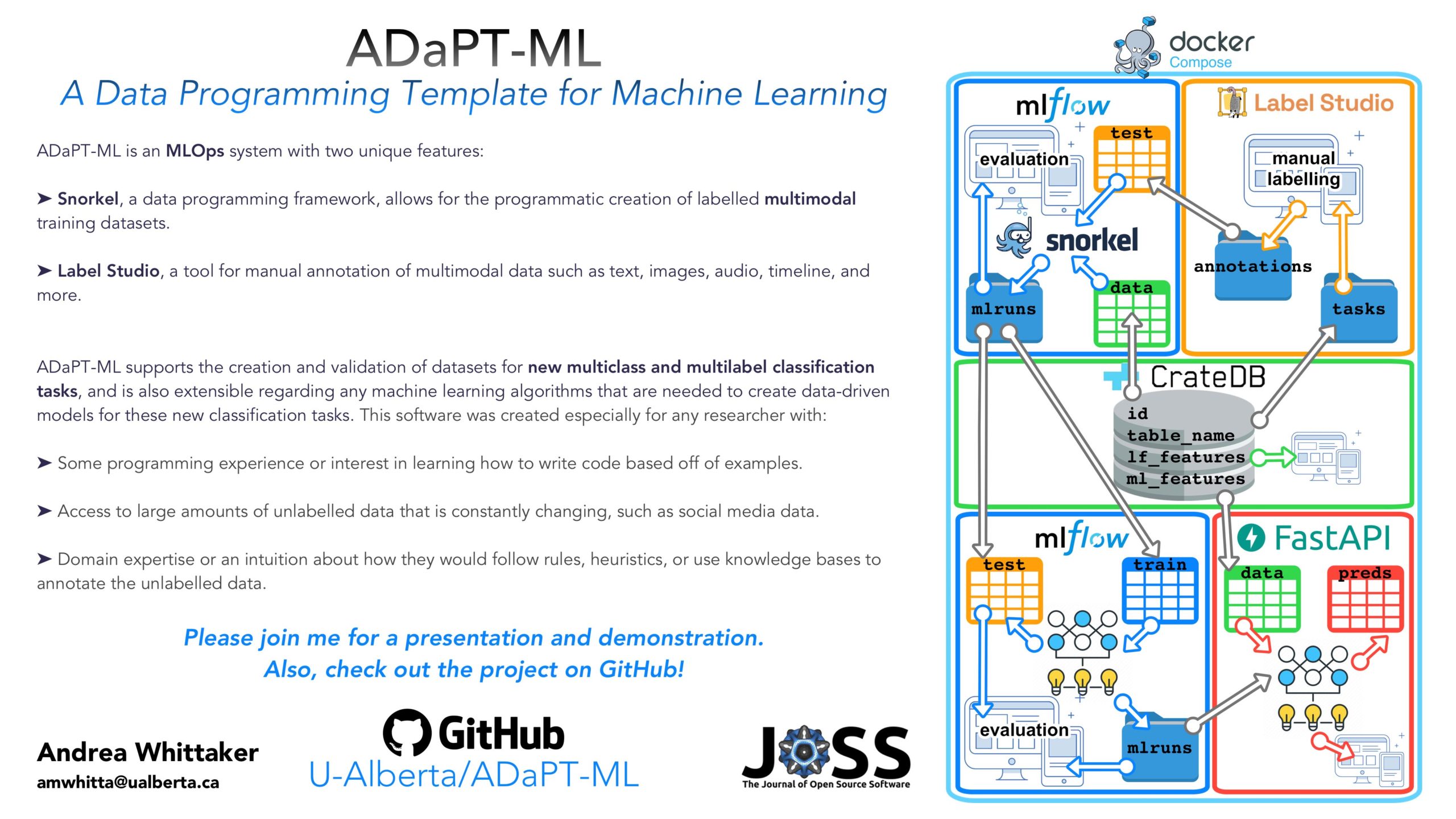
Next Steps for Voyant and Spyral
Researcher: Bennett Tchoh | Supervisor: Geoffrey Rockwell
With more and more text available as electronic text, there is a need for more efficient ways to get insight from these texts. Voyant-tools (https://voyant-tools.org/) is a web-based set of tools for text analysis and visualization. It is open source and can also be downloaded and installed on windows and mac platforms. Although Voyant-tools is popular and widely used, its use is limited to the options available in the user interface. To address this limitation, we are developing a web-based JavaScript notebook environment called Spyral (https://voyant-tools.org/spyral) that builds on Voyant-tools. With Spyral we can call the tools used in Voyant and it allows for more complex and customized analysis. We can also comment, save and share our analysis. Spyral is still in its testing phased. We are currently developing tutorial notebooks to introduce new users to Spyral. This also permits us to identify and correct issues like bugs and glitches. Voyant and Spyral are constantly being updated and we are looking at the possibilities to include more functionalities so developers, contributors and testers are welcomed..
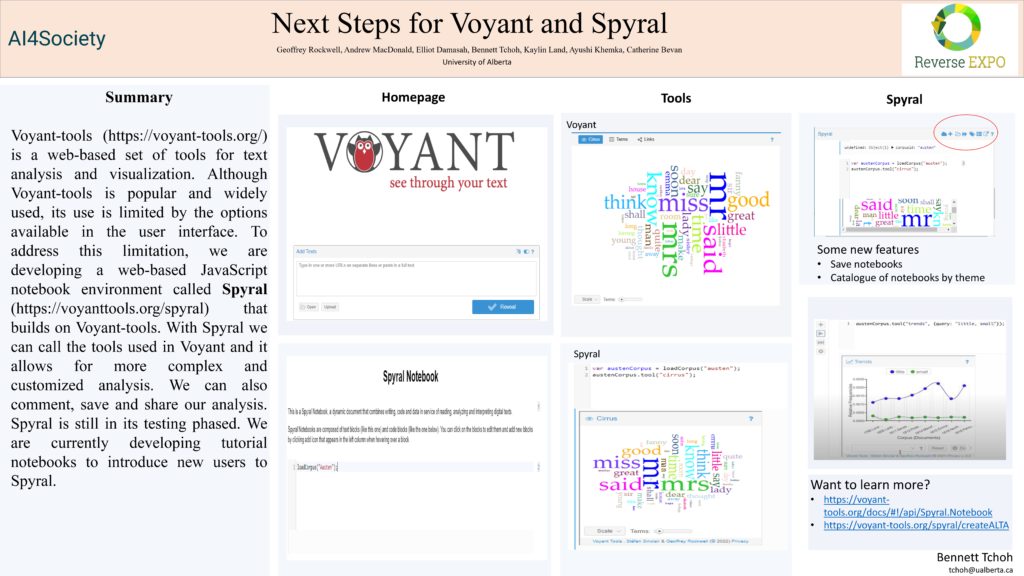
Analyzing and Visualizing Twitter Conversations
Researcher: Candelario A. Gutierrez Gutierrez | Supervisor: Eleni Stroulia
Social media platforms are public venues where users frequently participate in online conversations about topics of broad societal interest. For example, in Twitter they can interact in two ways: i) they can post an opinion or talk about an event, which is optionally accompanied with media, URL(s), hashtag(s) and/or handle(s); ii) they can like, share, reply and/or quote another user’s post. Private and public organizations have shown interest in this type of interactions to make event-driven decisions, and to achieve this, there is a necessity of Social Media Analytics (SMA) tools that facilitate the collection, processing, storage and extraction of insights from social media data. Hence, we present an automated and scalable data platform that contributes with three main components: a) an aspect based sentiment and a dictionary-based analysis within three lexicons, the personal values, sentiment, and humour; b) posts’ metadata and public’s engagement calculation; and c) feature rich visualizations to enable users to gain insights on their datasets. We demonstrate the usefulness of our platform with two case studies: (i) analyzing the fragmented narratives around established (hydro, oil and gas, coal, nuclear) and new (solar, wind, geothermal, biomass) energy sources; and (ii) comparing the social-media brands of academic institutions.
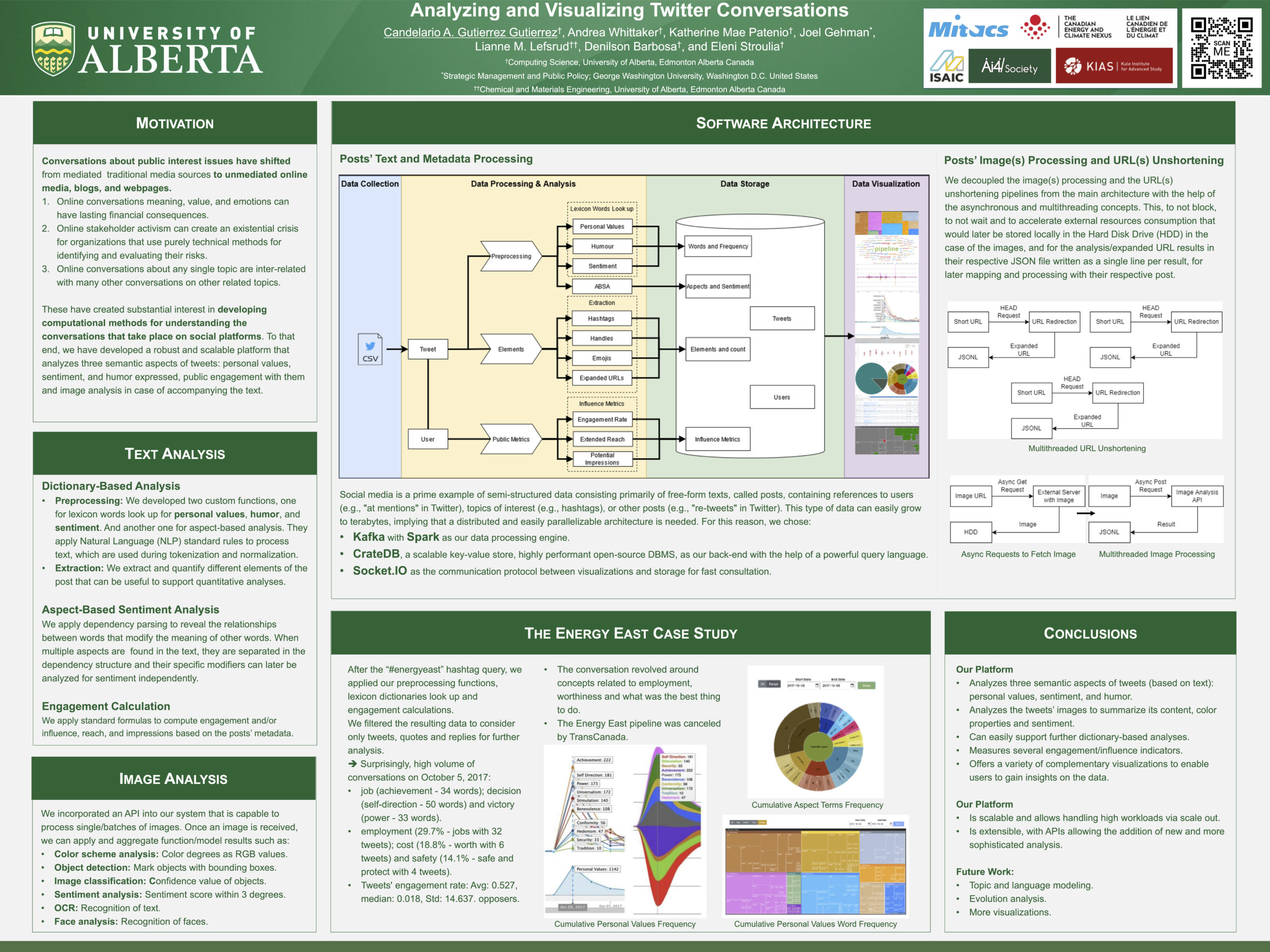
More at:
- Personal website: https://cande.me
- Project website: https://ai4buzz.ca
3D Human Behavior Modeling and Understanding
Researcher: Chuan Guo | Supervisor: Li Cheng
3D human behavior understanding and modeling aims to bridge the gap between 3D human behavior (e.g. motion, video) and other data domains such as action categories, control signals (e.g. directions, velocities, actions) and natural language, including topics of, for example, action recognition, motion captioning, or the inverse problems like human behavior simulation given actions, natural language descriptions or control signals.
In our project, we constructed large-scale datasets of annotated 3D human behaviors as well as developing a series of effective approaches to automatically understand, analyze and synthesize human behaviors in 3D environment.
Collaborative graph learning
Researcher: Fatemeh Tavakoli | Supervisor: Nidhi Hegde
Different organizations, companies, and even countries have invested a lot in creating or gathering valuable data for AI-developing purposes. Datasets owned by data owners may be biased, thus cannot generalize well over their interest population. For example, if we want to design drugs that are effective for all humankind, we need to share lab experiments on people of different nationalities. Or if we want to detect fraud, we need to have all the transactions in different financial institutions. For privacy and business reasons, it is not possible to share raw data. This research studies the problem of collaborative learning on graph-structured data. Consider many data owners that own a subset of a large graph. If we did not have any privacy concerns, the ideal case would be to connect these subgraphs according to their overlapping areas (shared nodes) to create a universal graph.
Our framework consists of a server and several data owners. Data owners encode their graphs into an embedding space using a GNN.
The goal of the server is to encourage the data owners’ models to encode their graphs into the same embedding space to reconstruct the universal graph in that embedding space and to enable collaborative training.
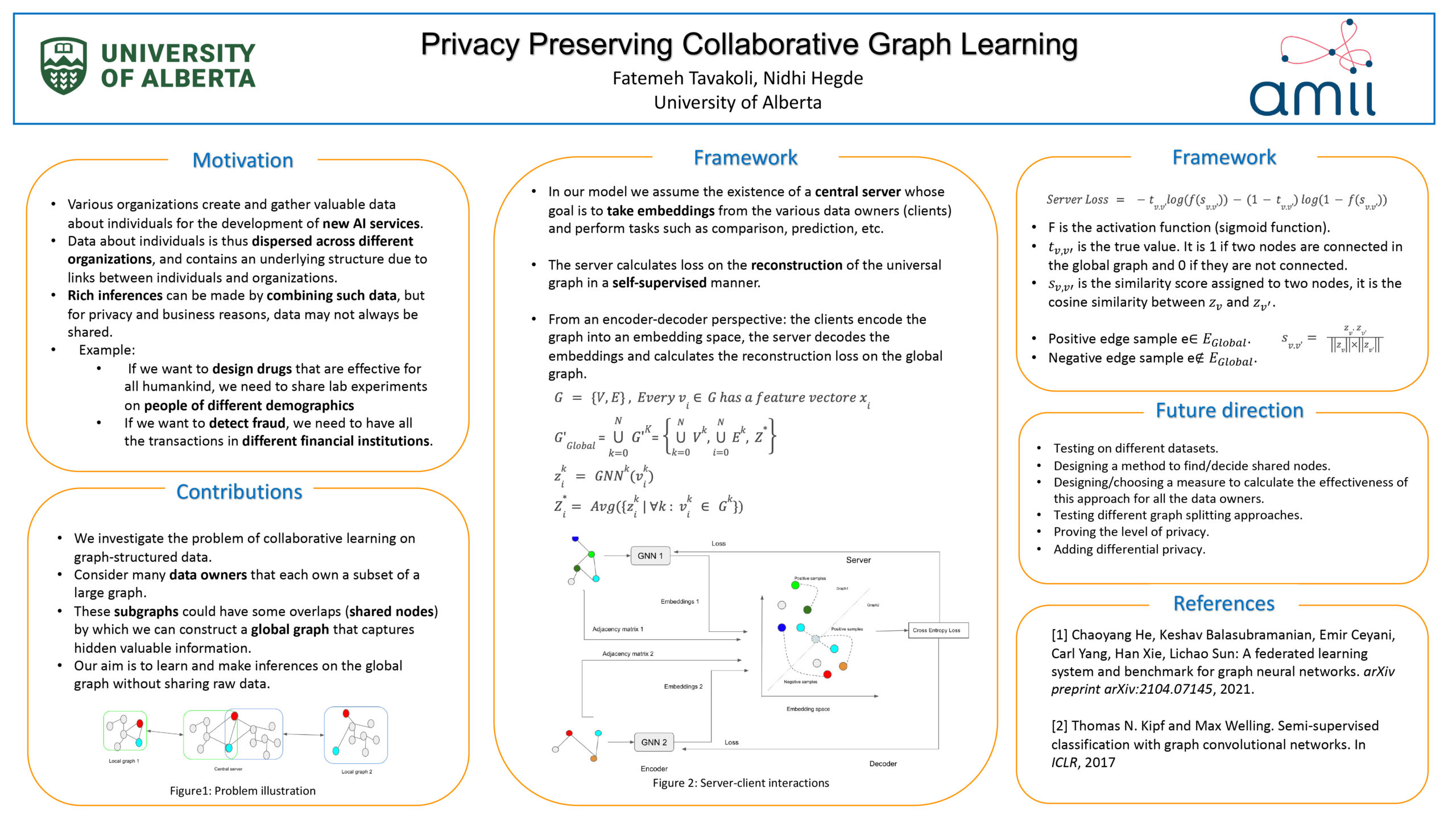
Personalized and Explainable Aspect-based Recommendation using Latent Opinion Groups
Researcher: Maryam Mirzaei | Supervisors: Joerg Sander, Eleni Stroulia
The problem of explainable recommendation—lsupporting the recommendation of a product or service with an explanation of why the item is a good choice for the user—is attracting substantial research attention recently. Recommendations associated with an explanation of how the aspects of the chosen item may meet the needs and preferences of the user can improve the transparency and trustworthiness of consumer-oriented applications, which is the motivation driving this research area.
Current methods are far from ideal because they do not necessarily consider the following issues: (i) users’ opinions are influenced not only by individual aspects but also by the dependency between sentiments towards aspect; (ii) not all users place the same value on all aspects; and, (iii) any explanation are not provided for how the item aspects have led to the recommendation. We introduce a personalized explainable aspect-based recommendation method that can address these challenges. To identify the aspects that a user cares about, our semantics-aware method learns the likelihood of an aspect being mentioned in a user’s review. To capture dependency between the users’ sentiments towards an aspect, reviews that express opinions with similar polarities towards sets of aspects are clustered together in latent opinion groups. To construct aspect-based explanations, item aspects are rated according to their importance based on these latent opinion groups and the preferences of the target user. Finally, to provide a user with a (set of) useful recommendation(s) of an item, our method selects and synthesizes the aspects important for the target user.
We evaluate our method over two datasets from (a) Yelp and (b) Tripadvisor. Our results demonstrate that our method outperforms previous methods in both recommendation performance and explainability.
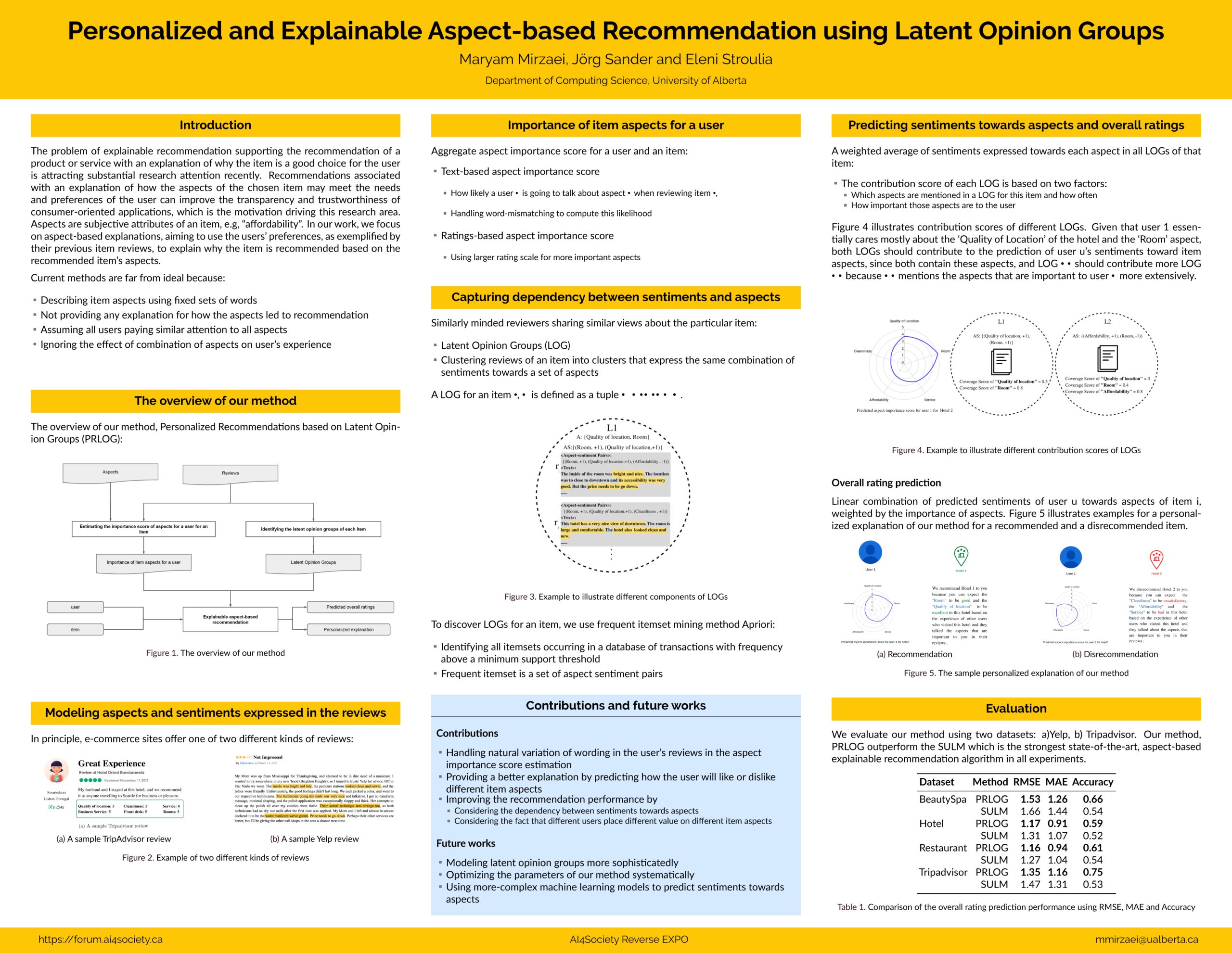
Collective Entity Linking with Quantum Annealing
Researcher: Peiran Yao | Supervisor: Denilson Barbosa
Entity linking is an important step towards the understanding of language, as it bridges natural language processing with knowledge graphs. When there are multiple entity mentions within a piece of text, collectively linking these entities by considering their intercompatibility will improve the linking accuracy. However, collective entity linking is a computationally hard problem as it requires solving a non-trivial combinatorial optimization problem. Recently, we have witnessed rapid developments, and even commercialization, of quantum annealing, which leverages quantum effects to physically provide approximate solutions to many combinatorial optimization problems in an efficient way. In this work, we propose a quantum annealing algorithm for collective entity linking based on the D-Wave quantum annealer. We show that the algorithm can provide comparable accuracy and speed to traditional approximation algorithms like simulated annealing. We also discuss certain limitations of deploying this algorithm in real-world applications.
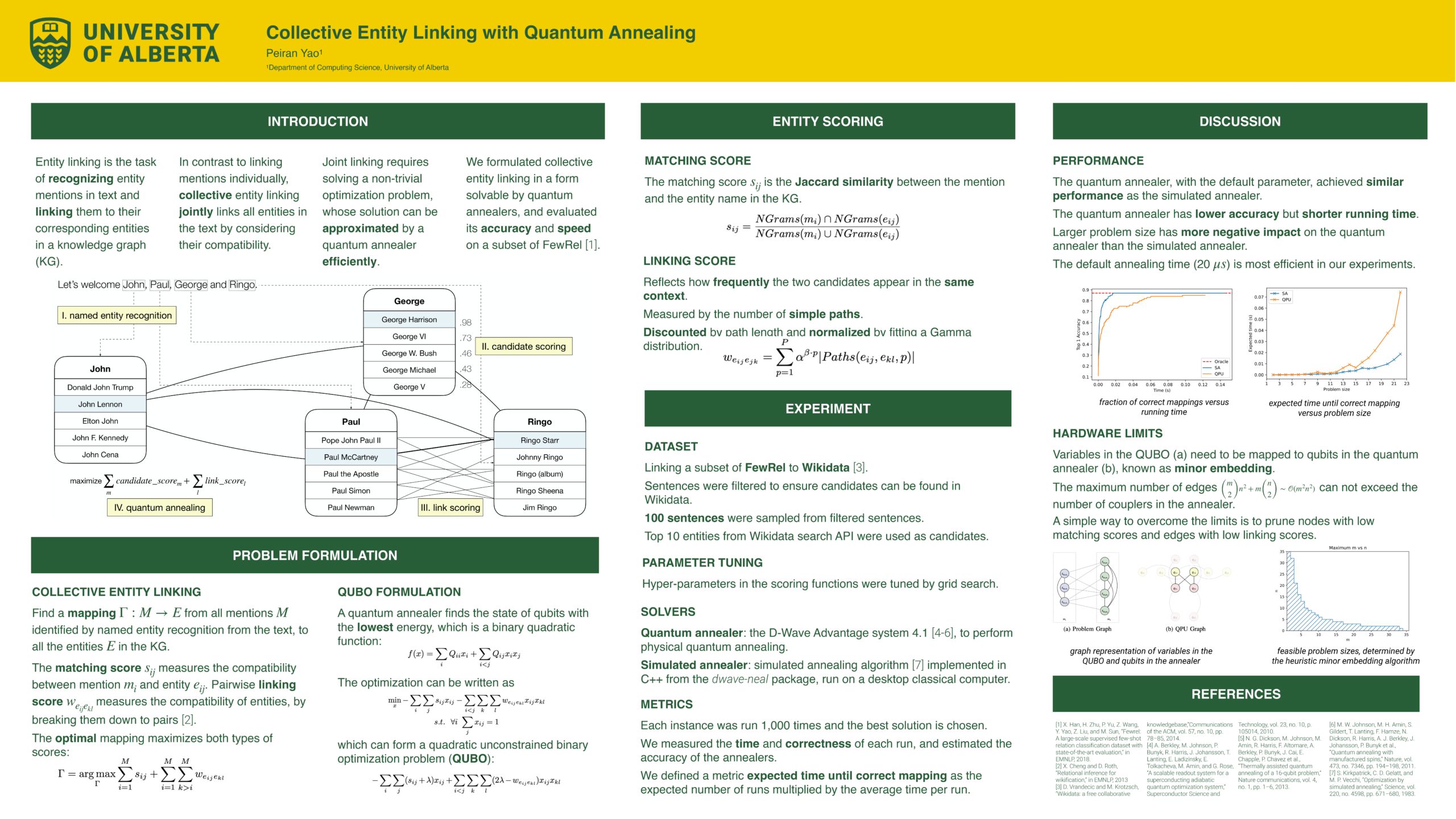
Cluster for Analysis of Relational Data (CARD): A new research cluster for equitable data analysis
Researcher: Pete Jones | Supervisor: Deb Verhoeven
The Cluster for Analysis of Relational Data (CARD) is a new research lab set up at UofA to bring together researchers working in the area of social network analysis and relational data science. The explicit goal of the lab is to pioneer research which combines the computational and data-driven tools developed in these related fields with the intersectional feminist ethics of the lab’s participants. Such research, which explores research questions from the humanities and social sciences using computational methods, is becoming increasingly impactful due to the rapid technological advances underpinning such methods, which have made previously intractable questions about people and their social worlds suddenly much more accessible. However, AI and the algorithms which structure our digital world continue to be biased by the entrenched power dynamics and inequalities that structure the societies that create and imagine them. In this poster, we introduce CARD by providing an overview of some of the challenges posed by these biased algorithms, and open a discussion regarding the kinds of solutions that might enable algorithms to be a tool for good in computational social research.
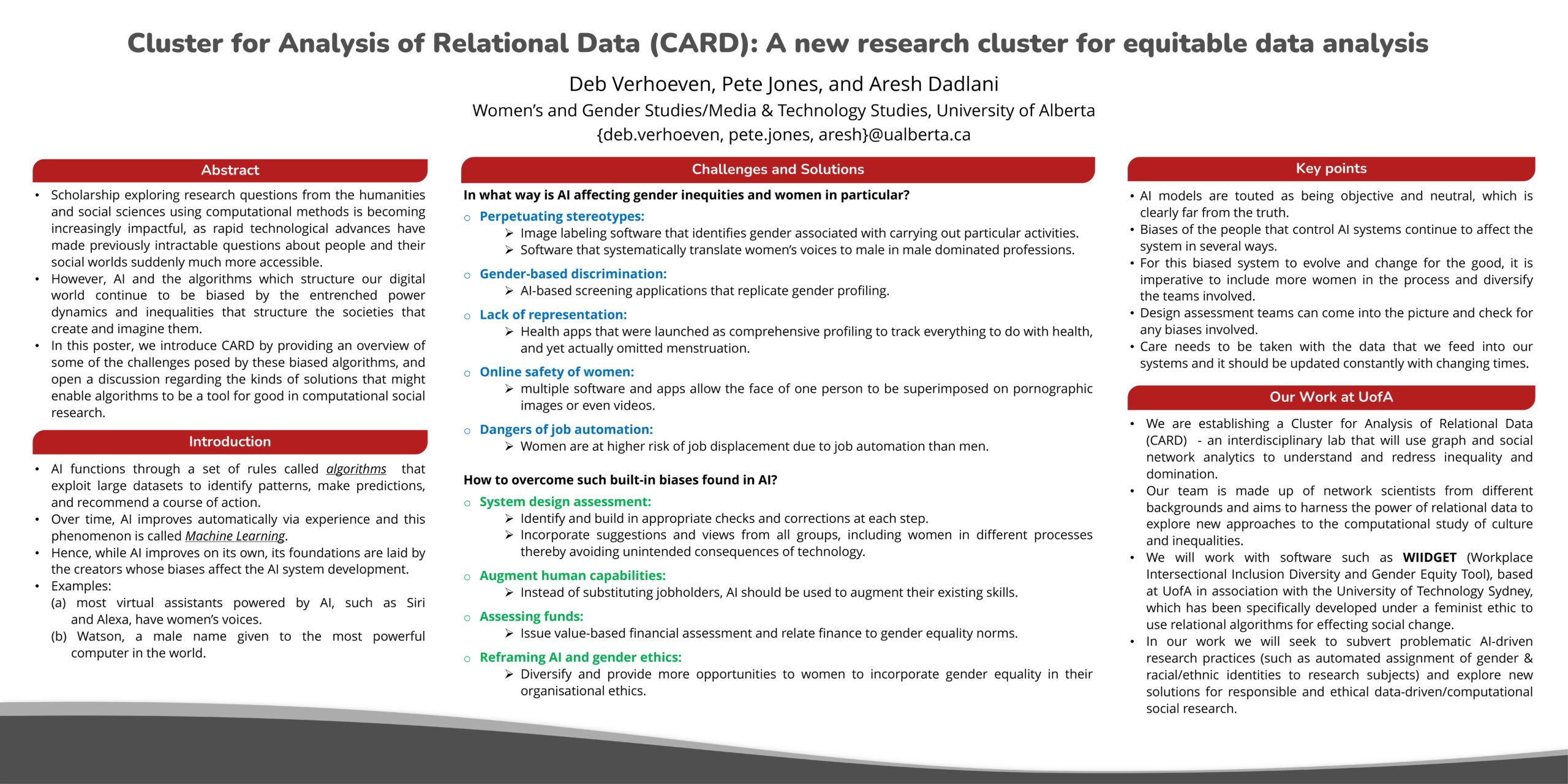
To Filter Prune, or to Layer Prune, That Is The Question
Researcher: Sara Elkerdawy | Supervisor: Nilanjan Ray
Recent advances in pruning of neural networks have made it possible to remove a large number of filters or weights without any perceptible drop in accuracy. The number of parameters and that of FLOPs are usually the reported metrics to measure the quality of the pruned models. However, the gain in speed for these pruned models is often overlooked in the literature due to the complex nature of latency measurements. In this paper, we show the limitation of filter pruning methods in terms of latency reduction and propose LayerPrune framework. Layer- Prune presents a set of layer pruning methods based on different criteria that achieve higher latency reduction than filter pruning methods on similar accuracy. The advantage of layer pruning over filter pruning in terms of latency reduction is a result of the fact that the former is not constrained by the original model’s depth and thus allows for a larger range of latency reduction. For each filter pruning method we examined, we use the same filter importance criterion to calculate a per-layer importance score in one-shot. We then prune the least important layers and fine-tune the shallower model which obtains comparable or better accuracy than its filter-based pruning counterpart. This one-shot process allows to remove layers from single path networks like VGG before fine- tuning, unlike in iterative filter pruning, a minimum number of filters per layer is required to allow for data flow which constraint the search space. To the best of our knowledge, we are the first to examine the ef-fect of pruning methods on latency metric instead of FLOPs for multiple networks, datasets and hardware targets. LayerPrune also outperforms handcrafted architectures such as Shufflenet, MobileNet, MNASNet and ResNet18 by 7.3%, 4.6%, 2.8% and 0.5% respectively on similar latency budget on ImageNet dataset.
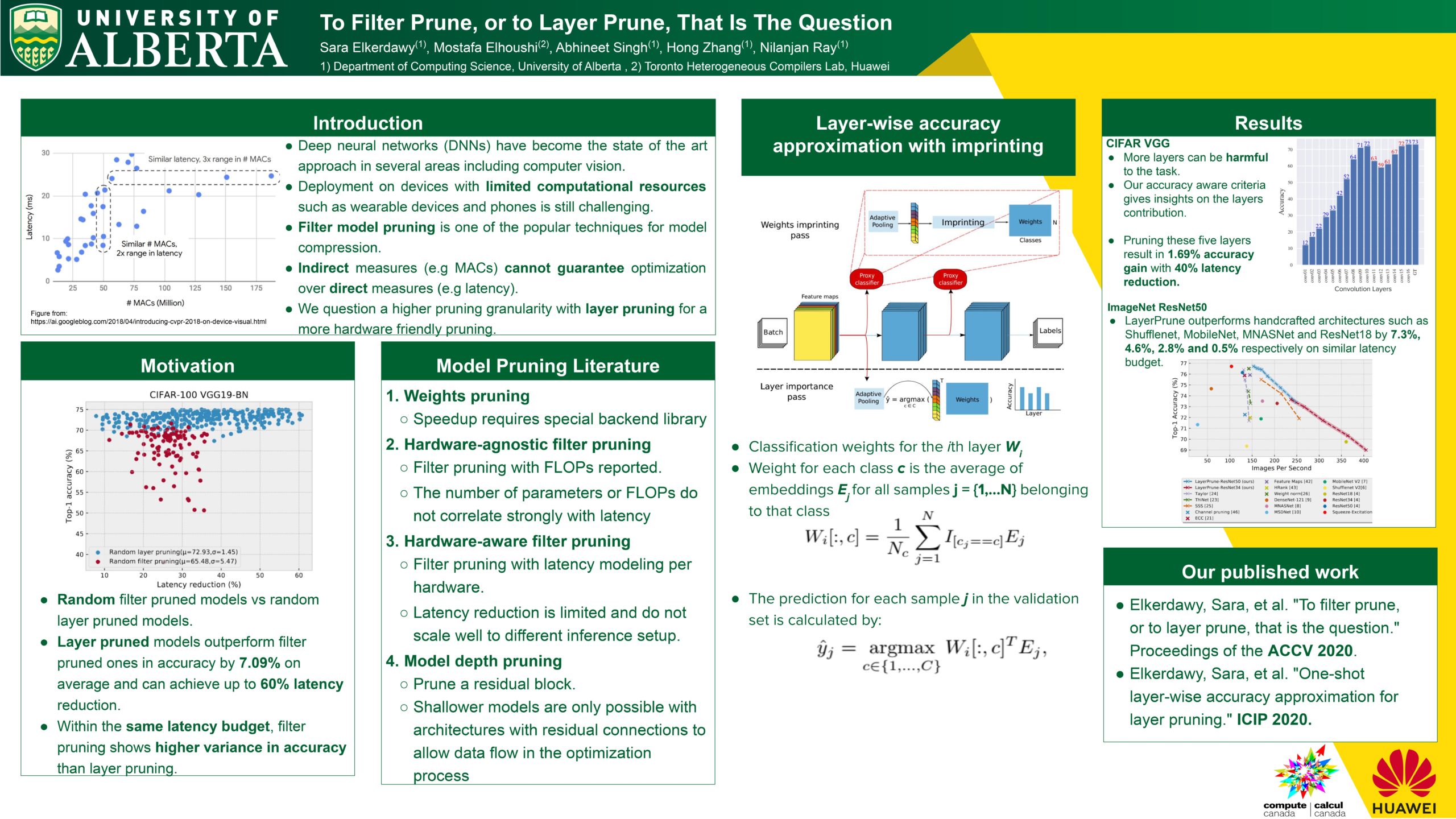
Online Search Under Convex Costs
Researcher: Siyuan Yu | Supervisor: Xiaoqi Tan
We propose online search under convex costs (OSCC), a new variant of online optimization problems that is fundamental to many applications such as electric vehicle charging, Internet advertising, financial trading, and various artificial intelligence and machine learning problems. In OSCC, agents arrive one at a time (e.g., sequential arrival of electric vehicles in a charging station), each with a value that remains unknown before its arrival. At each step when there is a new arrival, an irrevocable decision must be made in a “take-it-or-leave-it” manner, namely, to accept the agent and take its value, or reject it with no value. The crux of OSCC is that we must pay for an increasing and convex cost associated with the number of accepted agents – it is increasingly more difficult to accommodate additional agents. We are interested in social welfare maximization, namely, the sum of the accepted agents’ values minus the total convex cost.
We derive an adaptive threshold policy (ATP) that accepts an agent if its value exceeds an adaptively-changing threshold. Our ATP is logistically simple and intuitive to implement, and achieves the best-possible competitive ratio of all deterministic online algorithms. A key ingredient in our design is to connect the threshold function to the dual of the underlying offline optimization problem via the online primal-dual framework. We also give a lower bound on the competitive ratios of randomized algorithms, and prove that the competitive ratio of our ATP asymptotically converges to this lower bound when the number of agents that can be accommodated is sufficiently large.
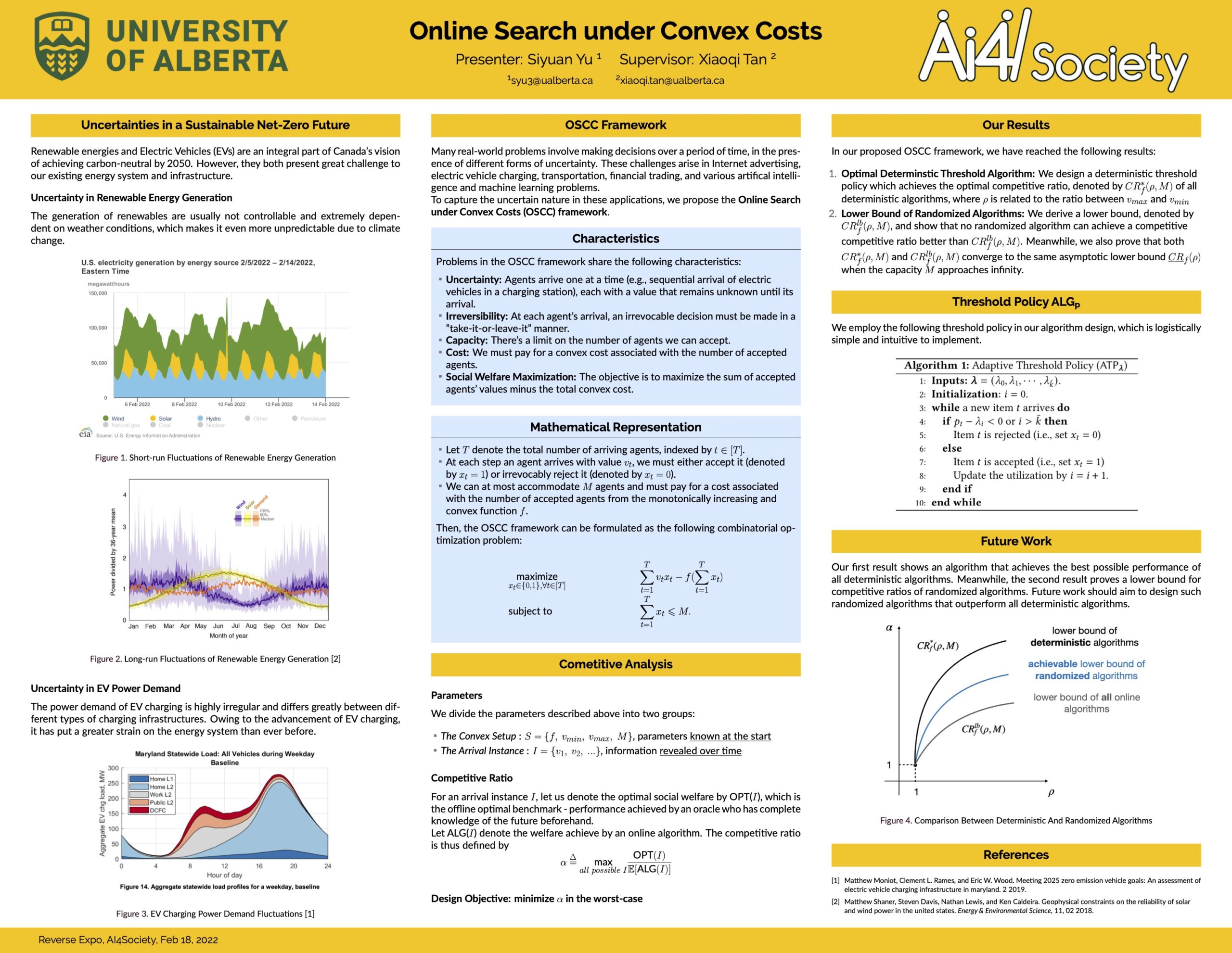
Generative Models Based on VAE and GAN for Data Synthesis
Researcher: Yuyang Nie | Supervisor: Anup Basu
In almost all supervised learning methods, the model’s quality depends on the input data. However, many research fields lack large-scale and diverse data sets. Image data collection and labelling can be challenging and costly in various tasks such as camera-capturing rare birds, labelling rare birds that requires domain expertise. Generative models like Generative Adversarial Networks (GAN) fill this gap by generating the data set needed by these models, and hence have a significant role in future AI applications.
In our work, we developed a generative model using Variational Autoencoder (VAE) and GAN to synthesize new image data. We have implemented a VAE model and used it as the generator for GAN and train the model on the “Caltech-UCSD Birds 200” dataset. The novelty about our work is that we added an extra component in the model to incorporate bird types. As a result, the model can not only generate more diverse images within the same category, but also counter-factual image data (e.g. sparrow-looking crow).
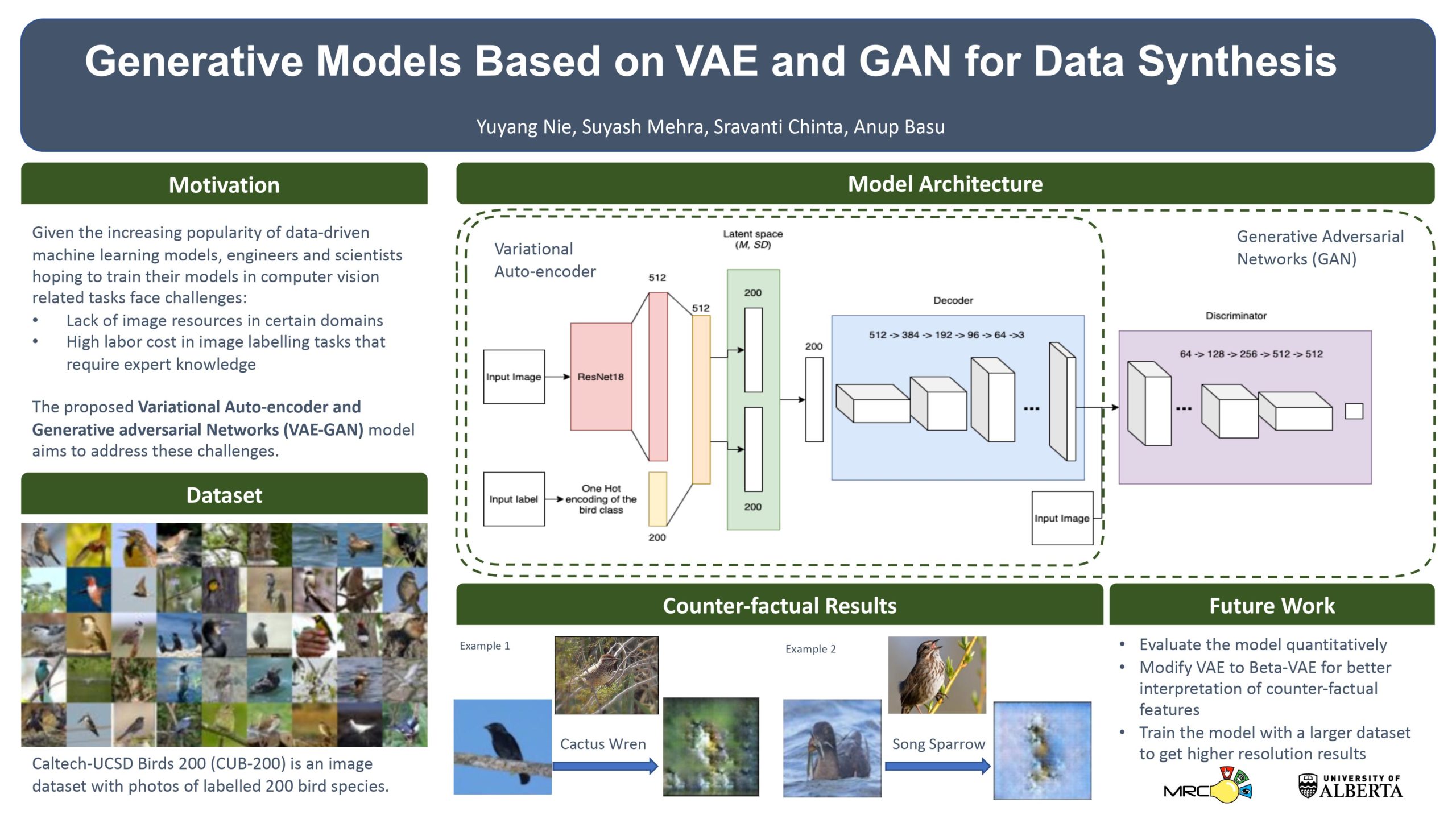
Generating Private Time Series Dataset
Researcher: Zahra Bashir | Supervisor: Nidhi Hegde
Some datasets contain sensitive private information that should not be shared publicly. The necessity of having a synthetic dataset that is so close to the original one but not leaking any private information appears here. I’m working on private data generation using Time-GANs for time series. Time-GAN is a natural framework for generating realistic time-series data in different domains. The model contains an Embedder, a Recovery, a Generator, and a Discriminator. For instance, I’m trying to generate an activities dataset(for one subject) in which we cannot tell that person A did X at time T. This is what I define as a private synthetic dataset. Differential Privacy(DP) and Pufferfish Privacy(PP) happen to be some concepts I’m interested in. I’m trying to preserve Time-GAN using DP and PP in terms of privacy. I’ll apply DP during the Time-GAN training to add randomness to the gradients(DPSGD), leading to a more private model that cannot be attacked(for instance, inference attack). Also, I’ll apply a mechanism named Markov Quilt(in the PP category) to the synthetic data directly to make the data private. I’m going to compare these two approaches to see which is more helpful in terms of fidelity, privacy, and utility.
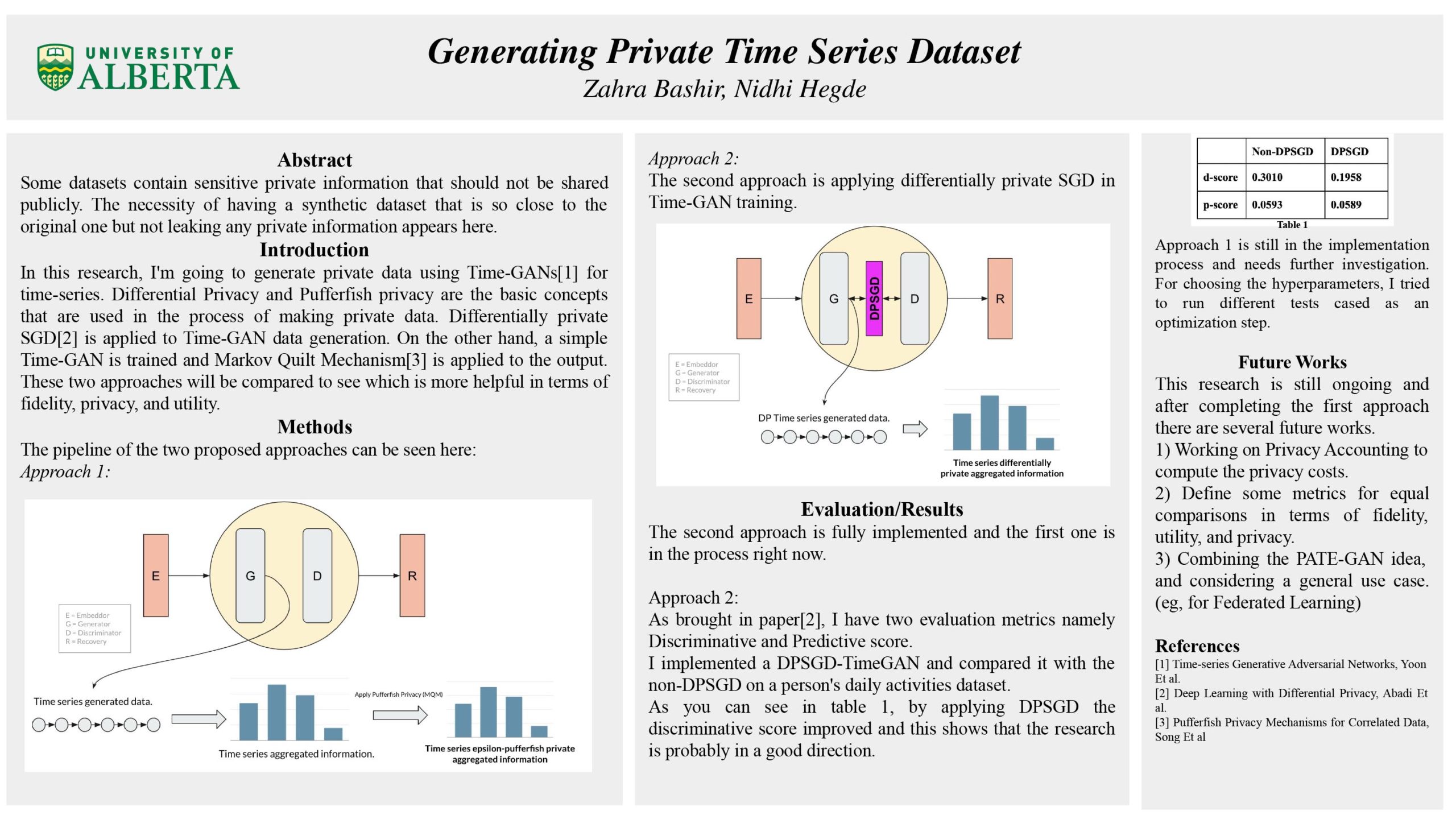
AI and Social Media Identity
Project description:
Supervisor:
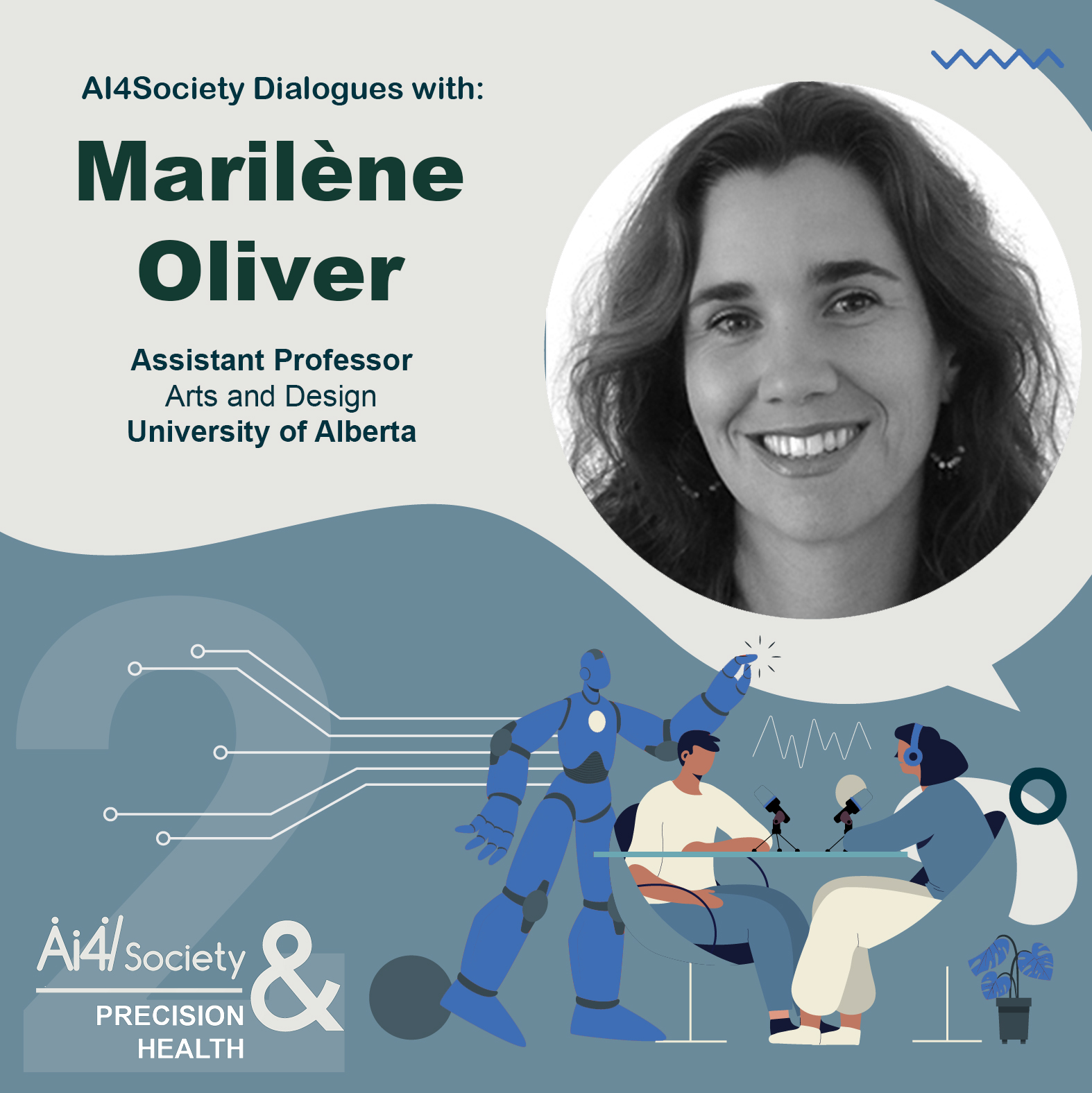
Description:
Supervisor:
Description:
Developing games is challenging as it requires expertise in programming skills and game design. This process is costly and consumes a great deal of time. In this paper we describe a tool we developed to help decrease this burden: Mechanic Maker. Mechanic Maker is designed to allow users to make 2D games without programming. A user interacts with a machine learning AI agent, which learns game mechanics by demonstration.
sdafsdf
Researcher: Vardan Saini
Supervisor: Matthew Guzdial
Developing games is challenging as it requires expertise in programming skills and game design. This process is costly and consumes a great deal of time. In this paper we describe a tool we developed to help decrease this burden: Mechanic Maker. Mechanic Maker is designed to allow users to make 2D games without programming. A user interacts with a machine learning AI agent, which learns game mechanics by demonstration.
Health Sciences
- Health Sciences
- Fei Wang
- Jasmine Noble
- Jeff Sawalha
- Melika Torabgar
- Ramon Diaz-Ramos
- Reed Sutton
- Shaista Meghani
- Shamanth Shankarnarayan
- Victor Fernandez Cervantes
- Yuyue Zhou
Health Sciences
10 projects related to General Health, Psychiatry, Pathogens, and more!
Click on the tabs in the left to access the material of each presenter
NPS-MS: A Highly Accurate MS/MS Prediction Model for Novel Psychoactive Substances
Researcher: Fei Wang | Supervisor: Russell Greiner, David Wishart
New psychoactive substances (NPS), also referred to as designer drugs, ‘legal highs’, or bath salts, have emerged as significant public health and drug control issues worldwide. These molecules possess the same psychoactive effects as conventional illicit drugs but with sufficiently different chemical structures that circumvent existing drug control legislation. Mass spectrometry, particularly tandem mass spectrometry (MS/MS), is the primary analytical technique used to screen for NPS in seizures or biological samples. However, it is challenging to match experimentally collected mass spectra to the chemical structures of these NPS due to the lack of reference mass spectra for NPS compounds. This work describes a learned model that can accurately predict ESI-QToF-MS/MS from NPS compound structure. Our model is trained via transfer learning from a pre-trained CFM-ID 4.0 model. The spectra predicted by that resulting NPS-MS are 77% and 69% better than CFM-ID 4.0 on average when measured by Dice and DotProduct, respectively. We also show that this improved performance enables more accurate NPS compound identification than existing tools for MS/MS spectrum interpretation.
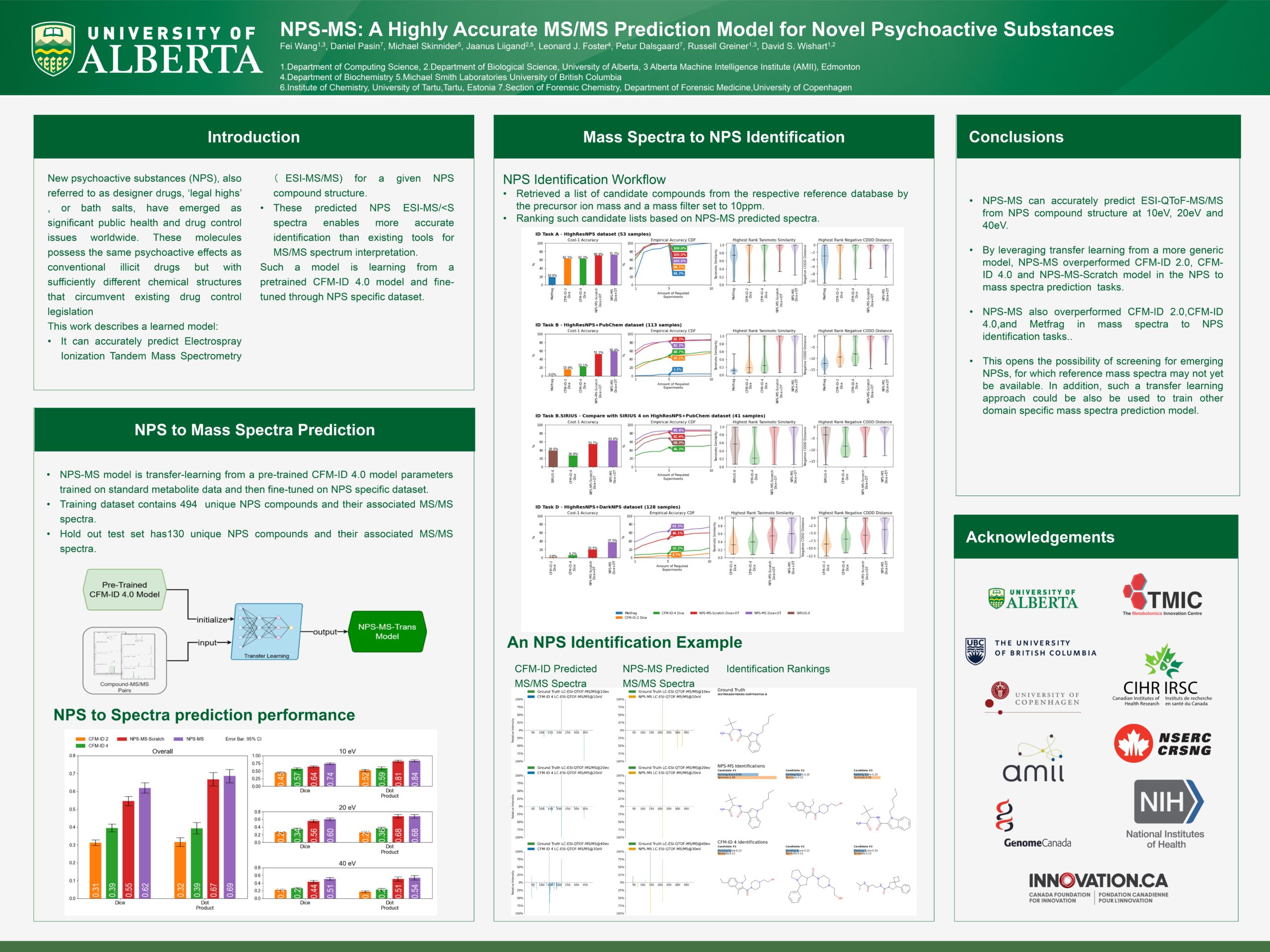
Developing and Implementing a Machine Intelligence Mental Health System Navigation Chatbot to Support Healthcare Workers in Two Canadian Provinces (Project Protocol)
Researchers: Jasmine Noble, Ali Zamani, Mohamad Ali Gharaat, Isabella Nikolaidis
Supervisors: Osmar Zaiane, Eleni Stroulia, Andrew Greenshaw
Background: One in three Canadians will experience an addiction and/or mental health challenge at some point in their lifetime. There are multiple barriers in accessing mental health care including stigma, system fragmentation, episodic care, long wait times, and insufficient supports for health system navigation. Digital technologies present opportunities to bridge gaps in mental health care. Chatbots may be explored to support those in need of information and/or access to services, and present the opportunity to address gaps in care, on demand, with personalized attention.
Objective: This pilot study seeks to evaluate the feasibility and effectiveness of a mental health system navigation machine intelligence chatbot (MIRA, the Mental Health Virtual Assistant).
Methods: Participants will be healthcare workers and their families located in Alberta and Nova Scotia. The effectiveness of the technology will be assessed through the analysis of voluntary follow-up surveys, and client engagement with the chatbot.
Results: This project was initiated April 1st, 2021. Data collection is anticipated to begin March 1st, 2022 to March 31st, 2023. Publication of a final report will be developed by April 1st, 2023.
Conclusions: Our findings can be incorporated into public policy and planning around mental health system navigation by any/all Canadian mental health care providers.
Using Machine Learning to Distinguish Dementia in Older Adults Based on Engagement-Related Behaviours While Playing Video Games
Researcher: Melika Torabgar | Supervisor: Adriana Rios Rincon
Dementia affects the behavioural, psychological, and social dimensions of the lives of older adults who live with the disease. Dementia may lead to behavioural changes. Older adults with dementia may demonstrate engagement through behaviours that differ from those of cognitively intact older adults. The objective of this project is to use machine learning techniques to test the ability of the dataset of engagement-related behaviours demonstrated by older adults while playing mobile games to distinguish persons with and without dementia. The relevance of this project relies on the fact that machine learning can potentially help rehabilitation professionals detect cognitive decline and dementia in older adults. Using alternative means such as playing video games may be more interesting or engaging than the application of standardized screening tools.
Project aim The aim of this project is to use artificial intelligence, especially supervised machine learning, to distinguish dementia based on older adults’ engagement-related behaviours while playing a video game. This project could be a useful option to help rehabilitation professionals to distinguish clients who are experiencing dementia based on their engagement-related behaviours while performing leisure activities.
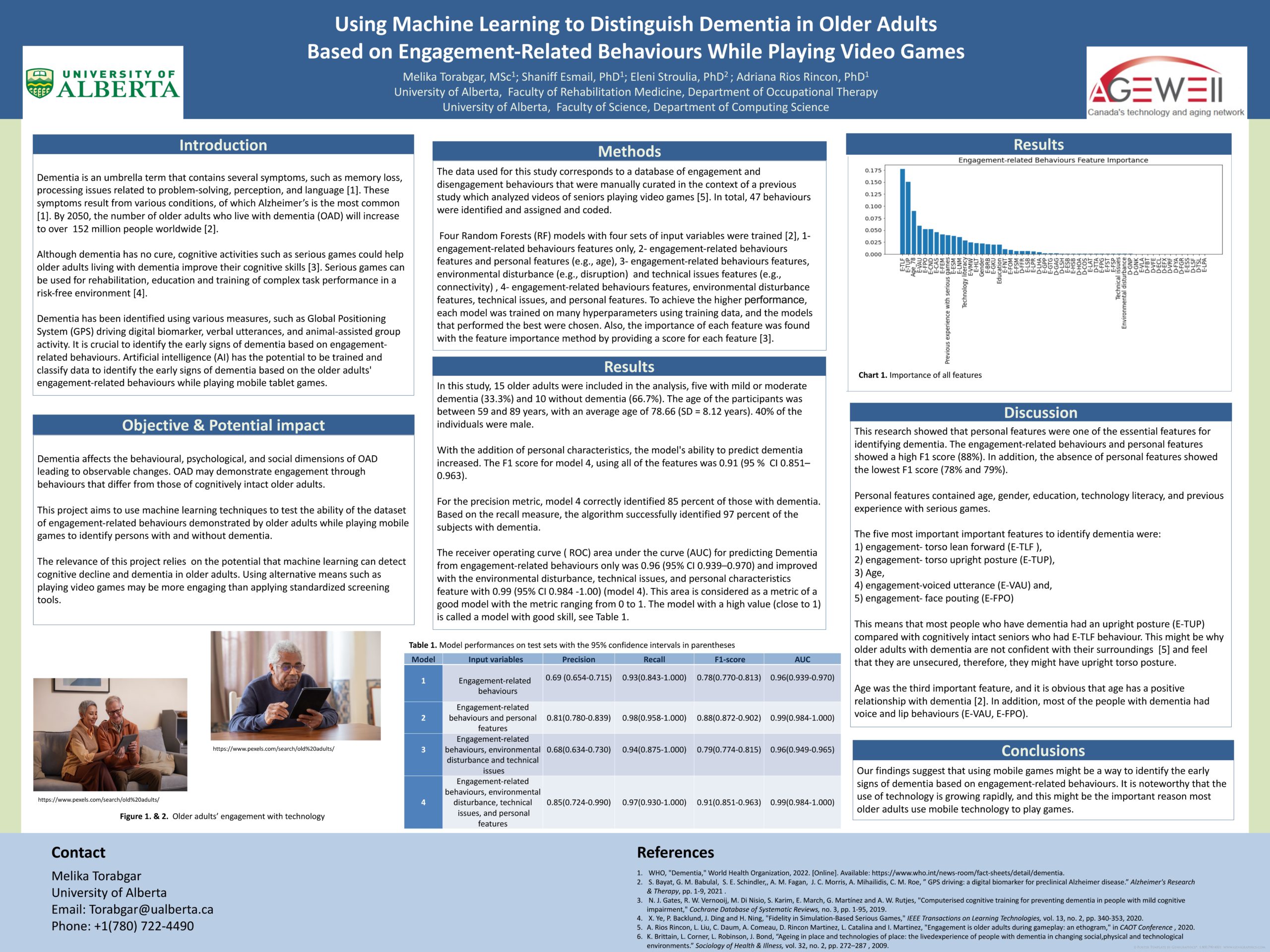
Physiological and Behavioural Data Collection to Predict Anxiety, Stress, and Depression Among Students
Researcher: Ramon Diaz-Ramos | Supervisor: Eleni Stroulia
Mental health is a severe concern to Universities around the world. University students have an increased risk of developing mental health problems due to the challenging conditions of academic pressure, financial burden, and changes in lifestyle habits [1]. Our focus is on stress, anxiety, and depression, which have been constantly correlated among university students to impair academic performance [2, 3, 4]. And more recently, the COVID pandemic has exacerbated this phenomenon. Due to recent events of the world pandemic of COVID-19, governments have implemented social distancing and other health measures that have changed the daily habits of university students, making them susceptible to mental health issues.The necessity of having technological resources for selfassessment and access to wellness practices becomes evident.
In our work, we are interested in developing a practical system that can collect physiological variables that are captured with embedded sensors of smartwatches (e.g., heart rate, body temperature, oxygen levels) and behavior data that are obtained with voice and text and analyze them to detect mood (stress, anxiety, and depression), in relation to the mental health of adult students. A prerequisite for the envisioned system is a model capable of detecting evidence of depression, anxiety, and stress from the mentioned data.
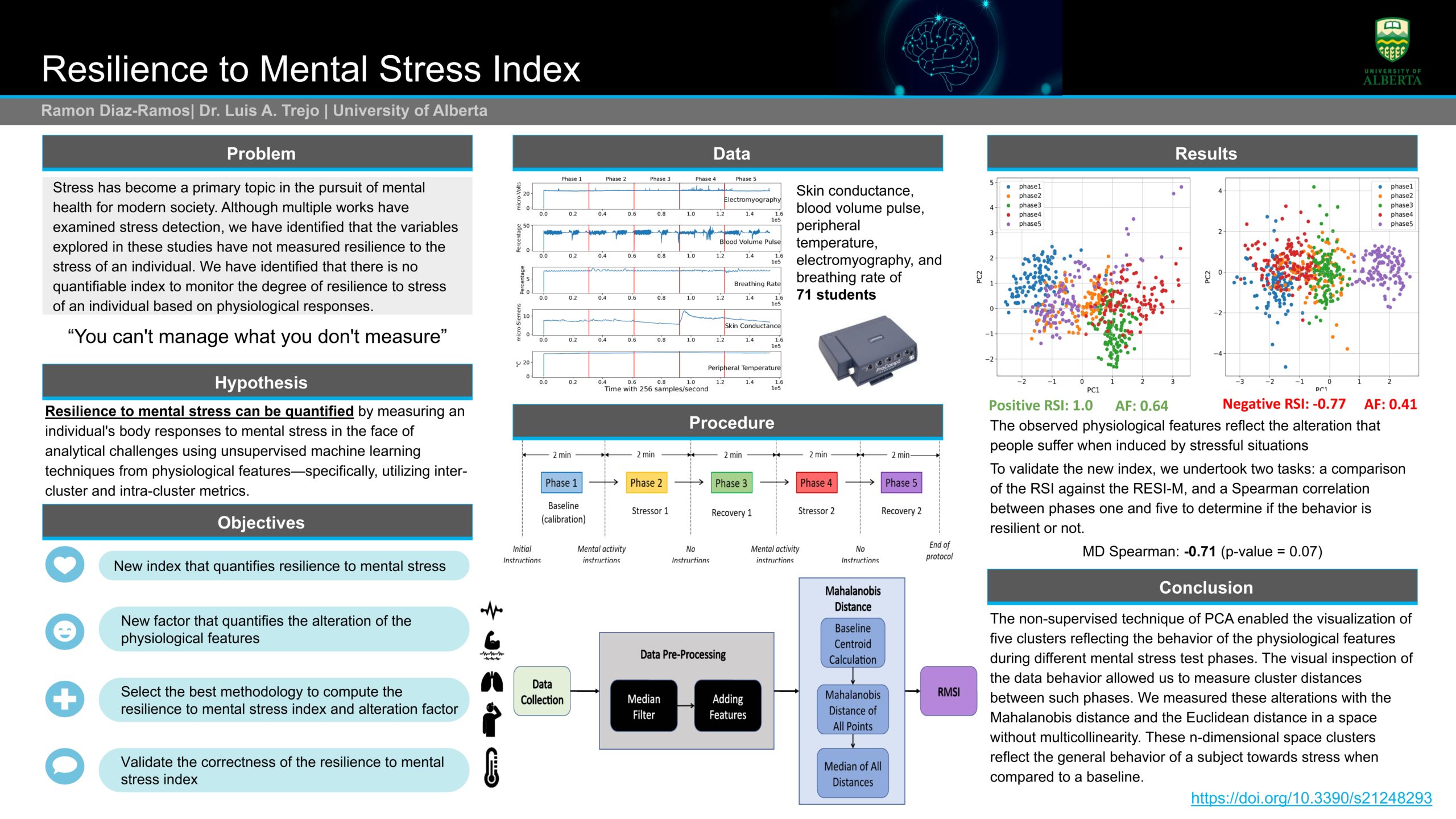
Artificial intelligence enabled automated diagnosis and grading of ulcerative colitis endoscopy images
Researcher: Reed Sutton | Supervisors: Daniel C. Baumgart, Randolph Goebel, Osmar R. Zaı̈ane
Endoscopic evaluation to reliably grade disease activity, detect complications including cancer and verification of mucosal healing are paramount in the care of patients with ulcerative colitis (UC); but this evaluation is hampered by substantial intra- and inter-observer variability. Recently, artificial intelligence methodologies have been proposed to facilitate more objective, reproducible endoscopic assessment. We compared how well several deep learning convolutional neural network architectures (CNNs) applied to a diverse subset of 8000 labeled endoscopic still images derived from HyperKvasir, the largest multi-class image and video dataset from the gastrointestinal tract available today. The HyperKvasir dataset includes 110,079 images and 374 videos and could (1) accurately distinguish UC from non-UC pathologies, and (2) inform the Mayo score of endoscopic disease severity. We grouped 851 UC images labeled with a Mayo score of 0–3, into an inactive/mild (236) and moderate/severe (604) dichotomy. Weights were initialized with ImageNet, and Grid Search was used to identify the best hyperparameters using fivefold cross-validation. The best accuracy (87.50%) and Area Under the Curve (AUC) (0.90) was achieved using the DenseNet121 architecture. Finally, we used Gradient-weighted Class Activation Maps (Grad-CAM) to improve visual interpretation of the model and take an explainable artificial intelligence approach (XAI).
Machine learning for the rapid detection of drug-resistant fungal pathogens
Researcher: Shamanth A Shankarnarayan | Supervisors: Randy Goebel, Jay Newby, Daniel Charlebois
Antimicrobial resistance is an emerging global health crisis that is undermining the advances in modern medicine. Systemic fungal infections among hospitalized patients are increasing and mortality rates exceeding 70% have been recorded [PMID: 29749387]. The limited number antifungal drugs available for treatment, along with the emergence of antifungal drug resistance, greatly restricts therapeutic options. Moreover, the emergence of multidrug-resistant fungal pathogens such as Candida auris are increasingly of concern [PMID: 28888662]. Early identification of infecting pathogens and their resistance profiles will enable prompt antimicrobial therapy, leading to better outcomes for patients. Machine learning methods are extensively used in medical diagnostics [PMID: 15333167]. In our research, we are applying machine learning to rapidly identify fungal pathogens. We are using high-resolution images of different fungal species to train machine learning classifiers, including support vector machines and convoluted neural networks. These high-resolution images are preprocessed to greyscale images and rescaled to keep the aspect ratios constant. The resulting image datasets are then divided into training and testing sets to train and evaluate the classifiers. Finally, Keras (a deep learning API written in Python) together with the TensorFlow machine learning platform and Scikit-learn modules are being used to classify the images.
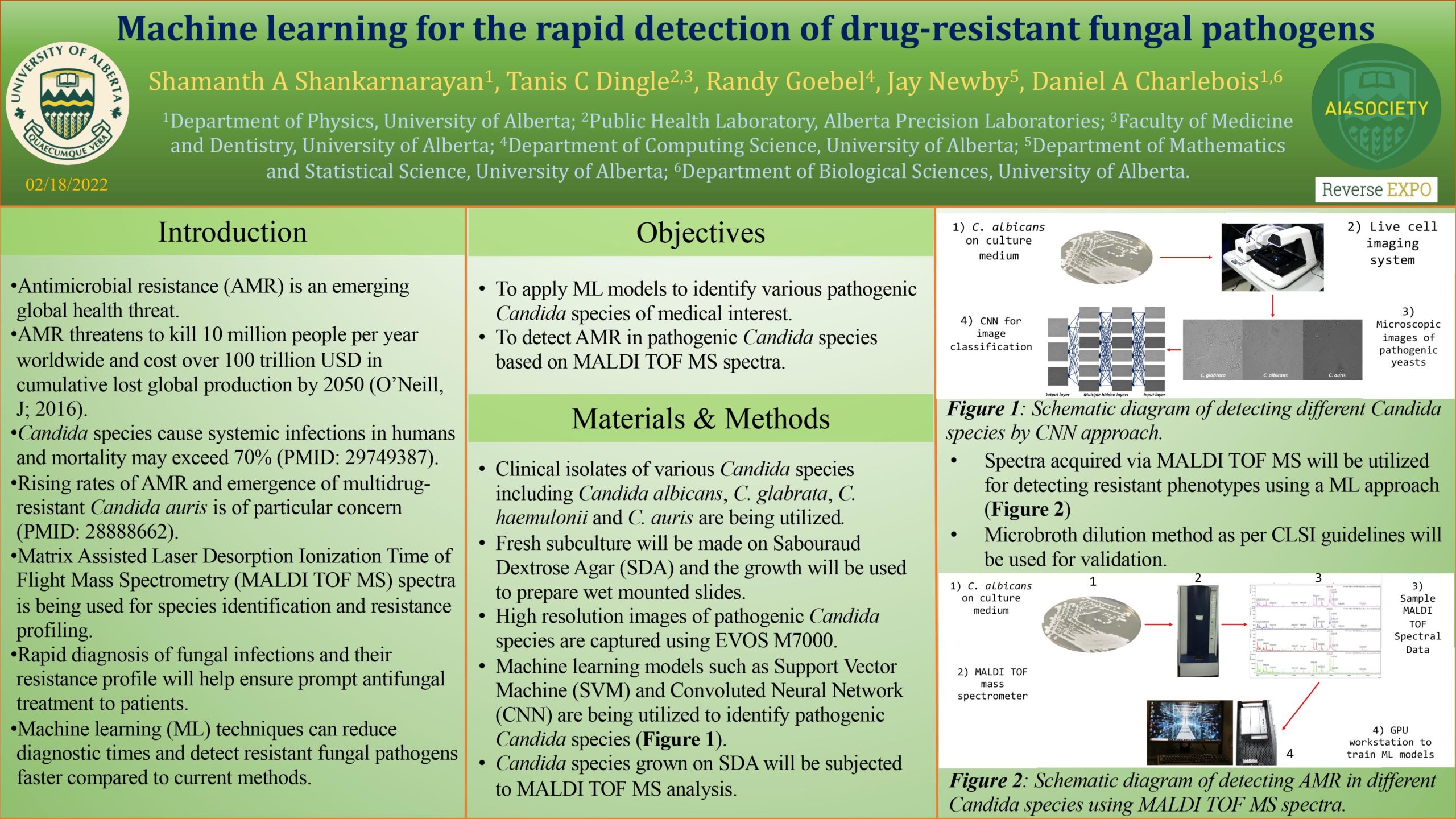
Virtual GymVR: Serious Exergame Platform for Personalized Physical and Cognitive Activities
Researcher: Victor Fernandez Cervantes | Supervisor: Eleni Stroulia
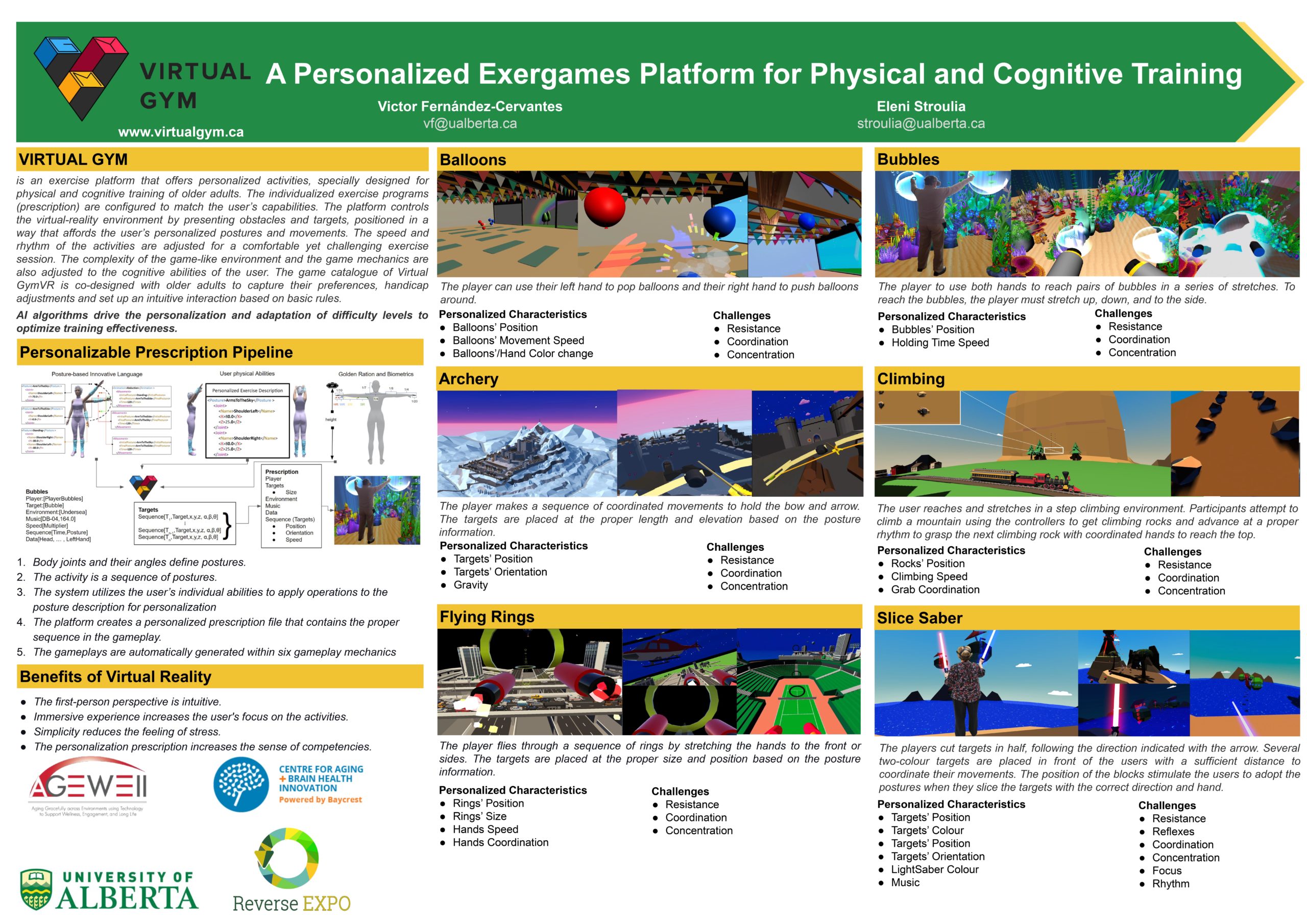
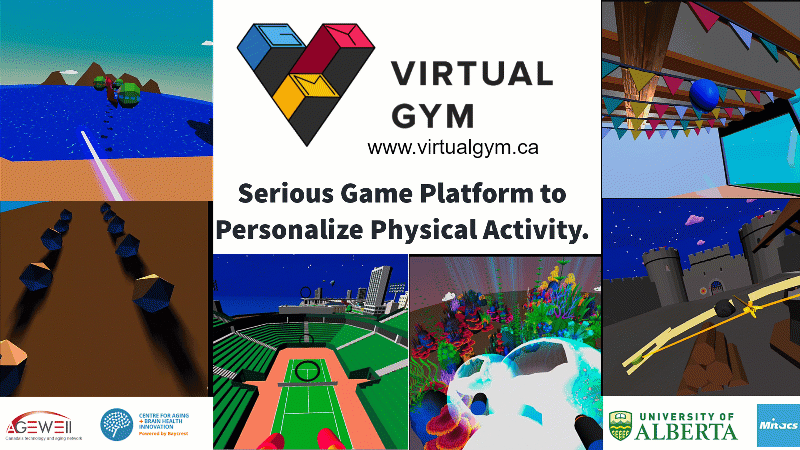
Wrist Ultrasound Segmentation by Deep Learning
Researcher: Yuyue Zhou | Supervisor: Jacob L. Jaremko
Ultrasound (US) is an increasingly popular medical imaging modality in clinical practice. It is ideally suited for wrist and elbow fracture detection in children as it does not involve any ionizing radiation. Automatic assessment of wrist images requires delineation of relevant bony structures seen in the image including the radial epiphysis, radial metaphysis and carpal bones. With the advent of artificial intelligence, researchers are using deep learning models for segmentation in US scans to help with automatic diagnosis and disease progression. In this research, we applied deep learning models including UNet and Generative Adversarial Network(GAN) to segment bony structures from a wrist US scan. Our ensemble models were trained on wrist 3D US datasets containing 10,500 images in 47 patients acquired from the University of Alberta Hospital (UAH) pediatric emergency department using a Philips iU22 ultrasound scanner. In general, although UNet gave the highest DICE score, precision and Jaccard Index, GAN achieved the highest recall. Our study shows the feasibility of using deep learning techniques for automatically segmenting bony regions from a wrist US image which could lead to automatic detection of fractures in pediatric emergencies.
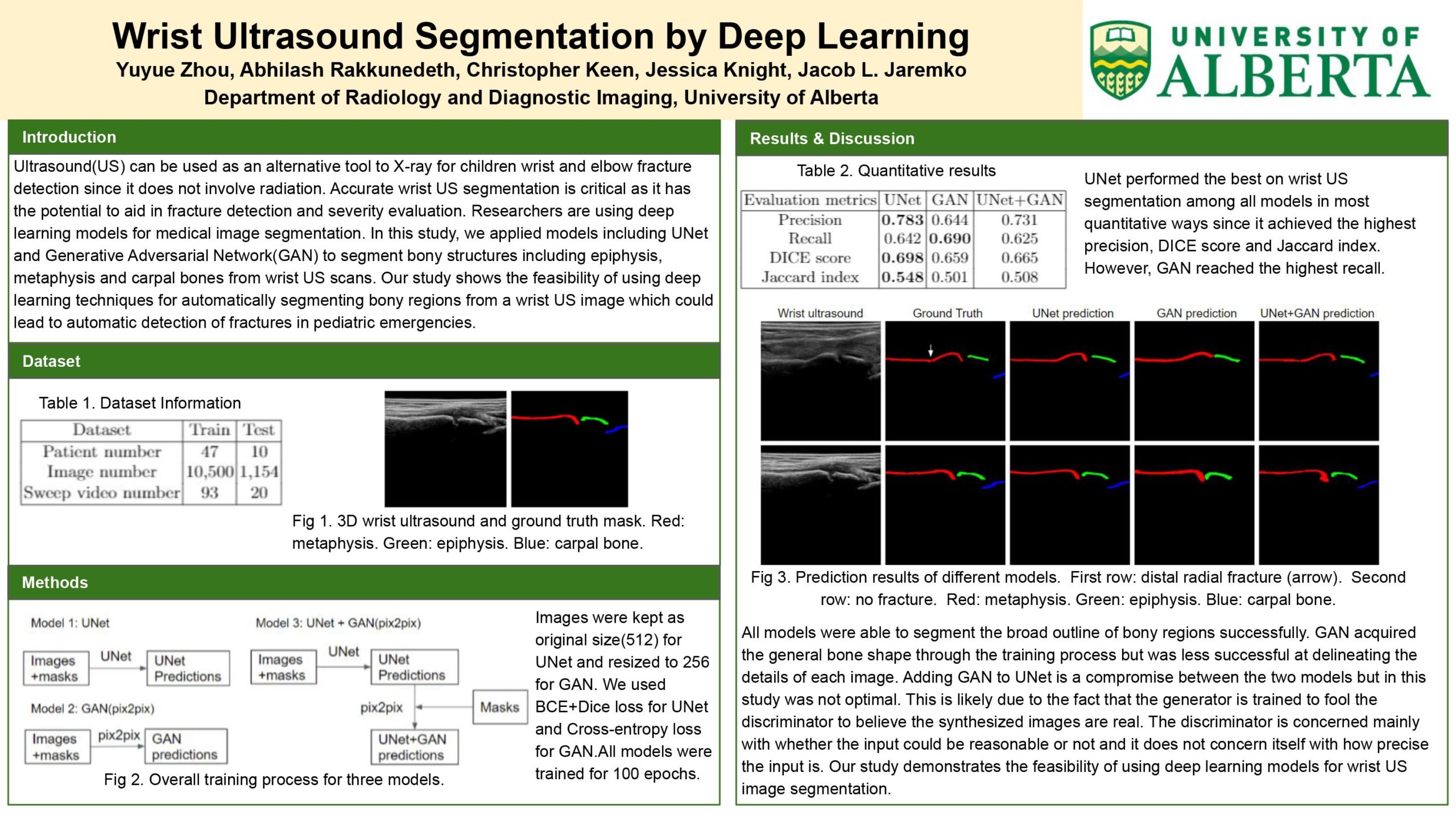
AI and Social Media Identity
Project description:
Supervisor:
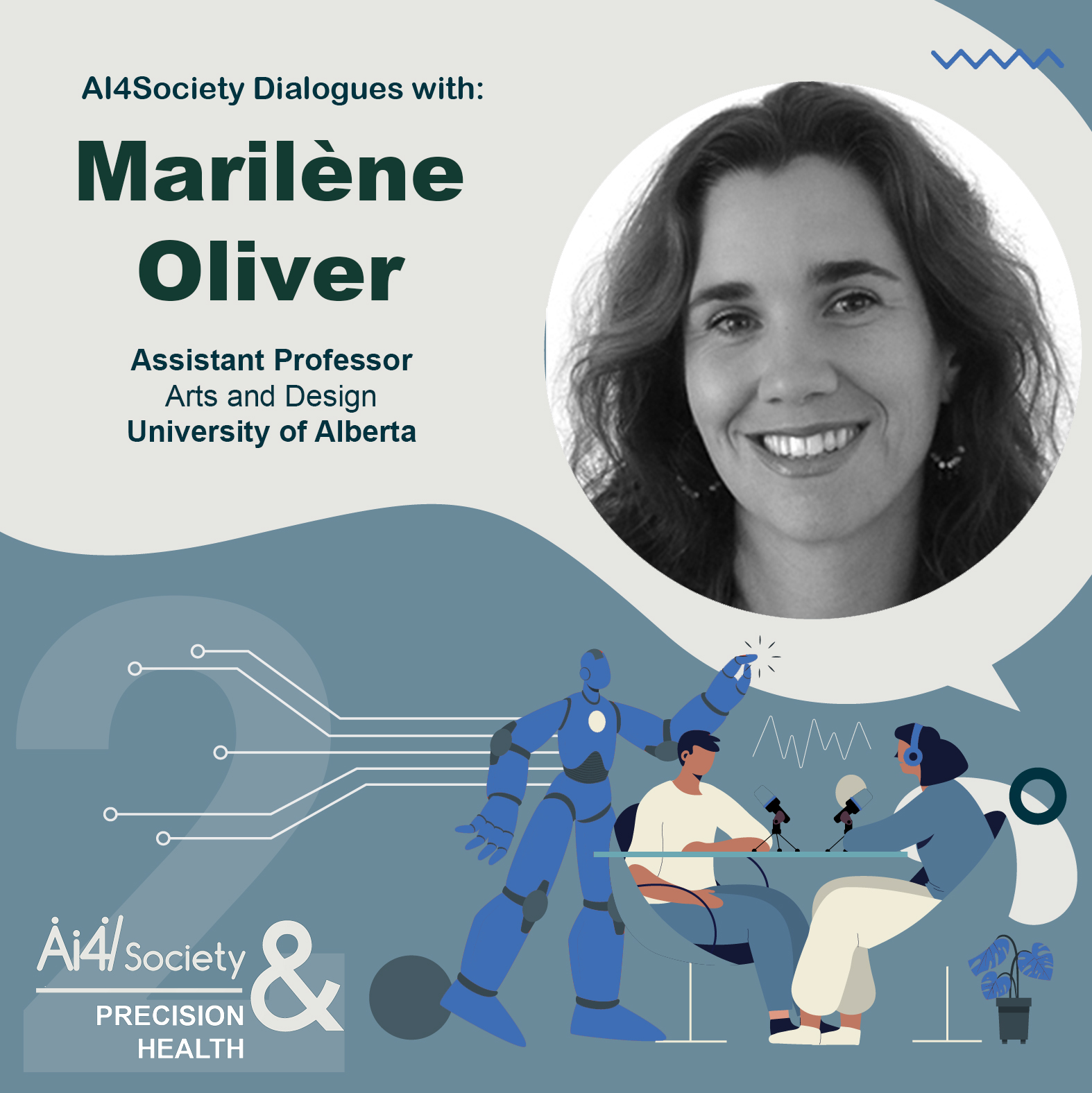
Description:
Supervisor:
Description:
Developing games is challenging as it requires expertise in programming skills and game design. This process is costly and consumes a great deal of time. In this paper we describe a tool we developed to help decrease this burden: Mechanic Maker. Mechanic Maker is designed to allow users to make 2D games without programming. A user interacts with a machine learning AI agent, which learns game mechanics by demonstration.
sdafsdf
Researcher: Vardan Saini
Supervisor: Matthew Guzdial
Developing games is challenging as it requires expertise in programming skills and game design. This process is costly and consumes a great deal of time. In this paper we describe a tool we developed to help decrease this burden: Mechanic Maker. Mechanic Maker is designed to allow users to make 2D games without programming. A user interacts with a machine learning AI agent, which learns game mechanics by demonstration.
Virtual GymVR: Serious Exergame Platform for Personalized Physical and Cognitive Activities
Researcher: Victor Fernandez Cervantes | Supervisor: Eleni Stroulia
Developing games is challenging as it requires expertise in programming skills and game design. This process is costly and consumes a great deal of time. In this paper we describe a tool we developed to help decrease this burden: Mechanic Maker. Mechanic Maker is designed to allow users to make 2D games without programming. A user interacts with a machine learning AI agent, which learns game mechanics by demonstration.
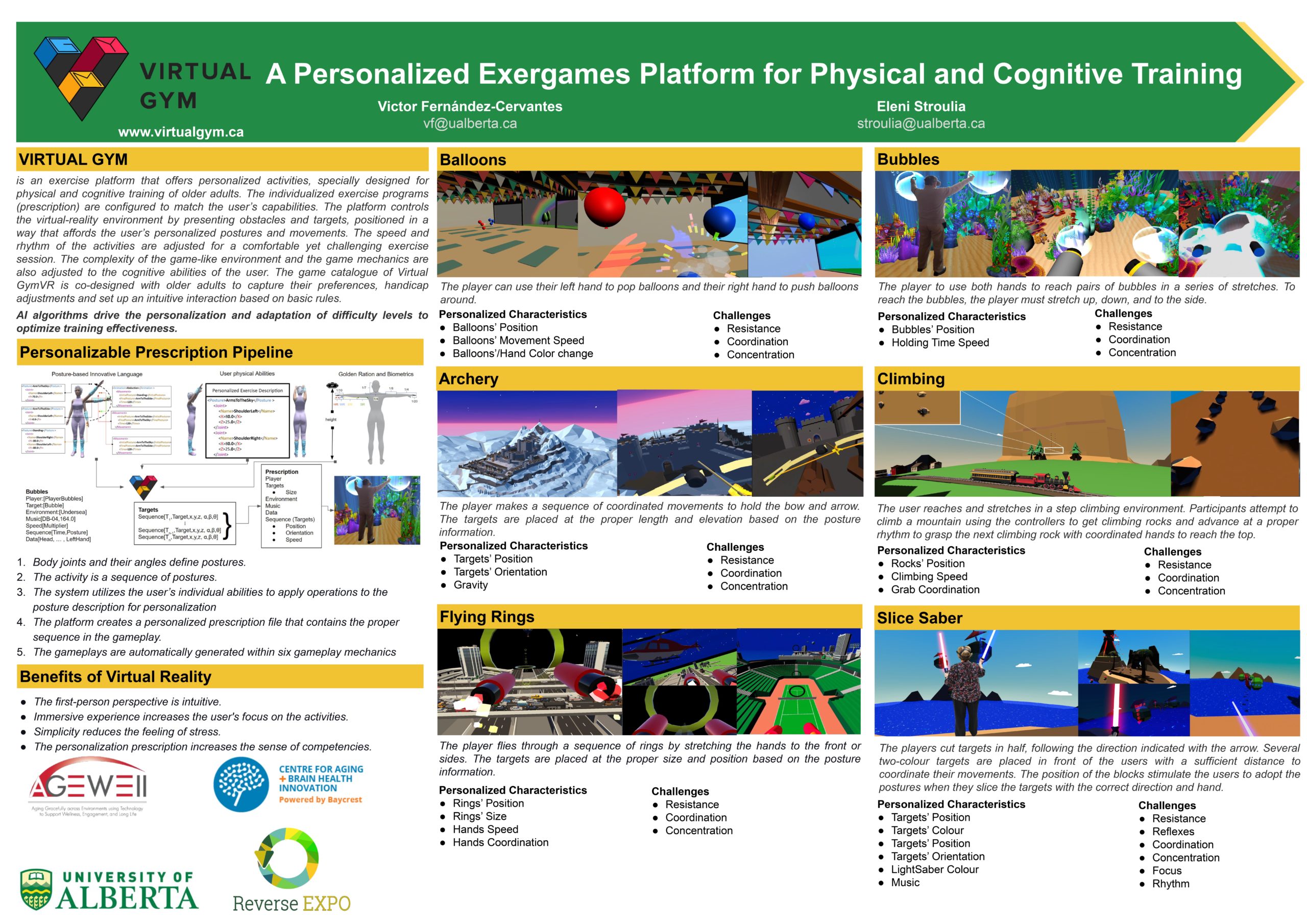
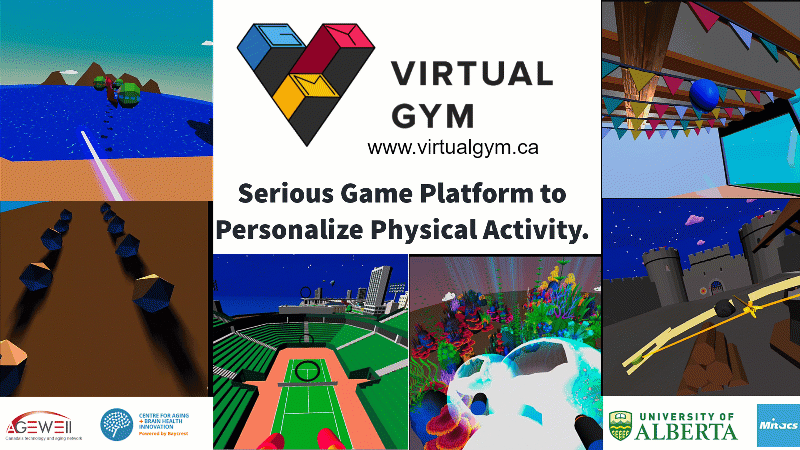
Thank to our sponsors